Differences in mobile phone affinity between demographic groups: implications for mobile phone delivered interventions and programs
Introduction
Because of the ubiquity of mobile phone use among American adults, the mobile phone has become an increasingly popular platform for delivery of behavioral interventions and health-related programs and has shown promise for promoting health behavior change and disease management on a large scale (1-11). Surveys conducted from 2016 to 2018 by the Pew Research Center show that 95% of American adults now own a mobile phone of some kind (12,13). Demographic surveys show high rates of mobile phone ownership across race, ethnicity and gender groups including African Americans (94%), Whites (94%), Hispanics (98%), men (96%), and women (94%) (12,13). In general, similar rates of mobile phone ownership are evident across most age groups (12,13), with text messaging being the communication feature most commonly used among mobile phone owners between ages 18 to 64 (12,13).
While overall ownership is high, there are differences in mobile phone usage that vary by education, income and employment status (14,15). For example, individuals with lower levels of education and income tend to have a higher frequency of mobile phone use and report being more dependent on their phone compared to higher income and more highly educated individuals (14). Greater use of this technology has also been noted more among adults with children (parents or guardians) than those without children (15). These primary demographic (PD) and social demographic (SocD) characteristics may also differentially influence attitudes and cognitions related to mobile phone use.
Over the past 10 years, health-related programs and interventions delivered through mobile phones (mHealth) have been developed to address a wide variety of health behaviors including diabetes management (7-9), smoking cessation (4-6), and physical activity (1-3). While mHealth platforms have shown promise in health behavior interventions, research with different demographic groups are only emerging. So far, while these small pilot studies show evidence of acceptability and preliminary efficacy of mHealth approaches, their overall effect sizes are small (1,10,11). More research is needed to determine the particular mHealth intervention components that are beneficial to enhance behavior across various subpopulations. It has been suggested that the frequency with which an individual carries his or her mobile phone with them, and their patterns of use of various mobile phone features may impact their receptivity to, and engagement with, interventions delivered through the mobile phone, and may ultimately impact the efficacy of mobile phone-delivered programs (16). Further, attitudes toward mHealth and the ways in which mHealth tend to be used may guide choices of potential features when developing a mHealth intervention for a particular target population.
The Mobile Phone Affinity Scale (MPAS) is a recently developed 24-item instrument designed to assess both positive and negative cognitions and behaviors associated with mobile phone use (16). Previous measures of mobile phone and/or internet use tended to focus on problematic patterns of usage (17-22), suggesting that high use of these technologies may be indicative of an addictive or otherwise pathological usage pattern (17-22). This approach appears to disregard potential beneficial aspects of technology use. Positive functions associated with mobile phone use (and technology use, more broadly) may include increasing connections with family and friends (12-16), organizing work related activities, and tracking to improve health behaviors (e.g., tracking physical activity, managing diabetes) (1-6). The psychometric evidence of the MPAS has been previously demonstrated, showing that each subscale has good internal validity and internal consistency.
To our knowledge, no studies thus far have assessed whether subgroups differ in the relationships they have with their mobile phone. This information may help inform the development of mobile phone intervention approaches that would be most relevant for demographic subgroups varying in race, ethnicity, gender and age. Moreover, this study extends the knowledge of SocD characteristics (e.g., education and income) which underscore the nature of the relationship these groups have with this device.
Methods
Procedure and participants
Participants were registered users of the Amazon Mechanical Turk (MTurk). MTurk is reliable and inexpensive recruitment site that facilitates data collection from large, ethnically diverse samples (23-25). Eligible participants were 18 years old or older, resided in the United States, were fluent in English (reading and writing), and owned a mobile phone. To participate in the study, MTurk workers who clicked on our study link were redirected through the MTurk website to our project survey website, which presented detailed information about the study and an informed consent form. After providing electronically signed consent, participants completed the study surveys assessing attitudes and cognitions regarding mobile phone use and demographic information. The online survey was managed through a secure, web-based application, Research Electronic Data Capture (REDCap) (26) hosted by our institution’s Information Services department. Informed consent, human subject protocols and the research were approved by the Institutional Review Board.
Measures
Demographics
Single items corresponding to the US Census were used to assess PD characteristics including participant race, ethnicity, gender and age. Participants also provided SocD information consisting of level of education, income, employment status, marital status, and whether they have children living at home.
MPAS
The MPAS is a 24-item instrument developed to assess both positive and negative behaviors and cognitions related to mobile phone use (16). Three positive subscales assess Connectedness, Productivity, and Empowerment/Safety associated with mobile phone use. These constructs measure individuals’ use of this technology to: (I) to remain connected with friends and family (e.g., “My phone helps me stay close to family and friends”); (II) organize work/school schedule and/or related tasks (e.g., “My phone helps me stay up-to-date with work/school activities”); and (III) the ability to access help when in an unsafe situation (e.g., “Having my phone with me makes it easier to leave a risky situation”). The subscales that assess negative constructs related to mobile phone use are Anxious Attachment (e.g., “I feel anxious if I don’t have my phone with me”), and Addiction (e.g., “I find myself occupied on my phone even when I’m with other people”). A sixth subscale examines Continuous Use (e.g., “I use my phone all day”). Participants respond to each item indicating how true each statement is for them using a 5-point Likert-type scale, ranging from 1 = “Not all true” to 5 = “Extremely True”. Confirmatory factor analysis has previously demonstrated strong measurement structure with high item factor loadings for these respective factors, as well as good internal consistency for each factor (16).
Statistical analyses
Preliminary analyses of the primary and SocD characteristics
Frequency tests were used to assess the distribution of the sample (n=1,055). For race and ethnicity sample size constraints allowed only for comparison between white vs. non-white, and Hispanic vs. non-Hispanic subgroups, respectively. For analyses of age, a median split approach was used to create two age groups, ages 18–29 years and ages 30 and older. Participant responses for level of education included: less than 8th grade, 12th grade, some college, associate degree, bachelor’s degree, and graduate/advanced degree. Employment status was coded into three groups: full-time, part-time, and unemployed or disabled. Marital status was coded into two categories: single or married. Annual income was reported by 747 participants’ and was coded into three categories: (≤$32,000, $33,000–60,000, and ≥$61,000).
Chi-square tests of independence were conducted and compared proportional differences within each of the four PD categories (race, ethnicity, gender and age) for the five SocD factors (education, income, employment, marital status and living with children). Post-hoc tests using adjusted residuals and adjusted P values were conducted following significant chi-square results that included SocD variables with more than two levels (e.g., education) to determine the particular cell that had disproportionately more cases than others. The criterion for statistical significance was set to P=0.01 to reduce the likelihood of type I error (27-29). A conservative threshold value of alpha is recommended particularly for studies with large size wherein even minuscule differences are likely to reach statistical significance at alpha 0.05 (27-29).
Next, a bivariate correlation analysis was used to assess potential collinearity between the six MPAS subscales. Correlation values ranged from to 0.39 to 0.68 (30). A series of multivariate analysis of variance (MANOVA) models evaluated potential interactions between PD variables for each of the six MPAS subscales. These models did not reach statistical significance (P values >0.05). Four separate MANOVAs were conducted for each of the four PD variables and the linear combinations of MPAS subscales while adjusting for any significant differences in SocD factors.
Results
PD
Most participants in this study were white (86.3%, 910/1,055), non-Hispanic (90%, 950/1,055), and nearly half of all participants were men (49.8%, 525/1,055). Half of all participants (50.1%, 526/1,055) were between age 18 and 29, while 49.9% (529/1,055) were age 30 and older. Participant aged 18–87 years old (mean=32.5, SD=10.3). See Table 1 for the distribution of participant SocD characteristics.
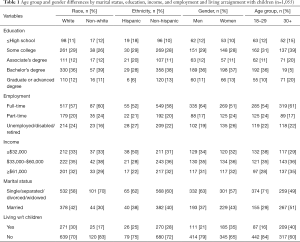
Full table
Differences in SocD variables between PD categories
Race
A significantly higher proportion of whites compared to non-whites were married (χ21=6.53, P=0.01), and whites were more likely to report living with children than non-whites (χ21=9.74, P=0.002). Racial differences were not found for the other SocD variables (all P>0.01).
Ethnicity
A statistically significant association was observed for ethnicity and income (χ24=10.64, P=0.005). Follow-up tests indicated that more non-Hispanics than Hispanics reported an annual income of ≤US $32,000 (χ21=10.56, P=0.0001). Overall, ethnicity was differentially associated with educational attainment (χ24=17.89, P=0.001), however, follow-up tests including a Bonforonni adjusted alpha (P≤0.005) yielded no statistical significance in proportions between Hispanics and non-Hispanics for the five levels of education. No other significant differences were detected for ethnicity and other SocD variables (all P>0.01).
Gender
Women were more likely to report living with children than men, (χ22=24.75, P<0.0001). Differences in employment status were also noted between genders (χ21=18.53, P<0.001). Post hoc tests indicated that more men than women reported full time employment (P<0.0001), while women were likely to report part-time employment (P=0.004). There were no significant gender differences for other SocD variables (P values >0.01).
Age
An examination of the data by participant age group showed that participants ages 18–29 were more likely to be single compared to older participants (χ21=50.61, P<0.0001), and individuals aged 30 or older were more likely to report living with children (χ21=76.86, P<0.0001). Age differences for the other SocD variables did not reach statistical significance (P values >0.01).
Multivariate results
Table 2 presents the means and standard deviations for the PD and SocD variables and each of the MPAS subscales.
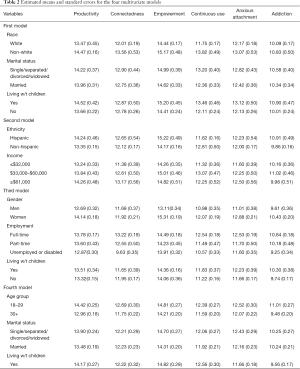
Full table
The first model assessed the role of race, marital status and living with children as they relate to scores on the MPAS. A significant main effect was noted only for the race variable [F6, 1042=3.58; Wilks Lambda (λ) =0.98; P=0.002]. Univariate results showed that non-white participants had significantly higher mean scores on connectedness (F1, 1047=6.70, P=0.01), Productivity (F1, 1047=6.27, P=0.01), and Continuous Use, (F1, 1047=15.99, P<0.0001) compared to white participants.
The second model assessed the relationship between ethnicity, income and the MPAS. A trend toward differences for each the variables was noted for the linear combination of the subscales; however, these associations did not reach statistical significance (P values >0.01).
The third model assessed the relationship between gender, employment, and living with children and the MPAS. A significant effect was found only for gender and the overall MPAS (F 6, 1038=7.11; λ=0.96; P<0.0001). Univariate statistics showed that women had higher mean scores than men on four subscales, connectedness (F1, 1043=15.56, P<0.001), empowerment/safety (F1, 1043=32.02, P<0.001), continuous use (F1, 1043=7.31, P=0.007), and anxious attachment (F1, 1043=19.09, P<0.0001). No significant gender differences were found for other MPAS subscales (P values >0.01).
Employment status was significantly associated with MPAS scores overall (F12, 2038=9.04, λ=0.90; P<0.001). Participants with full time employment scored higher on productivity (F2, 1043=40.36, P=0.001), continuous use (F2, 1023=14.17, P<0.0001), and addiction (F2, 143=6.61, P<001). Living with children did not contribute to the model (P=0.08).
A fourth model assessed the relationship between age, living with children, and marital status and the MPAS. A significant multivariate effect was found for age [F6, 1042=7.33, Wilks Lambda (λ) =0.95, P<0.0001] and living with children (F6, 1042=4.46, λ=0.97, P<0.0001) on the overall MPAS. Univariate analyses showed that participants under age 30 had higher mean scores than their older counterparts on connectedness (F1, 1047=22.70, P<0.0001), productivity (F1, 1047=6.38, P=0.01) and addiction (F1, 1047=20.49, P<0.0001). Individuals living with children at home scored higher on four MPAS subscales: connectedness (F1, 1042=9.65, P=0.002), anxious attachment (F1, 1042=12.07, P<0.0001), addiction (F1, 1042=16.46, P<0.0001), and continuous use (F1,1042=11.01, P=0.001). Marital status was not significantly associated with MPAS constructs (P>0.01).
Discussion
This study examined the role of race, ethnicity, gender and age in shaping the nature of the relationship that individuals have with their mobile phone, in a national sample of American adults. The study findings provide insights into mHealth interventions approaches that may be particularly appealing to different demographic subgroups.
Our data indicate that non-white participants were more likely than whites to rely on their mobile phone to stay connected with friends and family, to organize or complete work/school tasks and tended to use this device continuously throughout the day. These patterns of mobile use suggest that mHealth intervention approaches that incorporate the involvement of a close family member or a friend to provide social support and model positive behaviors may address an important social need for non-whites. In addition, the use of this device by non-whites to organize and/or monitor work and school activities indicates that mobile phone application features that facilitate behavioral monitoring (e.g., goal-setting and self-monitoring) may have stronger appeal among this population consistent with their continuous use of this device. Healthy People 2020 indicates that information technologies that people use on a daily basis provide substantial opportunities to leverage health communication strategies that promote health-related behaviors and outcomes (31,32). For example, a mHealth physical activity intervention that uses activity trackers (e.g., via mobile phone accelerometer) might be expected to have strong appeal to this group.
However, non-whites’ reported continuous use of their mobile phone may also indicate excessive use of this device, which may have important implications for the efficacy of mHealth approaches. It has been noted in previous studies that excessive mobile phone use is associated with poor physical health and overall well-being; it interferes with other aspects of functioning, including prioritizing engagement in health-related behaviors (e.g., physical activity and other self-care behaviors (33,34). A potential solution to overcoming this barrier might be to include sensory technology components to track users’ behaviors as they occur in real time and provide automatic tailored feedback to alter their attention to the target health behavior.
The results from this study also showed that women reported using their phone as a source of connection to family and friends and viewed the phone as a source of empowerment more so than men. Moreover, in comparison to men, women were more likely to report using this device continuously throughout their day and were more likely to be anxious about the availability of their phone and to be nervous when without it. In regards to connectedness, social support is a central facilitator to health behavior promotion that women in particular often report lacking (33,34). Thus, interventions that leverage social influence through the medium of a social networking platform (e.g., peer support chatroom) that women can easily access addresses the socialization aspect that allow women feel connected with others. mHealth intervention content messages that address psychological and social barriers that influence the prioritization of the needs of others over one’s personal health could empower this group to engage in various health behaviors and promote self-care. Similarly, mobile use patterns associated with continuous use and anxious attachment among women might be indicative of their preoccupation with daily and competing priorities that negate self-care. Intervention approaches that motivate women to set aside time to engage in health and mental health behaviors may help to decrease both the unremitting use and addictive relationship with their mobile phone. There is empirical evidence to suggest that physical activity engagement among women is associated with life satisfaction among women (35,36). Thus, mobile short text messages that address engagement in physical activity in the context of improved well-being may appeal to this group.
SocD results showed that employment status was tied to indicators of intense phone use. Specifically, individuals who were employed full time were more likely to use their phones for work productivity, but also to use it continuously throughout the day and showed addictive patterns of phone use. Mobile phone technologies provide users with the ease of accessing their work wherever they are and is related to work productivity in some groups (37,38). However, the availability of this technology may also facilitate obsessive working behaviors and conflicts between work and family life (e.g., spending time with family and friends (39,40). Over the past few years, clinical treatments have been developed to address the rise of mobile phone addiction, also called “nomophobia” (41-43). This pattern of mobile phone use may inform mHealth interventions that teach these individuals skills needed to eventually achieve a balanced work and personal life.
Age differences found in patterns of phone use showed that younger adults (those under age 30) were more likely than older adults to use their phones to connect with family and friends, to organize work/school, and were more likely to endorse addictive behaviors in mobile phone use. The high prevalence of mobile phone use among younger individuals is consistent with previous research (12,44-47). Moreover, persons aged 18–29 have been mobile phone owners during most of their lifetime, and thus are more likely to use this technology to complete many tasks compared to older adults. However, the prevalence of this technology and its long-term use may also predispose this group to developing abnormal/addictive relationships with their mobile phone (44-47). A recent report indicates that younger adults spend a significantly greater amount of time using various mobile applications compared to older adults (48). An increasing number of interventions aimed at decreasing mobile phone or internet addiction in young adults have been developed (49-51). While excessive use of mobile phone technology among this group indicates high level of acceptability to mHealth intervention, the nature of addiction to this device is a potential barrier for individuals to focus on the intervention messages and implementing recommended strategies.
Lastly, individuals who had children living at home noted using their phone more to stay connected to family and friends and tended to use their phones continuously throughout the day more than individuals not living with children. They also showed addictive behaviors towards their phones and endorsed feeling anxious about not having the phone continuously available. It may be that parents or guardians use their phone more frequently to communicate with their children because this form of communication is preferred among children (52-54). Moreover, parents or guardians may feel anxious without their mobile phone because it allows them to reach (and be reached by) their children in cases of emergencies. However, studies have shown mobile phone addiction among parents as an emerging problem, and that parents who were reportedly addicted to their mobile phone were also more likely to rate their children as having significant behavioral problems (55-57). Accordingly, parenting-skills based interventions or programs should assess the potential role of mobile use in parent-child relationships, children’s behavioral issues and identify parenting skills that are necessary to address these issues.
Limitations
The following limitations are noted. This study did not directly assess whether individuals’ relationship with their mobile phone would influence the likelihood of their participation in mobile-delivered interventions or programs. While individuals’ mobile phone affinity may serve as an indicator of the potential acceptability of mHealth interventions, information from the MPAS alone is limited in predicting acceptance of mHealth approaches. The relevance of intervention content to the particular target group has been shown to be a strong factor in the acceptability and engagement in behavioral health interventions (1,2,58-61). This study also had a relatively small proportion of participants who identified as Hispanic, thus this study should be replicated with a larger sample of that population in order to make more firm conclusions about mobile phone affinity among Hispanics. It should also be noted that analyses included only demographic subgroups that were of adequate sample size. Adequate statistical power was not available to analyze results by individual racial groups other than white and our sample of Hispanics was relatively small. Therefore, it is unclear whether similar findings would be noted with other ethnic and racial subgroups (e.g., African American or Asian subgroups). Further research with these populations should consider investigating the aforementioned factors underlying individuals’ acceptability of mHealth interventions and individuals’ relationships with their mHealth across various demographic subgroups.
Nevertheless, these results extend current explanatory knowledge of race, ethnicity, gender and age on empirical psychosocial constructs for mobile phone use among adults. Additionally, the findings demonstrate the role of employment and living with children as contributory factors to the understanding of mobile use pattern among women and men, and among age subgroups. These findings emphasize the importance of a complete understanding of individuals’ relationship with mobile technology in the assessment of acceptability and engagement of mHealth interventions among different adult subgroups.
Acknowledgements
Funding: Research reported in this paper was supported by the National Institute on Alcohol Abuse and Alcoholism of the National Institutes of Health (award number R21AA021014) to Dr. Bock. While completing this paper, Dr. Sillice was supported by the Minority Supplement awarded from the National Heart, Lung and Blood Institute National Institutes of Health (award number 3R01HL127695).
Footnote
Conflicts of Interest: The authors have no conflicts of interest to declare.
Disclaimer: The content is solely the responsibility of the authors and does not necessarily represent the official views of the National Institutes of Health.
References
- Fanning J, Mullen SP, McAuley E. Increasing physical activity with mobile devices: a meta-analysis. J Med Internet Res 2012;14. [Crossref] [PubMed]
- Head KJ, Noar SM, Iannarino NT, Harrington NG. Efficacy of text messaging-based interventions for health promotion: a meta-analysis. Soc Sci Med 2013;97:41-8. [Crossref] [PubMed]
- O'Reilly GA, Spruijt-Metz D. Current mHealth technologies for physical activity assessment and promotion. Am J Prev Med 2013;45:501-7. [Crossref] [PubMed]
- Scott-Sheldon LA, Lantini R, Jennings EG, et al. Text messaging-based interventions for smoking cessation: a systematic review and meta-analysis. JMIR mHealth and uHealth 206;4:e49.
- Rodgers A, Corbett T, Bramley D, et al. Do u smoke after txt? Results of a randomised trial of smoking cessation using mobile phone text messaging. Tob Control 2005;14:255-61. [Crossref] [PubMed]
- Spohr SA, Nandy R, Gandhiraj D, et al. Efficacy of SMS Text Message Interventions for Smoking Cessation: A Meta-Analysis. J Subst Abuse Treat 2015;56:1-10. [Crossref] [PubMed]
- Siopis G, Chey T, Allman-Farinelli M. A systematic review and meta-analysis of interventions for weight management using text messaging. J Hum Nutr Diet 2015;28:1-15. [Crossref] [PubMed]
- Holtz B, Lauckner C. Diabetes management via mobile phones: a systematic review. Telemed J E Health 2012;18:175-84. [Crossref] [PubMed]
- Liang X, Wang Q, Yang X, et al. Effect of mobile phone intervention for diabetes on glycaemic control: a meta-analysis. Diabet Med 2011;28:455-63. [Crossref] [PubMed]
- Coughlin SS, Williams LB, Hatzigeorgiou C. A systematic review of studies of web portals for patients with diabetes mellitus mHealth 2017;3:23. [Crossref] [PubMed]
- Finitsis DJ, Pellowski JA, Johnson BT. Text message intervention designs to promote adherence to antiretroviral therapy (ART): a meta-analysis of randomized controlled trials. PLoS One 2014;9. [Crossref] [PubMed]
- Free C, Phillips G, Watson L, et al. The effectiveness of mobile-health technologies to improve health care service delivery processes: a systematic review and meta-analysis. PLoS Med 2013;10. [Crossref] [PubMed]
- Pew Research Center. Mobile Fact Sheet 2018 (Accessed 2018 March 1). Available online: http://www.pewinternet.org/fact-sheet/mobile/. Archived at:
- Pew Research Center. Mobile Fact Sheet. 2017 (Accessed 2018 May 14). Available online: http://www.pewinternet.org/fact-sheet/mobile/
- Duggan M. Cell Phone Activities 2013. Washington, DC: Pew Research Center Internet & American Life Project; 2013 (Accessed 2018 May 14). Available online: http://pewinternet.org/Reports/2013/Cell-Activities.aspx
- Who is Smartphone Dependent. (Accessed 2018 March 1). Available online: http://www.pewinternet.org/fact-sheet/mobile/. Archived at:
- Bock BC, Lantini R, Thind H, et al. The Mobile Phone Affinity Scale: Enhancement and Refinement. JMIR mHealth and uHealth 2016;4. [Crossref] [PubMed]
- de la Vega R, Miro J. mHealth: a strategic field without a solid scientific soul. a systematic review of pain-related apps. PLoS One 2014;9. [Crossref] [PubMed]
- Fiordelli M, Diviani N, Schulz PJ. Mapping mHealth research: a decade of evolution. J Med Internet Res 2013;15. [Crossref] [PubMed]
- Riley WT, Rivera DE, Atienza AA, et al. Health behavior models in the age of mobile interventions: are our theories up to the task? Transl Behav Med 2011;1:53-71. [Crossref] [PubMed]
- Bianchi A, Phillips JG. Psychological predictors of problem mobile phone use. Cyberpsychol Behav 2005;8:39-51. [Crossref] [PubMed]
- Billieux J. Problematic use of the mobile phone: a literature review and a pathways model. Current Psychiatry Reviews 2012;8:1-9. [Crossref]
- Rathbone AL, Prescott J. The Use of Mobile Apps and SMS Messaging as Physical and Mental Health Interventions: Systematic Review. J Med Internet Res 2017;19. [Crossref] [PubMed]
- Berinsky AJ, Huber GA, Lenz GS. Evaluating Online Labor Markets for Experimental Research: Amazon.com's Mechanical Turk. Political Analysis 2012;20:351-368. [Crossref]
- Buhrmester M, Kwang T, Gosling SD. Amazon's Mechanical Turk: A new source of inexpensive, yet high-quality, data? Perspect Psychol Sci 2011;6:3-5. [Crossref] [PubMed]
- Paolacci G, Chandler J. Inside the Turk: Understanding Mechanical Turk as a Participant Pool. Current Directions in Psychological Science 2014;23:184-8. [Crossref]
- Harris PA, Taylor R, Thielke R, et al. Research Electronic Data Capture (REDCap) - A metadata-driven methodology and workflow process for providing translational research informatics support. J Biomed Inform 2009;42:377-81. [Crossref] [PubMed]
- Kline, RB. Beyond significance testing: Reforming data analysis methods in behavioral research. 2nd Ed. Washington, DC: American Psychological Association; 2013.
- Cohen J. The earth is round (p < .05). American Psychologist 1994;49:997-1003. [Crossref]
- Kline RB. Beyond significance testing: Reforming data analysis methods in behavioral research. 2nd Ed. Washington, DC: American Psychological Association; 2013.
- Harlow LL. The Essence of Multivariate Thinking. Mahwah, New Jersey: Lawrence Erlbraum Associates Publishers, 2005.
- Healthy People 2020 Health Communication and Health Information Technology Overview. (accessed on April 29 2018). Available online: http://www.healthypeople.gov/2020/topics-objectives/topic/health-communication-and-health-information-technology
- Healthy People 2020 Nutrition, Physical Activity, and Obesity Overview & Impact. (accessed on April 29 2018). Available online: http://www.healthypeople.gov/2020/leading-health-indicators/2020-lhi-topics/Nutrition-Physical-Activity-and-Obesity
- Peterson JA, Yates BC, Atwood JR, Hertzog M. Effects of a physical activity intervention for women. West J Nurs Res 2005;27:93-110. [Crossref] [PubMed]
- Peterson JA, Cheng AL. Heart and soul physical activity program for African American women. West J Nurs Res 2011;33:652-670. [Crossref] [PubMed]
- Moreno-Murcia JA, Belando N, Huescar E, et al. Social support, physical exercise and life satisfaction in women. Revista Latinoamericana de Psicología 2017;49:194-202. [Crossref]
- Elavsky S, McAuley E. Physical activity, symptoms, esteem, and life satisfaction during menopause. Maturitas 2005;52:374-385. [Crossref] [PubMed]
- Lazar M. Statistics on mobility in the workplace: Productivity improves. Nov 16, 2017. Available online: https://www.insight.com/en_US/learn/content/2017/01-16-2017-workplace-mobility-statistics-show-improved-productivity.html
- Karaboutis A. The Evolving workforce. Sept 12, 2011. (Accessed on April 2018). Available online: https://blog.devicemagic.com/how-mobile-technology-boosts-productivity-in-the-workplace
- Andreassen CS. Workaholism: An overview and current status of the research. J Behav Addict 2014;3:1-11. [Crossref] [PubMed]
- The inner working of workaholic. Sept. 27, 2017. (Accessed on April 2018). Available online: https://www.insurancequotes.com/business/the-inner-workings-of-a-workaholic
- King AL, Guedes E, Neto JP, et al. Nomophobia: Clinical and Demographic Profile of Social Network Excessive Users. J Addict Res Ther 2017;8:339.
- Bragazzi NL, Del Puente G. A proposal for including nomophobia in the new DSM-V. Psychol Res Behav Manag 2014;7:155-60. [Crossref] [PubMed]
- King AL, Valença AM, Nardi AE. Nomophobia: the mobile phone in panic disorder with agoraphobia: reducing phobias or worsening of dependence. Cogn Behav Neurol 2010;23:52-4. [Crossref] [PubMed]
- Toda M, Monden K, Kubo K, et al. Mobile phone dependence and health-related lifestyle of University studentts. Self Identity 2006;34:1277-84.
- Kuss DJ, Griffiths MD. Online social networking and addiction—a review of the psychological literature. Int J Environ Res Public Health 2011;8:3528-52. [Crossref] [PubMed]
- Chóliz M. Mobile phone addiction: a point of issue. Addiction 2010;105:373-4. [Crossref] [PubMed]
- Leung L. Leisure boredom, sensation seeking, self-esteem, addiction symptoms and patterns of cell phone use. In: Konijn EA, Utz S, Tanis M, et al. editors. Mediated Interpersonal Communication. Mahwah, NJ: Lawrence Erlbaum Associates; 2007:359-81.
- Acharya JP, Acharya I, Waghrey D. A Study on Some of the Common Health Effects of Cell-Phones amongst College Students. J Community Med Health Educ 2013;3:214. [Crossref]
- Young KS. Treatment outcomes using CBT-IA with intervent-addicted patients. J Behav Addict 2013;2:209-15. [Crossref] [PubMed]
- Young KS. Cognitive behavior therapy with internet addicts: Treatmet outcomes and implications. Cyberpsychol Behav 2007;10:671-9. [Crossref] [PubMed]
- Bataller SA. Tratamiento de un caso crónico de adicción a Internet. Cuadernos de Medicina Psicosomática y Psiquiatría de Enlace 2006;79/80:71-88.
- Leena K, Tomi L, Arja RR. Intensity of mobile phone use and health compromising behaviours – how is information and communication technology connected to health-related lifestyle in adolescence. J Adolesc 2005;28:35-47. [Crossref] [PubMed]
- Walsh SP, White KM, Young RM. Needing to connect: the impact of self and others on young people’s involvement with their mobile phone. Aust J Psychol 2010;62:194-203. [Crossref]
- Sánchez-Martínez M, Otero A. Factors associated with cell phone use in adolescents in the community of Madrid (Spain). Cyberpsychol Behav 2009;12:131-7. [Crossref] [PubMed]
- AVG Technologies’ research examines relationship between parents, children and smartphones. Available online: https://now.avg.com/digital-diaries-kids-competing-with-mobile-phones-for-parents-attention/
- Radesky J, Miller AL, Rosenblum KL, et al. Maternal mobile device use during a structured parent–child interaction task. Acad Pediatr 2015;15:238-44. [Crossref] [PubMed]
- Reid DJ, Reid FJ. Text or talk? Social anxiety, loneliness, and divergent preferences for cell phone use. Cyberpsychol Behav 2007;10:424-35. [Crossref] [PubMed]
- Radesky JS, Kistin CJ, Zuckerman B, et al. Patterns of mobile device use by caregivers and children during meals in fast food restaurants. Pediatrics 2014;133:e843-9. [Crossref] [PubMed]
- Sillice MA, Morokoff PJ, Ferszt G, et al. Using Relational Agents to Promote Exercise and Sun Protection: Assessment of Participants’ Experiences With Two Interventions. J Med Internet Res 2018;20. [Crossref] [PubMed]
- Muench F, van Stolk-Cooke K, Morgenstern J, et al. Understanding messaging preferences to inform development of mobile goal-directed behavioral interventions. J Med Internet Res 2014;16. [Crossref] [PubMed]
Cite this article as: Sillice MA, Dunsiger S, Jennings E, Lantini R, Bock BC. Differences in mobile phone affinity between demographic groups: implications for mobile phone delivered interventions and programs. mHealth 2018;4:39.