Engaging youth in mHealth: what works and how can we be sure?
Introduction
Engagement with digital health interventions (DHIs) can be defined using metrics such as the amount (e.g., total number of times accessed the intervention), duration (e.g., total time of use), frequency (e.g., patterns of use) and depth (e.g., content consumed within intervention) of intervention use (1,2). However, while these metrics do capture what the user does within the DHI, it may not fully capture the complex relationship between what the user is doing online and the DHI targets for offline behavior change. Recent work by Yardley et al. attempts to bridge these processes by conceptualizing that there are two levels of engagement that should be considered. Micro-level engagement is the moment-to-moment level of use including a user’s experience while in the DHI, while macro-level engagement refers to the depth of involvement in the behavior change process (including any offline actions the user takes) that the intervention is meant to address (3).
In many mHealth intervention trials, effective engagement, defined as the sufficient amount, duration, frequency or depth of interactivity required to achieve intended behavioral outcomes, does not occur (3,4). This challenge has been reported across DHI intervention studies enrolling youth with chronic health diseases, including HIV (5-9). Youth in particular may become habituated to the mobile application (app, which also includes mobile-optimized websites) or pulled in other directions due to competing demands from social media and entertainment-based apps (10-13). At present, however, it remains challenging to categorize engagement accurately. For example, researchers might perceive users’ disengagement to reflect premature intervention attrition (e.g., a user stopped engaging with the DHI before reaching the researchers’ desired engagement level) when in fact it may be that users required less engagement than expected to achieve the behavioral target. Thus, simply assessing in- app usage may fail to provide the full picture of engagement. A model proposed by Yardley et al., would suggest that disengagement at the micro-level (e.g., stopping app use) if this occurs after a period of effective engagement, does not necessarily mean that the user is not still immersed in the DHI at the macro-level (3). For example, a participant may stop using an adherence focused app to track their daily medication doses (e.g., disengage at the micro-level) but still use skills learned within the app (e.g., tying medication adherence to a daily behavior like eating breakfast) to continue taking daily medications as prescribed (e.g., continue to engage at the macro-level).
Defining and operationalizing effective intervention engagement is not simple. As a starting place, investigators should consider how, in an ideal world, they would want or intend users to engage with the DHI. This means deciding what is deemed to be sufficient engagement at both the micro and macro level to result in the behavior change desired. Given that most DHIs include multiple components, decisions regarding engagement with individual component as well as combined usage must be considered. Further, investigators should plan to account for pathways and patterns of use of features that may impact intervention outcomes and adjust their data analytic plans accordingly.
Given the vulnerability of youth in acquiring HIV and other sexually transmitted infections (14,15), researchers and policy makers have advocated for the inclusion of HIV programs and interventions that are “youth focused (16,17).” DHIs are well-suited for youth given the ubiquity of technology use in this population and its established feasibility and acceptability to address HIV prevention and care outcomes (10,18). However, bringing DHIs to scale will ultimately require a better understanding of both what effective engagement is, how to implement strategies linked to micro and macro level engagement, and how to measure it among youth participating in these trials. Recognizing this need, the Adolescent Trials Network for HIV Interventions (ATN) funded the UNC/Emory Center for Innovative Technology (iTech). iTech aims to lower the burden of HIV infection by developing and evaluating innovative, interdisciplinary research on DHIs across the HIV prevention and care continuum for at-risk youth and youth with HIV aged 15–24 years in the United States (US).
Methods
In this paper we highlight five iTech interventions (see Table 1 and intervention protocol papers for full details) (19-24) to explore how, by designing personalized and developmentally tailored interventions that prioritize the inclusion of strategies to facilitate both micro and macro level engagement, we can maximize the appeal and relevance of young users, thereby increasing the likelihood of positive behavioral change. In this paper, we first discuss six strategies that have shown promise for increasing engagement in DHIs and increasing its likelihood of being perceived as useful, relevant and “youth focused” among adolescents and young adults. These components include use of theory in DHI development, tailoring, provision of self-monitoring and feedback, fostering social support from peers and providers, including “push factors” (reminders, notifications) and inclusion of game-based elements (12,25-30). We then highlight how these components have been operationalized by providing concrete examples from five DHIs being evaluated for efficacy in iTech (19-24).
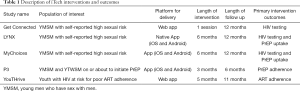
Full table
The University of North Carolina at Chapel Hill, Institutional Review Board has approved all iTech studies. The University of North Carolina at Chapel Hill has committed to uphold regulatory and ethical standards through a Federal Wide Assurance (FWA) approved by the federal Office for Human Research Protections (OHRP). Our assurance with OHRP is FWA #4801.
Results
Strategies that have shown promise for increasing online engagement
Theory-based
Theory can and should play an important role in DHIs by (I) narrowing intervention focus; (II) guiding intervention design and function; (III) informing individual intervention components; (IV) determining what outcomes of interest will be evaluated; and (V) directing analysis and interpretation of results. Theories used can be specific to models of health behavior change, such as the Information, Motivation, Behavioral-Skills Model (IMB) (31) and Social Cognitive Theory (SCT) (32) or they can be specific to digital interventions, such as the Fogg Behavioral Model, persuasive system design, or the eHealth Behavioral Intervention (BIT) model (33,34). Many reviews of eHealth interventions in HIV have assessed the incorporation of theory (28,35-37), with inclusion showing greater positive effect sizes. However, there has been an uneven application of theory within mHealth HIV intervention studies (28). While many DHIs failed to incorporate any behavioral theory, even when used to design intervention messaging and content, the mechanisms of change proposed by the theoretical framework are often not explored in intervention evaluation (28,35,36).
Tailoring
Within mHealth, tailoring refers to the use of individuals’ data to customize intervention content based on variation in their psychological, social, and behavioral profiles with the expectation that this individualization will lead to larger intended effects of these communications (38,39). Tailoring uses information about the individual to determine what: (I) content to deliver, (II) the context/frame for delivery, and the (III) method or channel(s) of delivery. Tailoring may be achieved through personalization and customization of the intervention design (e.g., as part of registration, the app requests the users first name, then all future messages address the user by their first name) or by allowing users to change specific features of the intervention (e.g., choose a background color or screen saver image, create a personal profile, edit an avatar, set medication alarms) that better suit their needs and preferences.
Tailoring has been shown to increase the message or content relevance to an individual end-user, with tailored interventions producing and maintaining higher rates of behavior change than non-tailored programs in a variety of health domains (40). Tailoring also allows investigators to understand behavioral mechanisms that contribute to intervention efficacy (41). A review of internet-based behavioral interventions for chronic illness suggests that user engagement may be sustained by addressing health concerns that are relevant to the user and offering tailored advice and feedback (42).
Provision of self-monitoring/feedback
Self-monitoring with provision of feedback (SM/F) promotes reflection for self-awareness, serves as cues to action (reminders), provides reinforcements from self-tracking, and can impact risk perceptions, motivations, skills, and behavioral activation (43). Systematic reviews indicate that interventions that increase the frequency of SM are likely to promote behavior change, especially when SM is combined with feedback (44,45). SM/F can introduce routines and habits that can become a behavior with time due to repetition, provides users with reflection and accountability and can be motivational especially if one is able to see positive results or a trend of improvement. While utilized most often in the health and fitness space, a systematic review among youth with HIV found SM/F to be one of the most promising strategies for improving antiretroviral (ART) adherence (46).
Fostering support (to peers and providers)
Features that facilitate access to social support have been found to positively influence engagement in a range of DHIs (1). The availability of social support has been shown to promote engagement by making users feel valued and supported throughout the intervention. A 2011 systematic review conducted to explore which strategies have been integrated into interventions to improve engagement, and what the relative effectiveness of these strategies were, found that provision of peer and counselor support was one of the most important factors associated with better intervention exposure (29).
In terms of provision of peer support, technological advances show promise for generating support networks that bypass geographic boundaries and leverage the desire for human connection and social interaction. In a systematic review evaluating the effectiveness of online social networks interventions, most (9 of the 10) trials included had significant improvements in health behavior change mechanisms or outcomes, offering preliminary evidence that social networking-based health interventions may be effective in changing behavior (47). Delivery of interventions that use existing online social networks such as Facebook and Twitter appear to offer particular promise for sustained engagement, due to their high level of user retention and engagement (48).
Inclusion of “push factors” (reminders, notifications)
These consist of prompts (email or text reminders) delivered either within or outside of the app designed specifically to promote continued user engagement with the DHI. In the same systematic review described above, regular contact by email or phone appeared to result in a greater number of log-ins (29). Another systematic review conducted specifically to evaluate the effectiveness of technology-based reminders for promoting engagement with DHIs found small but positive effects of these strategies on engagement compared to no strategy (25). The characteristics of notifications, such as timing, duration, frequency, content or delivery modality that are most impactful has not been fully elucidated.
Inclusion of game-based elements
Gamification is the term used to describe when game elements, such as game mechanics (e.g., competition, collaboration) and dynamics (e.g., points, rewards), are applied to a nongame context (26). Interventions can utilize gamification to deliver highly engaging content, enhancing the degree and depth of participant interaction and increasing behavior change opportunities (27). Including game-based elements can make health activities fun, enjoyable, and understandable thereby increasing one’s intrinsic motivation to engage.
Currently there is no unified framework for evaluating gamification principles within DHIs and data regarding the effectiveness of gamification techniques for increasing engagement is relatively limited. with some indication that effects on engagement are small and not sustained (49,50). A systematic review evaluating the effectiveness of gamification strategies to increase engagement included 15 studies (published through October 2015) involving a total of 10,499 participants. With regard to both direct engagement and downstream behaviors, 12 out of 15 studies found positive significant effects in favor of gamification (51).
Examples of engagement strategies within five iTech interventions
Table 2 provides overview of engagement features used in each of the five interventions within iTech.
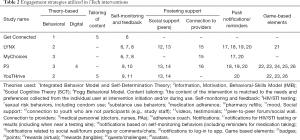
Full table
Use of theory
In thinking of the application of theory, it is most useful to consider how interventions in iTech operationalize theory within DHI components to both engage users and impact their intended behavioral outcomes. The LYNX and MyChoices apps, both designed to increase HIV testing among young men who have sex with men (YMSM), were developed based on different theories (e.g., IMB and SCT, respectively). As such, each employs unique intervention components in line with their theoretical constructs. For example, LYNX, which is based on the IMB model uses sexual history diaries and personalized risk scores to provide YMSM with information and motivation to test regularly; and instructions and guidance to support behavioral skills for HIV/STI testing and PrEP uptake. In MyChoices, in-app reminders and brief assessments about sexual risk and protective health behaviors within the app are used to promote self-efficacy, goal setting, and self-regulation. Self-efficacy is enhanced by facilitative environmental conditions such as the geospatial reminder system in the app. Goal setting and self-regulatory functions are promoted through the participant’s ability to self-monitor their HIV testing through development of testing plans. Some features are common to both interventions. For example, both apps provide home-based HIV/STI-testing options and geospatial-based testing site alerts—within LYNX these features are intended to increase YMSM behavioral skills while in MyChoices, they are targeting an increase in self-efficacy by enhancing the feeling of control over one’s ability to get tested regularly for HIV and use PrEP. While all five interventions were developed based upon well-described theories from health behavior, only one (P3) also incorporates a theory of digital design, the Fogg Behavioral Model, into its intervention framework. According to the Fogg Behavioral Model, the principal factors to promote behavior change using technology include triggers (app notifications), ability (provision of information), and motivation (social support, rewards) (28,52).
Tailoring interventions to users
While all five interventions include some level of tailoring, each does so to varying degrees within two main categories: tailoring of content within the intervention and personalization strategies through in-app messaging. The level and amount of tailoring can differ based on whether the intervention is designed to be a brief or on-going program. Get Connected, for example, is a brief intervention that provides content tailored to each participant based on their psychosocial and sexual profiles and self-reported values collected through a survey administered at intervention initiation. For example, a 19-year-old, Black, single YMSM who has never tested for HIV due to concerns regarding cost who values being strong and successful will receive very different content than a 22-year-old, Latino YMSM in a relationship who has tested previously but does not do so regularly because he doesn’t view testing as urgent and who most values being responsible and real. YouTHrive, on the other hand is an on-going adherence intervention for youth with HIV. While all users receive approximately three Thrive Tips (e.g., brief tips, videos or image-based content about how to live with HIV and better manage medication adherence) a day, users are notified which of the daily tips are personalized to reflect their unique adherence information, motivation, and adherence self-efficacy barriers. As assessed from the baseline survey, these tips are identified with an icon (e.g., a fire symbol) to encourage greater engagement and highlight their relevance to youth’s specific adherence barriers.
Self-monitoring and provision of feedback
This feature is included in all four of the interventions that are meant to last for more than a single session and is tied to youth either tracking a specific behavior (e.g., daily PrEP taking within P3) or responding to in-app surveys or assessments (e.g., completing a sexual partner diary entry in LYNX). Contextualizing what complete or partial engagement with these features are is behavior specific. For example, medication taking is a daily behavior and thus tracking is expected to be daily in order for the app to provide personalized feedback on this activity, while engaging in sex (and thus recording this activity in a sexual diary) may or may not be. It is notable that, while each intervention engages in self-monitoring related to the primary HIV related outcomes of their DHI, many also facilitate self-monitoring of behaviors that are either (I) known to impact HIV-related outcomes or (II) are likely to be important to youth participants, thereby increasing their potential usage of and engagement with the intervention overall.
Fostering social support from peers and providers
Provision of social support from peers and providers can be particularly important when dealing with stigmatized conditions and identities. These connections can be with other youth in the intervention through peer-to-peer forums. In these interventions, forums may be coordinated by youth who work with the study team (i.e., not study participants) to provide support/guidance (e.g., study ambassadors). To further increase engagement, the forums within P3 and YouTHrive share common features/functionality seen within typical social media platforms utilized by youth. For example, entries by participants are displayed in real time and can be liked, reacted to (e.g., thumbs up, heart emoji) or commented on by other participants. However, distinct from social media platforms, no direct messaging between participants is allowed and all posts are visible to everyone. While this may in fact have negative impacts on engagement, a balance must be struck to ensure full human subjects protections are in place. Alternatively, social support can be provided in the absence of direct peer-to-peer interactions through videos and testimonials in which youth share their experiences regarding how they dealt with (and often overcame) similar challenges. Three intervention (P3, YouTHrive and LYNX) utilize this strategy.
While none of the five interventions connect participants directly to their own medical providers or clinics, both LYNX and MyChoices provide access to medically trained study personnel who are able to answer health-related questions, via a bi-directional chat or email, respectively. Within P3, to reinforce PrEP adherence, an adherence coach conducts structured coaching sessions through text messaging within the app.
Inclusion of push factors (reminders, notifications)
Similar to self-monitoring, only the four interventions meant to be used for a sustained time period include push factors to facilitate continuous engagement (or re-engagement) of youth. These notifications may be related to both in-app (those related to forum postings or adherence counselor sessions) or out of app (reminders for HIV/STI testing or medication taking) activities. All four interventions allow employ discreet notifications (typically delivered via text message) specifically designed to re-engage those youth who have not been active on the app for varying periods.
Game-based elements
This feature was used the least among the five interventions and to varying degrees. LYNX awards badges for actions that a user has just completed (e.g., “PrEP’d” badge for starting PrEP or the “Golden Butt” badge for 100% condom use during receptive anal sex in the past month). Both YouTHrive and P3 employ more sophisticated game-based elements including awarding points for completion of in-app activities that lead towards unlocking content, meant to be a “virtual” reward. Only P3 includes “tangible” rewards in the form of financial incentives, based on behavioral economics principles (53,54) and tied to app engagement. Each participant begins the intervention with $90 “seeded” within their “virtual” bank account and can either gain $0.50/day for daily app engagement or lose $1.00/day on days when the app was not used. Thus, at 3-month trial completion, users can earn a range of $0–$135 dollars.
Discussion
Poor engagement is a critical barrier to the effectiveness of mHealth interventions. Deciding what “poor engagement” means and subsequently evaluating its role on study outcomes further complicates the assessment of mHealth effectiveness (2). Reflecting back on both the micro and macro levels of engagement proposed by Yardley will be critical to inform evaluation of effective engagement within iTech DHIs (3). For instance, youth may use the LYNX app routinely to track their sexual encounters (sustained micro-level engagement), but this may not translate into the behavior change of getting HIV tested (lack of macro level engagement). Conversely, another young person may use P3 only for a short period of time to track their PrEP doses (limited micro-level engagement) but continue to successfully adhere even after app discontinuation (sustained macro level engagement).
Another way to characterize engagement within DHIs is by partitioning involvement into three sequential stages: (I) first visit downloading and access; (II) staying on long enough to use and process the information; and (III) revisiting over time (55). This approach may be useful in early studies evaluating initial DHI feasibility and acceptability, it is less useful for evaluating intervention efficacy and limits researchers’ ability to conceptualize how diverse users interact with the DHI (e.g., what are popular features?), to measure feature-specific engagement (e.g., what interactions are linked to behavior change?), and to define thresholds for intervention use (e.g., what is high vs. low engagement?). Given that at least some exposure to content is needed to initiate the process of behavior change, researchers should define a priori what their desired and intended level of engagement is, and, offer a rationale for their decisions. For example, within P3, a decision was made prior to trial initiation to classify usage based on receiving the financial incentive payout each user receives at intervention completion (e.g., >$90= high user). This correlates to using the app for at least two (of the three) months during the intervention period and was chosen to align with literature regarding how long it takes to form a habit (56).
Across the five iTech interventions described, multiple strategies are being used in combination in an attempt to ensure effective youth engagement in DHIs. This is in line with prior studies showing that more than one strategy may be needed for maximal effect; a systemic review showed that the combination of tailored communication, the use of reminders, and the use of incentives resulted in higher exposure to online interventions among adolescents and young adults (12). Nevertheless, significant challenges arise in evaluating the role these strategies have both on engagement itself as well as on the intervention outcomes researchers are attempting to address. By using the “kitchen sink” approach (e.g., applying multiple strategies to all users) it may prove challenging to parse which strategy worked (or did not work) for an individual user. Further, even though empirical evidence suggests that appeal to specific engagement strategies varies between people (57,58), as well as over time within a person (59,60), lack of attention has been paid to systematically tailoring engagement strategies to the changing state and context experienced by youth. For example, while the goal of a reward is to maximize motivation prior to receiving the reward, as well as happiness after receiving the reward, this is only relevant if the reward is something of value to the participant (61). While one user may be motivated to earn points to unlock content in YouTHrive, another may find the content irrelevant to their particular interests or life situation. One solution, as is being done in P3, is to provide a variety of rewards (e.g., unlocking narrative and video content and financial incentives) in the hopes that if one reward is not seen as valuable, another one will be.
Advances in wireless devices and mobile technologies offer novel opportunities for delivering Just-in-Time Adaptive Interventions (JITAI)—an intervention approach that operationalizes the real time selection and personalization of intervention strategies and should be employed in future mHealth studies to systematically investigate ways to promote sustained mHealth engagement among youth within HIV prevention and treatment interventions. Recognizing and responding to distinct user profiles based on their engagement patters through processes such as machine learning has the potential to further tailor interventions to individuals and is a future research area worthy of exploration.
Conclusions
The five iTech studies presented provide insight into potential strategies to enhance youth engagement in HIV prevention and care DHIs, as well as offering ways to conceptualize how best to measure their effectiveness. Ultimately, the evaluation of their success will require careful monitoring of micro and macro level engagement within their ongoing trials, understanding how the distinct strategies contributed to patterns in engagement over time, and their potential inclusion as effect modifiers in each trial’s effectiveness analysis plan. Beyond these five studies, however, we encourage researchers to monitor and document how they incorporate youth-focused engagement within their DHIs in order to create a robust evidence-base for future DHIs and reinforce the importance of including engagement metrics and strategies during their design and testing.
Acknowledgments
Funding: NICHD (U19HD089881).
Footnote
Provenance and Peer Review: This article was commissioned by the Guest Editor (Lisa Hightow-Weidman) for the series “Technology-based Interventions in HIV Prevention and Care Continuum among American Youth” published in mHealth. The article has undergone external peer review.
Data Sharing Statement: Available at http://dx.doi.org/10.21037/mhealth-20-48
Conflicts of Interest: All authors have completed the ICMJE uniform disclosure form (available at http://dx.doi.org/10.21037/mhealth-20-48). The series “Technology-based Interventions in HIV Prevention and Care Continuum among American Youth” was commissioned by the editorial office without any funding or sponsorship. LHW served as the unpaid Guest Editor of the series. All authors report grants from NICHD, during the conduct of the study. The authors have no other conflicts of interest to declare.
Ethical Statement: The authors are accountable for all aspects of the work in ensuring that questions related to the accuracy or integrity of any part of the work are appropriately investigated and resolved. The University of North Carolina at Chapel Hill, Institutional Review Board has approved all iTech studies. The University of North Carolina at Chapel Hill has committed to uphold regulatory and ethical standards through a Federal Wide Assurance (FWA) approved by the federal Office for Human Research Protections (OHRP). Our assurance with OHRP is FWA #4801.
Open Access Statement: This is an Open Access article distributed in accordance with the Creative Commons Attribution-NonCommercial-NoDerivs 4.0 International License (CC BY-NC-ND 4.0), which permits the non-commercial replication and distribution of the article with the strict proviso that no changes or edits are made and the original work is properly cited (including links to both the formal publication through the relevant DOI and the license). See: https://creativecommons.org/licenses/by-nc-nd/4.0/.
References
- Perski O, Blandford A, West R, et al. Conceptualising engagement with digital behaviour change interventions: a systematic review using principles from critical interpretive synthesis. Transl Behav Med 2017;7:254-67. [Crossref] [PubMed]
- Short CE, DeSmet A, Woods C, et al. Measuring Engagement in eHealth and mHealth Behavior Change Interventions: Viewpoint of Methodologies. J Med Internet Res 2018;20:e292 [Crossref] [PubMed]
- Yardley L, Spring BJ, Riper H, et al. Understanding and Promoting Effective Engagement With Digital Behavior Change Interventions. Am J Prev Med 2016;51:833-42. [Crossref] [PubMed]
- Michie S, Yardley L, West R, et al. Developing and Evaluating Digital Interventions to Promote Behavior Change in Health and Health Care: Recommendations Resulting From an International Workshop. J Med Internet Res 2017;19:e232 [Crossref] [PubMed]
- Lopez C, Ramirez DC, Valenzuela JI, et al. Sexual and reproductive health for young adults in Colombia: teleconsultation using mobile devices. JMIR Mhealth Uhealth 2014;2:e38 [Crossref] [PubMed]
- Hightow-Weidman LB, LeGrand S, Simmons R, et al. healthMpowerment: Effects of a Mobile phone-optimized, Internet-based Intervention on Condomless Anal Intercourse Among Young Black Men who have Sex with Men and Transgender Women: Abstract# WEPEC1001. International AIDS Society Conference; July 23-26, 2017.; Paris, France, 2017.
- Goyal S, Nunn CA, Rotondi M, et al. A Mobile App for the Self-Management of Type 1 Diabetes Among Adolescents: A Randomized Controlled Trial. JMIR Mhealth Uhealth 2017;5:e82 [Crossref] [PubMed]
- Partridge SR, Allman-Farinelli M, McGeechan K, et al. Process evaluation of TXT2BFiT: a multi-component mHealth randomised controlled trial to prevent weight gain in young adults. Int J Behav Nutr Phys Act 2016;13:7. [Crossref] [PubMed]
- Pretlow RA, Stock CM, Allison S, et al. Treatment of child/adolescent obesity using the addiction model: a smartphone app pilot study. Child Obes 2015;11:248-59. [Crossref] [PubMed]
- Hightow-Weidman LB, Muessig KE, Bauermeister J, et al. Youth, Technology, and HIV: Recent Advances and Future Directions. Curr HIV/AIDS Rep 2015;12:500-15. [Crossref] [PubMed]
- Wagner B 3rd, Liu E, Shaw SD, et al. ewrapper: Operationalizing engagement strategies in mHealth. Proc ACM Int Conf Ubiquitous Comput 2017;2017:790-8.
- Crutzen R, de Nooijer J, Brouwer W, et al. Strategies to facilitate exposure to internet-delivered health behavior change interventions aimed at adolescents or young adults: a systematic review. Health Educ Behav 2011;38:49-62. [Crossref] [PubMed]
- Paz Castro R, Haug S, Filler A, et al. Engagement Within a Mobile Phone-Based Smoking Cessation Intervention for Adolescents and its Association With Participant Characteristics and Outcomes. J Med Internet Res 2017;19:e356 [Crossref] [PubMed]
- Centers for Disease Control and Prevention. HIV Surveillance Report, 2016. Volume 28. November 2017. Available online: http://www.cdc.gov/hiv/library/reports/hiv-surveillance.html. Accessed April 12, 2020.
- Centers for Disease Control and Prevention. STDs in Racial and Ethnic Minorities - 2016 STD Surveillance. Available online: https://www.cdc.gov/std/stats16/minorities.htm. Accessed June 12, 2018.
- Centers for Disease Control and Prevention. HIV Among Youth. Available at: Available online: https://www.cdc.gov/hiv/group/age/youth/index.html. Last accessed: May 11, 2020.
- Légaré F, Politi MC, Drolet R, et al. Training health professionals in shared decision-making: an international environmental scan. Patient Educ Couns 2012;88:159-69. [Crossref] [PubMed]
- Mulawa MI, LeGrand S, Hightow-Weidman LB. eHealth to Enhance Treatment Adherence Among Youth Living with HIV. Curr HIV/AIDS Rep 2018;15:336-49. [Crossref] [PubMed]
- Biello KB, Marrow E, Mimiaga MJ, et al. A Mobile-Based App (MyChoices) to Increase Uptake of HIV Testing and Pre-Exposure Prophylaxis by Young Men Who Have Sex With Men: Protocol for a Pilot Randomized Controlled Trial. JMIR Res Protoc 2019;8:e10694 [Crossref] [PubMed]
- Bauermeister JA, Golinkoff JM, Horvath KJ, et al. A Multilevel Tailored Web App-Based Intervention for Linking Young Men Who Have Sex With Men to Quality Care (Get Connected): Protocol for a Randomized Controlled Trial. JMIR Res Protoc 2018;7:e10444 [Crossref] [PubMed]
- Hightow-Weidman LB, Muessig K, Rosenberg E, et al. University of North Carolina/Emory Center for Innovative Technology (iTech) for Addressing the HIV Epidemic Among Adolescents and Young Adults in the United States: Protocol and Rationale for Center Development. JMIR Res Protoc 2018;7:e10365 [Crossref] [PubMed]
- LeGrand S, Knudtson K, Benkeser D, et al. Testing the Efficacy of a Social Networking Gamification App to Improve Pre-Exposure Prophylaxis Adherence (P3: Prepared, Protected, emPowered): Protocol for a Randomized Controlled Trial. JMIR Res Protoc 2018;7:e10448 [Crossref] [PubMed]
- Liu A, Coleman K, Bojan K, et al. Developing a Mobile App (LYNX) to Support Linkage to HIV/Sexually Transmitted Infection Testing and Pre-Exposure Prophylaxis for Young Men Who Have Sex With Men: Protocol for a Randomized Controlled Trial. JMIR Res Protoc 2019;8:e10659 [Crossref] [PubMed]
- Horvath KJ, MacLehose RF, Martinka A, et al. Connecting Youth and Young Adults to Optimize Antiretroviral Therapy Adherence (YouTHrive): Protocol for a Randomized Controlled Trial. JMIR Res Protoc 2019;8:e11502 [Crossref] [PubMed]
- Alkhaldi G, Hamilton FL, Lau R, et al. The Effectiveness of Prompts to Promote Engagement With Digital Interventions: A Systematic Review. J Med Internet Res 2016;18:e6 [Crossref] [PubMed]
- Cugelman B. Gamification: what it is and why it matters to digital health behavior change developers. JMIR Serious Games 2013;1:e3 [Crossref] [PubMed]
- Hightow-Weidman LB, Muessig KE, Bauermeister JA, et al. The future of digital games for HIV prevention and care. Curr Opin HIV AIDS 2017;12:501-7. [Crossref] [PubMed]
- Simoni JM, Ronen K, Aunon FM. Health Behavior Theory to Enhance eHealth Intervention Research in HIV: Rationale and Review. Curr HIV/AIDS Rep 2018;15:423-30. [Crossref] [PubMed]
- Brouwer W, Kroeze W, Crutzen R, et al. Which intervention characteristics are related to more exposure to internet-delivered healthy lifestyle promotion interventions? A systematic review. J Med Internet Res 2011;13:e2 [Crossref] [PubMed]
- Jeminiwa RN, Hohmann NS, Fox BI. Developing a Theoretical Framework for Evaluating the Quality of mHealth Apps for Adolescent Users: A Systematic Review. J Pediatr Pharmacol Ther 2019;24:254-69. [Crossref] [PubMed]
- Fisher JD, Fisher WA, Williams SS, et al. Empirical tests of an information-motivation-behavioral skills model of AIDS-preventive behavior with gay men and heterosexual university students. Health Psychol 1994;13:238-50. [Crossref] [PubMed]
- Bandura A. Social cognitive theory: an agentic perspective. Annu Rev Psychol 2001;52:1-26. [Crossref] [PubMed]
- Mohr DC, Cheung K, Schueller SM, et al. Continuous evaluation of evolving behavioral intervention technologies. Am J Prev Med 2013;45:517-23. [Crossref] [PubMed]
- Mohr DC, Schueller SM, Montague E, et al. The behavioral intervention technology model: an integrated conceptual and technological framework for eHealth and mHealth interventions. J Med Internet Res 2014;16:e146 [Crossref] [PubMed]
- Henny KD, Wilkes AL, McDonald CM, et al. A Rapid Review of eHealth Interventions Addressing the Continuum of HIV Care (2007-2017). AIDS Behav 2018;22:43-63. [Crossref] [PubMed]
- Navarra AD, Gwadz MV, Whittemore R, et al. Health Technology-Enabled Interventions for Adherence Support and Retention in Care Among US HIV-Infected Adolescents and Young Adults: An Integrative Review. AIDS Behav 2017;21:3154-71. [Crossref] [PubMed]
- Riley WT, Rivera DE, Atienza AA, et al. Health behavior models in the age of mobile interventions: are our theories up to the task? Transl Behav Med 2011;1:53-71. [Crossref] [PubMed]
- Hawkins RP, Kreuter M, Resnicow K, et al. Understanding tailoring in communicating about health. Health Educ Res 2008;23:454-66. [Crossref] [PubMed]
- Kreuter MW, Skinner CS. Tailoring: what's in a name? Health Educ Res 2000;15:1-4. [Crossref] [PubMed]
- Morrison LG, Yardley L, Powell J, et al. What design features are used in effective e-health interventions? A review using techniques from Critical Interpretive Synthesis. Telemed J E Health 2012;18:137-44. [Crossref] [PubMed]
- Horvath KJ, Bauermeister JA. eHealth Literacy and Intervention Tailoring Impacts the Acceptability of a HIV/STI Testing Intervention and Sexual Decision Making Among Young Gay and Bisexual Men. AIDS Educ Prev 2017;29:14-23. [Crossref] [PubMed]
- Noar SM, Benac CN, Harris MS. Does tailoring matter? Meta-analytic review of tailored print health behavior change interventions. Psychol Bull 2007;133:673-93. [Crossref] [PubMed]
- Swendeman D, Ramanathan N, Baetscher L, et al. Smartphone Self-Monitoring to Support Self-Management Among People Living With HIV: Perceived Benefits and Theory of Change From a Mixed-Methods Randomized Pilot Study. J Acquir Immune Defic Syndr 2015;69:S80-91. [Crossref] [PubMed]
- Michie S, Abraham C, Whittington C, et al. Effective techniques in healthy eating and physical activity interventions: a meta-regression. Health Psychol 2009;28:690. [Crossref] [PubMed]
- Harkin B, Webb TL, Chang BP, et al. Does monitoring goal progress promote goal attainment? A meta-analysis of the experimental evidence. Psychol Bull 2016;142:198-229. [Crossref] [PubMed]
- Reisner SL, Mimiaga MJ, Skeer MM, et al. A review of HIV antiretroviral adherence and intervention studies among HIV-infected youth. Top HIV Med 2009;17:14-25. [PubMed]
- Maher CA, Lewis LK, Ferrar K, et al. Are health behavior change interventions that use online social networks effective? A systematic review. J Med Internet Res 2014;16:e40 [Crossref] [PubMed]
- Young SD, Cumberland WG, Lee SJ, et al. Social networking technologies as an emerging tool for HIV prevention: a cluster randomized trial. Ann Intern Med 2013;159:318-24. [Crossref] [PubMed]
- Sardi L, Idri A, Fernandez-Aleman JL. A systematic review of gamification in e-Health. J Biomed Inform 2017;71:31-48. [Crossref] [PubMed]
- Kelders SM, Sommers-Spijkerman M, Goldberg J. Investigating the Direct Impact of a Gamified Versus Nongamified Well-Being Intervention: An Exploratory Experiment. J Med Internet Res 2018;20:e247 [Crossref] [PubMed]
- Looyestyn J, Kernot J, Boshoff K, et al. Does gamification increase engagement with online programs? A systematic review. PLoS One 2017;12:e0173403 [Crossref] [PubMed]
- Agha S, Tollefson D, Paul S, et al. Use of the Fogg Behavior Model to Assess the Impact of a Social Marketing Campaign on Condom Use in Pakistan. J Health Commun 2019;24:284-92. [Crossref] [PubMed]
- Haff N, Patel MS, Lim R, et al. The role of behavioral economic incentive design and demographic characteristics in financial incentive-based approaches to changing health behaviors: a meta-analysis. Am J Health Promot 2015;29:314-23. [Crossref] [PubMed]
- Kimmel SE, Troxel AB. Novel incentive-based approaches to adherence. Clin Trials 2012;9:689-95. [Crossref] [PubMed]
- Crutzen R, de Nooijer J, Brouwer W, et al. A conceptual framework for understanding and improving adolescents' exposure to Internet-delivered interventions. Health Promot Int 2009;24:277-84. [Crossref] [PubMed]
- Lally P, Van Jaarsveld CHM, Potts HWW, et al. How are habits formed: Modeling habit formation in the real world. Eur J Soc Psychol 2010;40:998-1009. [Crossref]
- Haugtvedt CP, Petty RE, Cacioppo JT. Need for cognition and advertising: Understanding the role of personality variables in consumer behavior. J Consum Psychol 1992;1:239-60. [Crossref]
- Cialdini RB, Trost MR, Newsom JT. Preference for consistency: The development of a valid measure and the discovery of surprising behavioral implications. J Personal Soc Psychol 1995;69:318. [Crossref]
- Cialdini RB, Goldstein NJ. Social influence: Compliance and conformity. Annu Rev Psychol 2004;55:591-621. [Crossref] [PubMed]
- Sinclair RC, Mark MM, Clore GL. Mood-related persuasion depends on (mis) attributions. Soc Cogn 1994;12:309-26. [Crossref]
- Lewis ZH, Swartz MC, Lyons EJ. What's the Point?: A Review of Reward Systems Implemented in Gamification Interventions. Games Health J 2016;5:93-9. [Crossref] [PubMed]
Cite this article as: Hightow-Weidman LB, Horvath KJ, Scott H, Hill-Rorie J, Bauermeister JA. Engaging youth in mHealth: what works and how can we be sure? mHealth 2021;7:23.