Engagement in mHealth behavioral interventions for HIV prevention and care: making sense of the metrics
Introduction
Engagement in mHealth interventions is the primary metric by which researchers can assess whether participants in a mHealth intervention use and engage with the intervention’s content as intended over a pre-specified period to result in behavior change (1). Engagement in mHealth interventions has been defined as “the extent (e.g., amount, frequency, duration, depth) of usage” and can serve as a proxy for participants’ subjective experience with a mHealth intervention (e.g., attention, interest) (2) alongside (or in lieu of) satisfaction scales (3), usability surveys (4), and qualitative interviews. Data on where and how users access and move through an intervention may be automatically collected as users log on to the technology and use its components. These metrics, referred by Couper et al. as paradata, capture details about the process of interacting with the online intervention (1). Paradata (i.e., intervention usage metrics) have been associated with differential treatment outcomes in mHealth interventions (5-8), yet they remain underexamined and underreported in technology-based HIV interventions (9,10). Given paradata’s utility in characterizing engagement and enhancing the rigor of mHealth trial evaluations, metrics of engagement must be systematically collected, analyzed, and interpreted to meaningfully understand a mHealth intervention’s efficacy.
There has been an increase in mobile health (mHealth) interventions addressing the HIV prevention and care continuum in recent years, given this modality’s potential to reach individuals who may not participate in in-person HIV interventions due to logistical (e.g., transportation, timing) and other structural barriers (e.g., stigma) (11-14). In contrast to many face-to-face interventions in which participants are guided through intervention components by a facilitator, mHealth interventions are designed to be accessed by participants on their own time and in the location of their choosing, creating unique challenges regarding participants’ equitable intervention engagement and rendering evaluation more challenging. To date, there has not been a concerted effort to standardize these metrics across mHealth HIV interventions or characterize how engagement within these interventions is linked to HIV prevention and care outcomes. In a review of published research (Jan 2016–Mar 2017) on the development and testing of online behavioral interventions for HIV prevention and care, only one published trial reported paradata metrics describing participants’ engagement with the intervention (9,10). This review highlights the importance of systematic collection and analysis of paradata to not only strengthen the evidence base for technology-based interventions (i.e., do they work?), but also to inform reach (i.e., for whom do they work?) and scale-up (i.e., under what conditions?) in HIV prevention interventions for young men who have sex with men.
“Effective” engagement required for behavior change support is likely to differ across users and contexts and can only be determined by analyzing complex patterns of relationships between usage, user experiences, and outcomes. Defining a priori what level of engagement is needed for maximal intervention effectiveness is a critical first step that should be done in concert with technology development. What constitutes engagement likely differs based on both the intervention itself (e.g., type of intervention components, technology-platform) and the targeted behavioral outcome (e.g., reduced sexual risk behavior, uptake of HIV/sexually-transmitted infection (STI) testing, pre-exposure prophylaxis (PrEP) or antiretroviral therapy (ART) adherence, stigma reduction). Similarly, the theoretical framework underlying the intervention should serve as a guide to determining what constitutes engagement (15). Given that few studies have presented engagement metrics in this manner to date, this paper provides a review of common paradata metrics in mHealth interventions that can measure the amount, frequency, duration, and depth of use using case studies from four HIV mHealth interventions.
Methods
We propose that the extent of engagement in mHealth interventions is best captured through four domains of use: amount, frequency, duration, and depth. It is critical that deciding what metrics are most important for assessing engagement occur during the process of intervention development, thus ensuring that the backend systems are capturing the correct information in the format needed for analysis prior to implementation. Working with developers to describe how these data will be used can ensure that they are building systems that allow these needs to be met. It can be worthwhile to conduct beta testing of the intervention prior to deployment, not just for assessing the technology itself, but also for ensuring the paradata metrics are robust and the format is correct.
Towards the development of harmonization of technology interventions, we provide a definition for engagement metrics across the four domains assessing extent of use. While some overlap in metrics occurs, we try to include common metrics within each domain. Amount captures a quantity of something, especially the total of a thing or things in number, size, or value. Frequency is the rate at which something occurs or is repeated over a particular period of time or in a given sample. Duration is the time during which something continues. Depth refers to the more detailed study of how different interventions components were used. Most engagement information is assessed through time stamps of each “touch” a user makes with the technology. For example, by capturing each time a participant logs in to the intervention, you can calculate the total duration of use (duration), the total number of times of use (amount), and any patterns of use (frequency). Timestamps of all actions performed in the intervention can be manipulated to determine duration spent using the intervention or within specific components (depth). Directed or closed interventions, where participants typically proceed through modules or components in a pre-specified way, pose fewer analytic challenges as compared to undirected or open interventions, where participants can access all of the intervention components, in any order and for whatever time they choose.
We illustrate how paradata can be collected and analyzed to examine engagement through four case studies led by the authors, with the intent of highlighting how engagement can inform an array of different types of interventions. Each case study was designed to address distinct HIV prevention and care outcomes for young men who have sex with men using diverse theoretical frameworks and intervention strategies, and each had a different expected duration to achieve behavioral change. Table 1 provides a brief description, including information on the outcome of the trials conducted to evaluate each intervention. Standard paradata collected are provided in Table 2 based on evaluating the amount, frequency, duration, and depth of use.
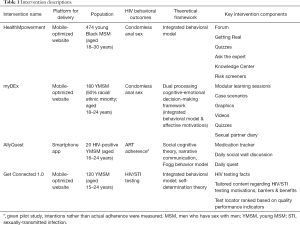
Full table
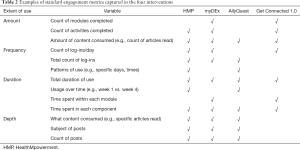
Full table
Results
For each case study below, we briefly summarize the purpose and main outcomes of the intervention and describe different ways by which paradata might be used to supplement intervention design and evaluation. In AllyQuest, we describe the use of paradata to create user profiles and determine a minimum engagement threshold for a future randomized trial based on the initial pilot trial data. In myDEx, we describe how paradata can inform how intervention exposure and dosage might influence the strength of the observed intervention effects and suggest modifications to the intervention design. In Get Connected 1.0, we illustrate how paradata can be used to note how participants’ psychosocial characteristics might influence what theoretically-driven content participants choose to engage with as part of a brief intervention. Finally, in HealthMpowerment, we note the importance of engagement dosage on intervention effects and the utility of employing mixed-methods approaches to paradata in order to examine how interactive engagement between participants influences their perceptions of HIV-related stigma.
A daily interaction intervention: AllyQuest
AllyQuest was a novel, theoretically-based smartphone app intervention designed to improve ART adherence among HIV-positive young men who have sex with men, aged 16–24 years. Core activities were meant to be completed daily throughout the duration of the trial and included a medication tracker, a social wall discussion question, and a daily quest (actionable routine tasks aimed to help users set goals and build knowledge or skills). Each day, participants received a “trigger” to log in to the app and track their ART adherence. This activity resulted in participants receiving a “reward” which included both points and a monetary incentive displayed within their app’s virtual bank. In a 1-month pilot study enrolling 20 young men who have sex with men, the metric used to measure duration was mean total time of app usage (M =158.4 min, SD =114.1). To measure frequency, we assessed the number of login days and number of days where participants logged their medication use. There was a mean of 21.2 days (SD =16.7) of use with a mean of 19.4 days (SD =13.5) of logging medication (16).
But what do these data mean and how do we determine the key engagement metric to use for a future efficacy trial? Based on the theoretical framework, we hypothesized that any daily usage of the app, as assessed by days logging on, would be the best proxy for exposure to at least one of the three core intervention components. In fact, we found a positive and statistically significant association between the number of days logged into the app and participants, knowledge about HIV (rho =0.53, Cohen’s d =1.25) and confidence in their ability to reliably take their HIV medications (rho =0.49, Cohen’s d =1.12) (16).
While not included in the primary analysis, we also assessed amount and depth of exposure. For AllyQuest, the maximum number of activities was 107 and included 30 days of tracking medications, posting on the social wall, completing a quest, and reading all 17 Knowledge Center articles. We examined the total content each participant was exposed to as a percentage of how much content was available. While the overall mean number of activities completed was 49 (45.8%), four participants (25% of sample) completed at least 75% of the total activities and another six participants (50% of sample) completed at least 60%. Differentiating patterns of use among users and how that translates into behavioral outcomes can lead to a more nuanced understanding of which intervention components should be changed or adapted in future iterations.
In anticipation of the next trial of AllyQuest, design changes were made to more accurately assess users’ comprehension with the material provided within the articles. In the pilot trial, an article was considered read if a user opened an article and scrolled to the end of the page. New features which include a check-in and reflection question were added to the end of each article. The check-in question is the same for all articles and asks, “Did you find this article useful?” Users can select from the following three options: “I get it now”, “I already knew this”, or “I’m confused”. The reflection question differs based on the content of the article and provides a space for users to reflect on how the content in the article impacted them directly. An example question based on an article about living with HIV is, “What’s the best piece of advice you have received since your diagnosis? What advice would you give someone else who was just diagnosed?” Analyzing these paradata will provide a more reliable indication of engagement with the Knowledge Center articles in terms of both amount (how many articles actually read) and depth (level of understanding).
A modular intervention: myDEx
myDEx is a mobile-optimized website designed to offer tailored HIV risk reduction content and skills to 180 high-risk, HIV-negative young men who have sex with men who reported meeting sexual partners online. As a pilot randomized controlled trial (RCT), participants were randomized either to myDEx or to an information-only control condition based on the Centers for Disease Control and Prevention’s HIV Risk Reduction Tool Beta Version. myDEx sessions were designed to be modular and delivered through interactive, tailored story-telling, motivational interviewing messaging, and graphics and videos (17). Within each session, participants had access to brief activities designed to build their HIV risk reduction skills and promote self-reflection about their sexual health and partner-seeking behaviors. Cognizant of the challenges in maintaining users’ attention in a mobile-optimized website, we designed each session to keep users engaged for no more than 10 minutes per session.
Overall evaluation of the pilot RCT comparing myDEx to the control arm noted greater reductions in condomless anal intercourse in the myDEx arm at the 3-month follow-up (26.7% in myDEx vs. 45.7% in control arm, Cohen’s d =−0.47), and moderate effect sizes across the proposed theoretical mechanisms of change (range of Cohen’s d: 0.20–0.50) informing the intervention (18). In reviewing the paradata for amount of sessions viewed, however, between-arm analyses of the myDEx paradata suggested differential exposure between the arms. While young men who have sex with men in the control condition were more likely to have viewed all modular sessions compared to those in the myDEx arm, participants using myDEx spent significantly more time interfacing with the content of each module than counterparts in the control condition (see Table 3).
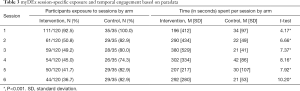
Full table
In the 3-month pilot study, we estimated duration as the mean time participants used the intervention. Duration for overall intervention use varied greatly between and within arms given participants’ ability to navigate content autonomously (i.e., users chose what content and activities to interact with across the sessions). myDEx participants’ duration of intervention use was quite varied across both conditions. Participants assigned to myDEx spent more time on the site than participants assigned to the control condition (Cohen’s d =0.71). myDEx participants had a duration ranging from 0 to 98 min (M =4.95 min; SD =11.0), whereas participants assigned to the control condition spent between 0 and 26 min (M =1.15 min; SD =3.5) on the site. Given the large variability in duration, caution should be taken in the interpretation of mean time spent on site as a marker of intervention efficacy—particularly given users’ ability to autonomously navigate content and select for themselves what was most interesting and relevant for them.
In a secondary analysis of paradata from myDEx participants, duration, amount, and depth seemed to influence intervention dosage and treatment strength. In within-group paradata analyses of the myDEx arm (n=120), participants who reported no condomless receptive anal intercourse during the 3-month follow-up (n=72) were more likely than peers who engaged in condomless receptive anal intercourse (n=48) to have spent more time using the intervention [M =29.34 (SD =29.69) min vs. M =15.51 (SD =26.46) min; Cohen’s d =0.49], navigating a greater number of topics in the intervention [M =45.61 (SD =30.56) vs. M =31.54 (SD =29.53); Cohen’s d =0.47], engaging with more in-depth tailored context [M =17.56 (SD =18.86) vs. M =7.98 (SD =12.73); Cohen’s d =0.62], and interacting with a greater number of activities [M =5.97 (SD =8.39) vs. M =2.83 (SD =6.10); Cohen’s d =0.44].
Even though the pilot trial results from myDEx are encouraging, the use of paradata in between-arm and within-arm secondary analyses suggest that myDEx could have even stronger intervention effects than initially reported if the intervention could promote higher overall engagement as measured by duration, amount and depth.
A brief intervention: Get Connected 1.0
Get Connected 1.0 was designed as a pilot web-based brief intervention that employed individual- and system-level tailoring technology to reduce barriers to HIV prevention among young men who have sex with men. Get Connected 1.0 content followed motivational interviewing principles by offering four webpages focused on resolving ambivalence about HIV prevention behaviors, increasing self-efficacy for change, and enhancing motivation moving toward action. As a brief intervention, the site was designed so that exposure to site content (duration) would not take more than 5 minutes to read and navigate. The average time spent on the intervention was 322.67 seconds (SD =385.40). Participants in the pilot RCT (n=130; aged 15–24 years) were randomized to receive the Get Connected 1.0 intervention or an attention-control condition (a test locator that ranked sites based on quality performance metrics). Results from the pilot trial at the 30-day post-intervention follow-up indicated clinically meaningful effect sizes in HIV or STI testing behavior (Cohen’s d =0.34), as well as self-efficacy to discuss HIV testing with partners (Cohen’s d range from 0.33 to 0.50) and trust in providers (Cohen’s d =0.33) (19).
Paradata were collected as participants navigated the Get Connected 1.0 site. The site’s database timestamped every action performed by each participant. As a measure of amount, we measured intervention use by counting the number of theoretically informed content features that participants clicked while navigating each section of the intervention. There were 40 clickable features embedded in the intervention. On average, participants clicked a total of 10.28 features (SD =6.94) across all four sections of the intervention. Similarly, we measured engagement by extracting the time participants spent in each of the four sections of the site and the overall engagement with the intervention (total time spent on intervention).
In a secondary analysis of the paradata (20), we explored whether participants’ sociodemographic characteristics were associated with the duration and amount of content viewed within each of the four theoretically-informed sections of the intervention: (I) knowledge-related content regarding HIV/STI infection and testing; (II) motivations and decisional balance regarding HIV/STI testing; (III) addressing barriers to HIV/STI testing and recognizing participants’ personal strengths, and; (IV) an HIV/STI testing site locator in the participant’s community. Knowledge-related content was used more often by participants who reported being single and those who reported a prior STI test, perhaps indicating greater relevance of the content to their lives. Engagement with motivational content, on the other hand, varied based on participants’ overall ability to navigate structural barriers to testing. For example, participants were more likely to use the decisional balance feature to assess the pros and cons of getting tested if they had experienced recent residential instability. Similarly, participants who had never tested for HIV/STIs were more likely to spend more time and have greater engagement with content related to overcoming barriers to HIV/STI testing (20).
In the context of a pilot study, these paradata analyses—knowing what content areas participants engaged with and for how long—proved useful for intervention refinement prior to the larger RCT efficacy trial (21). For instance, content that received little use in the pilot was replaced with new content emerging from HIV prevention advances (e.g., information about PrEP). Furthermore, these theoretically-informed paradata metrics are being monitored in the larger RCT in order to examine how paradata metrics are associated with the ongoing efficacy trial’s outcomes, offering opportunities to test the proposed mechanisms of change through mediation analyses and examine whether the intervention’s effects are subject to dose-response relationships.
A social engagement intervention: HealthMpowerment
HealthMpowerment was a mobile optimized online HIV intervention designed to increase safer sex behaviors among HIV-positive and HIV-negative young Black men who have sex with men (aged 18–30 years) (22). Core components of HealthMpowerment (three social spaces: The Forum, Getting Real, and Ask Dr. W) were designed to encourage interactive sharing to foster peer support and reinforce positive behavioral norms to reduce engagement in condomless anal intercourse. A RCT (n=474) comparing HealthMpowerment to an information-only control website found greater reductions in condomless anal intercourse in the HealthMpowerment arm at 3 months. Stronger effects were tied to HealthMpowerment dosage, which was defined as using the intervention for 60 or more minutes during the 3-month trial (23). Engagement, as assessed through number of logins and total time spent on the site was greater for the HealthMpowerment arm than the control across the mean number of logins (HealthMpowerment: M =8.69, SD =24.28 vs. Control: M =2.36, SD =5.07, P=0.0002; Cohen’s d =0.36) and total time spent on the site (HealthMpowerment: M =86.50 min, SD =205.99 vs. Control: M =22.00 min, SD =58.61; Cohen’s d =0.43).
In reviewing HealthMpowerment’s paradata, we sought to quantify a more granular metric of participant engagement (amount) in order to examine whether engagement with HealthMpowerment content provided additional insights on dosage. For example, do participants help to develop new content (i.e., active engagement; e.g., forums, Ask Dr. W) or engage in researcher-developed content (i.e., passive engagement; e.g., reading content)? As noted in Table 4, we categorized the main features of HealthMpowerment into passive or active engagement metrics. Passive engagement included the number of articles read, risk screeners completed, entries into the health journal, and number of quizzes taken. Active engagement included the number of posts added by a participant to the Getting Real and Forum sections of the intervention, number of “likes” to peers’ posts in the Getting Real section, and number of questions asked to Dr. W. Of the 238 participants randomized to the HealthMpowerment arm, 95 (39.9%) had some passive engagement and 62 (26.1%) had active engagement. As noted in Table 4, this categorization of variables into passive and active engagement yielded good reliability.
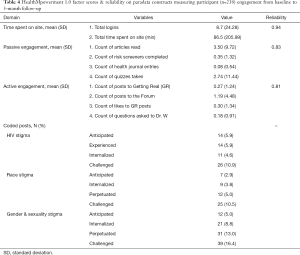
Full table
Throughout the trial, participants in HealthMpowerment authored a total of 1,497 posts to the social spaces. In a social engagement intervention like HealthMpowerment, it is important to understand the nature and quality of the created content by users and explore how participant engagement with user contributed (active) content impacted behavior. Using a mixed methods approach, we qualitatively coded these forum posts. Once coded, we estimated the frequency of stigma-related content (915 posts, 61.1%) and examined what proportion of participants had contributed these posts (see Table 4). Alongside frequency, we examined depth of engagement with stigma content and found that a large proportion of posts included stigma-related conversations regarding anticipated (74/915, 8.1%), experienced (125/915, 13.7%), internalized (410/915, 44.8%), and/or challenged (639/915, 69.8%) stigma regarding sexuality and HIV. Although only a quarter of HealthMpowerment participants (n=62; 26.1%) contributed this new stigma-related content in the forum (see Table 4), a secondary analysis of these data found that these discussions were associated with changes in the overall sample’s HIV-related stigma scores by the 6-month follow-up. Taken together, these findings suggest that participants’ amount of passive and active engagement in the forums, alongside the frequency and depth of the conversations, can influence participants’ perceptions of HIV-related stigma over time.
Discussion
The four cases presented above provide lessons learned regarding the collection and analysis of paradata collected in diverse mHealth HIV prevention and care interventions for young men who have sex with men. Across these studies, evaluation of paradata metrics has guided decision-making related to technology-refinement, helped quantify users’ experiences with the mHealth interventions, enabled examination of how engagement is linked to outcomes of interest, and highlighted the importance of prioritizing and optimizing participants’ engagement in mHealth interventions in future mHealth HIV prevention intervention research.
Duration is the most commonly reported paradata metric, offering insights into the amount of time participants spent using a mHealth intervention. Overall duration, as well with specific intervention components (e.g., time reading theoretically-driven content, time spent interacting in forums, or watching video content), can help to characterize engagement and create thresholds for both brief interventions (e.g., Get Connected 1.0) and interventions designed to be used over time (e.g., AllyQuest). As we move forward, determining a minimal threshold of intervention usage or how intended usage is defined, might help researchers be more realistic regarding the “half-life” of an intervention and invest retention efforts accordingly. Further, it is crucial to evaluate this paradata metric if we are to create products that are used consistently by participants and aim to compete with market-driven programs (e.g., social media platforms). Continued efforts to report duration across mHealth interventions are encouraged; however, duration by itself may provide an incomplete picture of participants’ engagement and should be supplemented by other paradata metrics including frequency of engagement, amount of intervention engagement, and depth of engagement.
The use of paradata to measure frequency (i.e., the rate at which something occurs or is repeated over time) can also inform how users interface and experience mHealth interventions. For instance, how frequently do participants need to engage with different types of intervention activities to maintain engagement? Monitoring frequency of engagement through paradata might get us that answer, help us design better interventions, and reduce costs/investments in components that won’t be used or lead to behavior change. For example, we hypothesized that within an adherence app such as AllyQuest, the medication tracking component would need to be used daily, for about two to three months to ensure habit formation (24,25). However, it may be that use of other components of the app, such as participating in the social discussion or completing a quest is sufficient. Future studies of the app within a larger sample of HIV-positive youth will provide more robust hypothesis testing and perhaps more guidance on how these technologies can impact and sustain healthy adherence behaviors.
Amount of intervention engagement, often measured based on the count of actions taken (e.g., “clicks”) within an intervention, is often used to create a cumulative score denoting participants’ use of content and features. In a secondary analysis of Get Connected 1.0, we used engagement amount to quantify participants’ engagement with the theoretically-driven content, which helped inform the planning of analyses focused on examining whether participants’ amount of engagement with theoretically-driven content influences the key mechanisms of change (e.g., testing motivation, decisional balance) and outcomes of interest in the on-going efficacy trial. Researchers interested in quantifying cumulative engagement amounts, however, must discuss the desired content to be tallied with their intervention development team to ensure that the application’s design and back-end coding captures users’ actions appropriately. In HealthMpowerment, for instance, we were able to characterize participants’ active engagement with the intervention’s content (e.g., number of articles viewed, count of new posts created) and identify associations with intervention outcomes. In the absence of an action (e.g., clicking on a forum post to open it) within an intervention, however, researchers may be limited in their ability to accurately ascertain passive engagement. For instance, we believe participants in HealthMpowerment who passively engaged with intervention content (e.g., reading users’ posts without contributing to the forum) benefited from the intervention, yet we were unable to measure how these “lurking” behaviors in the forum spaces (e.g., a user reading posts without posting or commenting themselves) impacted behavior. Thus, adaptations to the HealthMpowerment platform have been made to account for both active and passive engagement and to allow a more complete analysis of exactly what content users of HealthMpowerment are exposed to over time. Future research examining how active and passive engagement differentially affect HIV-related outcomes is warranted and may inform novel design and behavior change strategies in mHealth interventions.
Within behavioral mHealth interventions, the assessment of engagement depth through paradata may offer a more detailed study of how different intervention components were used and provide greater transparency than self-report alone. Understanding and addressing not simply the time spent within an intervention but the level of engagement with the actual content itself is, however, more challenging to measure. This goes beyond simply assessing the proportion of sessions that are viewed but requires an understanding of the topics participants choose to focus on or those that create discourse within the intervention. In HealthMpowerment, for instance, we observed that depth of discussions regarding different components of stigma (e.g., anticipated, internalized) were linked to key theoretical mechanisms known to affect HIV prevention and care outcomes. Based on these findings, we have invested time to add features to HealthMpowerment’s design and user experience within the forums to reinforce opportunities for these conversations to occur in a new RCT of HealthMpowerment 2.0. Additional mixed-method strategies, including real-time analysis of textual data through natural language processing, to evaluate and test the association between depth and behavioral outcomes are warranted.
As we move towards greater implementation of these technologies across different populations and geographic locations, considerations of how collection and analysis of paradata could be better harmonized should be entertained. Harmonization of measures would allow for the creation of standard feasibility metrics for pilot studies and for comparison of similar interventions (i.e., What metrics should be consistently measured and reported across all mHealth interventions? What metrics should be consistently measured and reported across different behavior change outcomes?). Moreover, harmonization efforts may ensure that intervention effectiveness could be adequately tied to engagement of the right features at the right time. This would allow for the use of paradata to systematically evaluate and optimize mHealth engagement leading to better health outcomes (i.e., Should all interventions targeting adherence report a certain metric? Do diverse engagement metrics carry different weight on health outcomes?). Harmonization and systematic monitoring of these metrics may also help us create user profiles and make just-in-time decisions to support intervention engagement (i.e., Under what threshold of engagement do participants need a booster or a different intervention?). Adaptive trial designs which utilize decision rules that are based on participant characteristics and responses to the intervention can allow for customization and tailoring of intervention strategies. For example, within the pilot AllyQuest study, app usage declined over the course of the trial, with a mean of 4.3, 3.4, 3.0, and 2.8 days of usage during weeks 1, 2, 3 and 4, respectively. What might have been done if this information was evaluated in “real-time”? In its next iteration, AllyQuest will be evaluated within a Sequential Multiple Assignment Randomized Trial (SMART) (26,27). Users will be escalated or de-escalated to or from a more intensive condition (provision of in-app adherence counseling) based on app usage (e.g. medication tracking) and biologic outcomes (e.g., viral suppression). Other trial designs, such as the Continuous Evaluation of Evolving Behavioral Intervention Technologies (CEEBIT) (28), Just-in-Time Adaptive Intervention (JITAI) (29) or Multiphase Optimization Strategy (MOST) (29), may also offer new opportunities to evaluate trial efficacy and dosing using paradata.
Though beyond the scope of this paper, it is relevant to briefly mention the importance of evaluating the metrics of mHealth components that have been included solely in an attempt to increase intervention engagement. Examples include the use of gamification elements (30), financial incentives (31), tailoring of content (32), and inclusion of “push factors” such as reminders or notifications (33). These strategies have all shown promise in overcoming engagement challenges with mHealth interventions (34). Future studies evaluating how engagement with specific types of intervention activities are linked to behavior change outcomes might inform best practices moving forward, yet will require researchers to systematically report these metrics in their publications.
Conclusions
Online interventions are designed to reach large numbers of users across diverse regions, increase participants’ convenient access to HIV prevention and care tools, and promote iterative learning. Evaluation approaches examining changes in HIV risk behaviors will require researchers to consider both exposure and engagement metrics in their studies.
Acknowledgments
Funding: This publication was made possible through support from the Penn Center for AIDS Research (CFAR; P30 AI 045008), and National Institute of Child Health and Human Development (NICHD) (U19HD089881).
Footnote
Conflicts of Interest: The authors have no conflicts of interest to declare.
Ethical Statement: The authors are accountable for all aspects of the work in ensuring that questions related to the accuracy or integrity of any part of the work are appropriately investigated and resolved.
References
- Couper MP, Alexander GL, Zhang N, et al. Engagement and retention: measuring breadth and depth of participant use of an online intervention. J Med Internet Res 2010;12:e52. [Crossref] [PubMed]
- Perski O, Blandford A, West R, et al. Conceptualising engagement with digital behaviour change interventions: a systematic review using principles from critical interpretive synthesis. Transl Behav Med 2017;7:254-67. [Crossref] [PubMed]
- Brooke J. A quick and dirty usability scale. In: Jordan RW, Thomas B, McClelland IL, et al. editors. Usability Evaluation in Industry. 1st edition. London: Taylor and Francis, 1996:189-94.
- Horvath KJ, Oakes JM, Rosser BR, et al. Feasibility, acceptability and preliminary efficacy of an online peer-to-peer social support ART adherence intervention. AIDS Behav 2013;17:2031-44. [Crossref] [PubMed]
- Crutzen R, Roosjen JL, Poelman J. Using Google Analytics as a process evaluation method for Internet-delivered interventions: an example on sexual health. Health Promot Int 2013;28:36-42. [Crossref] [PubMed]
- Van Gemert-Pijnen JE, Kelders SM, Bohlmeijer ET. Understanding the usage of content in a mental health intervention for depression: an analysis of log data. J Med Internet Res 2014;16:e27. [Crossref] [PubMed]
- Kelders SM, Bohlmeijer ET, Van Gemert-Pijnen JE. Participants, usage, and use patterns of a web-based intervention for the prevention of depression within a randomized controlled trial. J Med Internet Res 2013;15:e172. [Crossref] [PubMed]
- Graham ML, Strawderman MS, Demment M, et al. Does Usage of an eHealth Intervention Reduce the Risk of Excessive Gestational Weight Gain? Secondary Analysis From a Randomized Controlled Trial. J Med Internet Res 2017;19:e6. [Crossref] [PubMed]
- Baltierra NB, Muessig KE, Pike EC, et al. More than just tracking time: complex measures of user engagement with an internet-based health promotion intervention. J Biomed Inform 2016;59:299-307. [Crossref] [PubMed]
- Bauermeister JA, Golinkoff JM, Muessig KE, et al. Addressing engagement in technology-based behavioural HIV interventions through paradata metrics. Curr Opin HIV AIDS 2017;12:442-6. [Crossref] [PubMed]
- Muessig KE, LeGrand S, Horvath KJ, et al. Recent mobile health interventions to support medication adherence among HIV-positive MSM. Curr Opin HIV AIDS. 2017;12:432-41. [Crossref] [PubMed]
- Muessig KE, Nekkanti M, Bauermeister J, et al. A systematic review of recent smartphone, Internet and Web 2.0 interventions to address the HIV continuum of care. Curr HIV/AIDS Rep 2015;12:173-90. [Crossref] [PubMed]
- Mulawa MI, LeGrand S, Hightow-Weidman LB. eHealth to Enhance Treatment Adherence Among Youth Living with HIV. Curr HIV/AIDS Rep 2018;15:336-49. [Crossref] [PubMed]
- Henny KD, Wilkes AL, McDonald CM, et al. A Rapid Review of eHealth Interventions Addressing the Continuum of HIV Care (2007-2017). AIDS Behav 2018;22:43-63. [Crossref] [PubMed]
- Simoni JM, Ronen K, Aunon FM. Health Behavior Theory to Enhance eHealth Intervention Research in HIV: Rationale and Review. Curr HIV/AIDS Rep 2018;15:423-30. [Crossref] [PubMed]
- Hightow-Weidman L, Muessig K, Knudtson K, et al. A Gamified Smartphone App to Support Engagement in Care and Medication Adherence for HIV-Positive Young Men Who Have Sex With Men (AllyQuest): Development and Pilot Study. JMIR Public Health Surveill 2018;4:e34. [Crossref] [PubMed]
- Bauermeister JA, Tingler RC, Demers M, et al. Development of a Tailored HIV Prevention Intervention for Single Young Men Who Have Sex With Men Who Meet Partners Online: Protocol for the myDEx Project. JMIR Res Protoc 2017;6:e141. [Crossref] [PubMed]
- Bauermeister JA, Tingler RC, Demers M, et al. Acceptability and Preliminary Efficacy of an Online HIV Prevention Intervention for Single Young Men Who Have Sex with Men Seeking Partners Online: The myDEx Project. AIDS Behav 2019. [Epub ahead of print]. [Crossref] [PubMed]
- Bauermeister JA, Pingel ES, Jadwin-Cakmak L, et al. Acceptability and preliminary efficacy of a tailored online HIV/STI testing intervention for young men who have sex with men: the Get Connected! program. AIDS Behav 2015;19:1860-74. [Crossref] [PubMed]
- Bonett S, Connochie D, Golinkoff JM, et al. Paradata Analysis of an eHealth HIV Testing Intervention for Young Men Who Have Sex With Men. AIDS Educ Prev 2018;30:434-47. [Crossref] [PubMed]
- Bauermeister JA, Golinkoff JM, Horvath KJ, et al. A Multilevel Tailored Web App-Based Intervention for Linking Young Men Who Have Sex With Men to Quality Care (Get Connected): Protocol for a Randomized Controlled Trial. JMIR Res Protoc 2018;7:e10444. [Crossref] [PubMed]
- Hightow-Weidman LB, Muessig KE, Pike EC, et al. HealthMpowerment.org: Building Community Through a Mobile-Optimized, Online Health Promotion Intervention. Health Educ Behav 2015;42:493-9. [Crossref] [PubMed]
- Hightow-Weidman LB, LeGrand S, Muessig KE, et al. A Randomized Trial of an Online Risk Reduction Intervention for Young Black MSM. AIDS Behav 2019;23:1166-77. [Crossref] [PubMed]
- Lally P, Wardle J, Gardner B. Experiences of habit formation: a qualitative study. Psychol Health Med 2011;16:484-9. [Crossref] [PubMed]
- Gardner B, Lally P, Wardle J. Making health habitual: the psychology of 'habit-formation' and general practice. Br J Gen Pract 2012;62:664-6. [Crossref] [PubMed]
- Almirall D, Nahum-Shani I, Sherwood NE, et al. Introduction to SMART designs for the development of adaptive interventions: with application to weight loss research. Transl Behav Med 2014;4:260-74. [Crossref] [PubMed]
- Collins LM, Nahum-Shani I, Almirall D. Optimization of behavioral dynamic treatment regimens based on the sequential, multiple assignment, randomized trial (SMART). Clin Trials 2014;11:426-34. [Crossref] [PubMed]
- Mohr DC, Cheung K, Schueller SM, et al. Continuous evaluation of evolving behavioral intervention technologies. Am J Prev Med 2013;45:517-23. [Crossref] [PubMed]
- Pham Q, Wiljer D, Cafazzo JA. Beyond the Randomized Controlled Trial: A Review of Alternatives in mHealth Clinical Trial Methods. JMIR Mhealth Uhealth 2016;4:e107. [Crossref] [PubMed]
- Hightow-Weidman LB, Muessig KE, Bauermeister JA, et al. The future of digital games for HIV prevention and care. Curr Opin HIV AIDS 2017;12:501-7. [Crossref] [PubMed]
- Haff N, Patel MS, Lim R, et al. The role of behavioral economic incentive design and demographic characteristics in financial incentive-based approaches to changing health behaviors: a meta-analysis. Am J Health Promot 2015;29:314-23. [Crossref] [PubMed]
- Crutzen R, de Nooijer J, Brouwer W, et al. Strategies to facilitate exposure to internet-delivered health behavior change interventions aimed at adolescents or young adults: a systematic review. Health Educ Behav 2011;38:49-62. [Crossref] [PubMed]
- Alkhaldi G, Hamilton FL, Lau R, et al. The Effectiveness of Prompts to Promote Engagement With Digital Interventions: A Systematic Review. J Med Internet Res 2016;18:e6. [Crossref] [PubMed]
- Wagner B, Liu E, Shaw SD, et al. ewrapper: Operationalizing engagement strategies in mHealth. Proc ACM Int Conf Ubiquitous Comput 2017;2017:790-8.
Cite this article as: Hightow-Weidman LB, Bauermeister JA. Engagement in mHealth behavioral interventions for HIV prevention and care: making sense of the metrics. mHealth 2020;6:7.