Capturing the trend of mHealth research using text mining
Introduction
Mobile technology is readily available to individuals worldwide. In the U.S., 95% of adults have cell phones and 77% have smartphones as of February 2018 (1). With their increasing presence and continuous advances over the years, these handheld devices offer the potential to transform the landscape of health-care delivery (2). According to the WHO Global Observatory for eHealth, mHealth is defined as “medical and public health practices supported by MDs, such as mobile phones, patient monitoring devices, personal digital assistants (PDAs), and other wireless devices” (3). This may include but is not limited to the use of mobile phones, wearable monitoring devices, personal digital assistants, and other wireless devices to monitor patients and assist in the delivery of health information (4).
Mobile health has been used to prevent diseases (5) through educational and motivational messaging regarding healthy lifestyle choices, disease risk factors (6), and the importance of treatment and medication adherence (7). Mobile technology also allows individuals to self-monitor and regulate their health practices, often without the involvement of a health-care professional, which has been proven to be beneficial in areas where resources, like hospitals and clinics, are scarce and where mobile technology is prevalent (8).
mHealth has attracted researchers from various fields, and the number of research publications, including systematic reviews, has increased (9). For example, 290 systematic reviews related to mHealth were published in Web of Science from January 2018 to March 2019. Jusoh (9) found in a systematic review that research into mHealth apps has focused on (I) the prevention of specific medical conditions, such as diabetes (10), gout (11), chronic obstructive pulmonary disease (12), bipolar disorder (13), inflammatory bowel disease (14), and congenital heart disease (15), and (II) improving well-being (16). A review study (17) found that, historically, three distinct time periods have marked the types of mobile devices used in mHealth research. Personal digital assistants were used before 2007, basic and feature phones were used from 2007 to 2012, and smart devices (smartphones, tablet PCs, and iPod Touches) have mainly been used since 2012.
Although existing systematic reviews offer information about the evolution of mHealth, Ozaydin et al. (18) determined that some were too specific to a particular patient population, medical condition, or geographical location. They also found that, in most reviews, researchers conducted a manual categorization of subject areas, themes, and ontologies, which is challenging because of the large number of studies in this rapidly growing area of research. Text mining can categorize a large number of studies because an automated or semi-automated process can reveal the underlying patterns and trends (19). Text mining, in particular, can discover hidden patterns, important concepts, and themes within the literature, since such publications are often text heavy (20).
A few studies have used text-mining techniques to identify trends in mHealth research. One study (18) explored the evolution of mHealth research by text mining 5,644 abstracts. The main objectives of that study were to understand the trends in mHealth research and to define a global perspective of the past, present, and future of mHealth research as an academic field. Their findings revealed the existence of ten clusters in mHealth research. However, the study explored the most frequently surfaced terms using clusters, which has limitations in determining the details of relationships among terms and in producing in-depth understanding.
Therefore, this study aims to answer the following research questions:
- RQ1 What medical conditions, interventions, and study populations have been studied in mHealth research?
- RQ1-1 What medical conditions were studied in mHealth research by year of publication?
- RQ1-2 What interventions were used in mHealth studies by year of publication?
- RQ1-3 What populations were studied in mHealth studies by year of publication?
- RQ2 How are those medical conditions, interventions and study populations, and medical conditions interrelated in mHealth studies?
- RQ2-1 Which interventions were used to study each medical condition in mHealth interventions?
- RQ2-2 Which research populations were studied for each medical condition?
Methods
Dataset
This systematic review used text-mining techniques to assist in identifying terms related to research methods, populations, and medical conditions studied in mHealth research. Web of Science was searched using the terms “mHealth” and “mobile health.” The search was restricted to peer-reviewed journal articles in English published between 2008 and 2018. A total of 5,600 journal articles were retrieved and downloaded from Web of Science.
Analysis
The title and abstract of each article were used in the analysis. KH Coder 3 (21), an open source software package, was used for the text mining. In the first step, 39,292 terms were extracted using the text-mining tool after removing stop words (e.g., %, kg, and mg/L). Variations in terms were combined using the software’s embedded functionality. For example, the terms “eyes” and “eye” were merged as “eye.” In the following step, researchers independently reviewed the extracted terms to identify meaningful terms related to medical conditions (e.g., mood disorder, neoplasms, and injury), types of intervention (e.g., cell phone, SMS, and face-to-face), and study populations (older adults, children, and female). Disagreements were resolved by discussion. If no agreement was reached for a term, it was excluded from the analysis. In the final step, the identified terms were imported into a text-mining tool to measure the productivity of the research as well as to explore the relationships among intervention, medical conditions, and study populations. A full list of the terms used is given in the Supplementary. In this paper, only the top ten identified meaningful terms were addressed. Synonyms and substrings were considered when computing the productivity and relationships among the identified medical conditions, research methods, and study populations. For medical conditions, MeSH Subject Headings 2019 (22) and tree structures were referenced to group them. For example, for bipolar disorder, the substrings “bipolar I disorder,” “bipolar II disorder,” and “bipolar disorder” were considered equivalent. “Neoplasmas” represents diseases such as “cancer,” “tumor,” and “cysts” according to the MeSH tree structures. Regarding interventions, the intervention taxonomy was referenced (23).
The mHealth research by year and latent relationships among research methods, studied medical conditions, and study populations were measured using the following text-mining techniques:
- Document frequency (DF) was used to measure the productivity of the identified medical conditions, research methods, and study populations. The DF is the number of documents (i.e., journal articles in this study) within which a term occurs.
- A co-occurrence network was used to discover relationships among the targeted medical conditions, research methods, and research populations. A co-occurrence network, which is produced by statistical analysis, represents the associative relationships between terms based on their paired presence within a specified unit of text (24,25).
The degree of similarity was determined using Jaccard’s coefficient (26) and the betweenness centrality (27). The Jaccard coefficient indicates the similarity of paired terms based on the frequency of the pair in a document. The value varies between 0 and 1, where 1 indicates maximum similarity and 0 indicates maximum dissimilarity (28). The betweenness centrality of a node is a measure of centrality in a graph based on the number of shortest paths between a pair of vertices that go through the node (29). In other words, two nodes in a network may be connected via an intermediate node with a higher betweenness centrality. A node with a higher betweenness centrality score has more control over the network because more information passes through it (30).
Results
Terms related to medical conditions used in mHealth research
A total of 48 different types of medical conditions were identified in the dataset. The majority of medical conditions among the top ten medical conditions were non-communicable diseases (NCDs) (84.1%). Only two medical conditions were related to communicable diseases (15.9%). Mood disorders (e.g., depression, mood swings, and mood dysregulation) appeared to be the most frequently studied medical condition from 2008 to 2018 (DF =544, 9.7%), followed by diabetes (DF =525, 9.4%) and infection (DF =474, 8.5%). These medical conditions appeared to be the most frequently adopted as a study context in mHealth. Table 1 shows the top ten most frequently studied medical conditions out of the 48 medical conditions identified in the mHealth literature during the last decade. Rest of medical conditions are showed in Supplementary. When examining the publication productivity by year, mood disorders was the most common recently, whereas studies on infection and neoplasms (e.g., cancer, tumor, and cysts) were more frequent in 2008. mHealth research into mood disorders has increased gradually and became the most popular topic beginning in 2016. In 2008, only four articles (6.6% of 61 articles) concerned mood disorders. The number of publications on mood disorders in 2016 was 63 (11.2% of 561), 71 in 2017 (9.3%), and 175 in 2018 (11.7%). In contrast, the percentage of studies on neoplasms (10 out of 61, 16.4%) and infection (8 out of 61, 13.1%) was highest in 2008. The number of articles on these medical conditions was larger in 2018: 84 (5.6% of 1,497) on infection and 97 (6.5% of 1,497) on neoplasms. However, the proportion of articles on these two medical conditions dropped, which implies that mHealth researchers focused on mood disorders rather than neoplasms and infection. Figure 1 visually represents the productivity of journal articles by year.
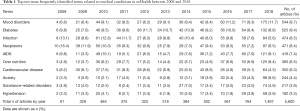
Full table
Terms related to interventions used in mHealth research
A total of 30 different interventions used in mHealth studies were found. Cell phone appeared to be the most frequently used intervention type, appearing in 1,049 journal articles (18.7%) published in the last decade. This was followed by SMS intervention (DF =825, 14.7%), involving messaging and texting, Internet-based intervention (DF =728, 13.0%), and hospital/clinic/operating room-based intervention (DF =510, 9.1%). Table 2 shows the top ten most frequently identified terms related to intervention techniques in mHealth research. Rest of interventions are showed in Supplementary.
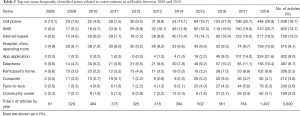
Full table
When examining publication productivity by year, the top three most common intervention techniques—cell phone-, SMS-, and Internet-based interventions—have gained popularity in the last five years among mHealth researchers. In particular, app application-based intervention was very low or nonexistent before 2012. But in 2018, this intervention technique became more common than both SMS- and Internet-based interventions. Figure 2 visually represents the publication of journal articles by year.
Terms related to study populations used in mHealth research
“Female” appeared to the most frequently used term regarding studied populations in mHealth research in the last decade. Approximately 20% of the studies (DF =1,132) had a female population. Next most common were health-care workers (e.g., doctors, general practitioners, medical staff, clinicians, and nurses), children, and outpatients. Table 3 shows the top ten most frequently identified terms related to study populations in mHealth research between 2008 and 2018. Rest of study populations are showed in Supplementary.
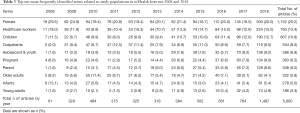
Full table
Looking at the productivity by year reveals that female populations have been consistently popular. In 2008, female populations were more common than in later years, being mentioned in 29.5% of the journal articles (18 out of 61). In contrast, mHealth research on children and health-care workers was relatively less popular than females but relatively more popular and consistent than other study populations. Young adults were relatively less frequent among the frequently studied populations. See Table 3 and Figure 3 for details.
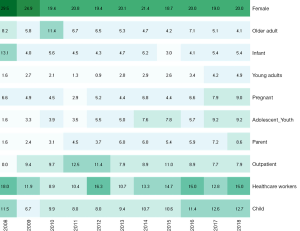
Relationships among medical conditions and interventions
To identify the interventions used for each medical condition, the relationships among the top ten interventions and the top ten medical conditions were examined. The co-occurrence analysis indicated that cell phone-based intervention serves as the center of the network, showing that this type of intervention is not only the most popular intervention technique but also used for other types of intervention, sending messages (Jaccard =0.31) and serving as a base of app applications (Jaccard =0.23) (Figure 4). Cell phone-based intervention appeared to be applied in various medical conditions, such as diabetes, AIDS, anxiety, and mood disorders. Diabetes patients also appeared to be managed using telephone, SMS, and the Internet. Mood disorders and anxiety patients appeared to be managed using the Internet and mobile applications more frequently.
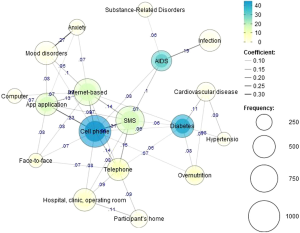
Relationships among medical conditions and study populations
Co-occurrence between the top ten medical conditions and the top ten study populations revealed that females appeared to be studied in the widest range of medical conditions, including pregnancy issues, overnutrition, neoplasms, and AIDS. Pregnancy issues appeared to have the strongest relationship with mHealth studies for female populations (Jaccard =0.22). This similarity is relatively higher than that for relationships between any other medical conditions and female populations. Neoplasms (Jaccard =0.9) and overnutrition (Jaccard =0.9) had slightly stronger relationships with female populations than AIDS (Jaccard =0.8). Outpatients had the second strongest betweenness for diabetes (Jaccard =0.8) and mood disorders (Jaccard =0.8), indicating that these two medical conditions were frequently studied in outpatients. Adolescents have strong relationships with substance-related disorders (Jaccard =0.7) and mood disorders (Jaccard =0.7) (Figure 5).
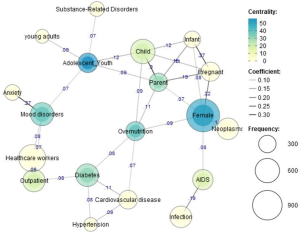
Discussion
Trends in mHealth research were identified using text-mining techniques. The most frequently identified terms related to medical conditions, interventions, and study populations were mined to better understand research trends and gaps in mHealth research. In addition, the relationships between these identified terms were determined from the analyses to identify the interventions and populations studied for different medical conditions. Titles and abstracts of 5,600 journal articles from Web of Science published between 2008 and 2018 were analyzed.
Terms related to medical conditions in mHealth research
First, the types of medical conditions studied in mHealth research from 2008 to 2018 were determined. The majority of the medical conditions addressed in this review study were NCDs. In 2018, mood disorders and overnutrition were the most frequently identified medical condition-related terms in mHealth research whereas infection was a relatively less studied medical condition. This study’s findings are in line with those in other studies (17,31,32). One study (31) reviewed published mHealth research in India from 1997 to 2017. In the study, almost half (44.6%) of the selected articles were related to NCDs. After 2012, the number of NCD-focused articles sharply increased while research related to communicable diseases comprised less than 15 percentages. Another study (32) found that NCDs were commonly addressed in mHealth research in China whereas few research articles focused on infectious disease. Ali’s study (17) also identified NCDs as a clear trend in mHealth research.
In this study, the percentage of mHealth studies related to anxiety and overnutrition has been gradually increasing whereas the percentages of studies on neoplasms and infection have decreased. Interestingly, hypertension has consistently been addressed by a small portion of publications (lower than 4.0%), whereas attention to diabetes appeared to be relatively consistent (between 7.6% and 11.7%) over the last 11 years. This implies that researchers have recently switched to looking at mood disorders, overnutrition, and anxiety from infections and neoplasms. According to the World Health Organization (WHO) (33), the top ten medical conditions causing deaths in 2016 were ischemic heart disease or coronary artery disease, strokes, chronic obstructive pulmonary disease, lower respiratory infections, Alzheimer’s disease or dementia, lung cancer, diabetes mellitus, road injuries, diarrheal disease, and tuberculosis. Thus, mHealth research should be conducted into these medical conditions to decrease mortality. This study found that mental illnesses, such as mood disorders and anxiety, have increasingly been studied in mHealth research over the last 11 years. A possible reason is that mental illness became prevalent. The Anxiety and Depression Association of America (34) reported that 322 million people worldwide had depression in 2018 compared to 264 million in 2017. Despite the increase in mental illness, most patients who need treatment do not receive it (35) because of lack of trained health-care providers (36). In addition, stigma and self-reliance are barriers to seeking treatment and engagement (37). Mobile health, thus, has significant potential for alleviating these medical conditions, making it a common research focus.
Associations between terms related to medical conditions and study populations
Female populations were strongly correlated to pregnancy issues, overnutrition, neoplasms, and AIDS in mHealth research. WHO (38) noted that the top issues for women’s health were cancer, sexual and reproductive health, maternal health, HIV, sexually transmitted infections, and mental health. This fact may be the reason that mHealth research was more focused on issues for women. In this study, outpatients had a strong relationship with diabetes and mood disorders, anxiety, hypertension, cardiovascular disease, and overnutrition. This is not surprising because for chronic medical conditions such as overnutrition and diabetes, quality of life is best maintained by self-managing symptoms and by regular visits to an outpatient department.
Terms related to interventions in mHealth research and associations between terms related to medical conditions and interventions
Among the 30 different interventions used in mHealth studies, cell phone-, SMS- (messaging or texting), and Internet-based interventions appeared to be the most frequently used techniques in this review study. Interestingly, after 2012, mobile application intervention sharply increased and app interventions became more common than cell phone-, SMS- or Internet-based interventions. Similar study findings regarding interventions emerged from another study (17). The study (17) found that PDAs and mobile phones were the most frequently used interventions before 2012, and after 2012 apps were the most widely used interventions in mHealth research. Another study (31) also found that articles using feature phones and smartphones emerged after 2012. mHealth research focused more on smart devices in the last year, and this is not surprising because the increase in smartphone usage globally. In terms of associations between terms related to medical conditions and interventions, mobile applications and Internet-based interventions were applied to mood disorders and anxiety. Telephone-, SMS-, and Internet-based interventions were used for diabetes.
Terms related to study populations
Interestingly, females appeared to be the most frequently studied population in mHealth research in 2018, followed by health-care workers (e.g., doctors, general practitioners, medical staff, clinicians, and nurses), children, and outpatients. A possible reason is that, of the top ten medical conditions studied in mHealth (mood disorders, diabetes, neoplasms, AIDS, overnutrition, anxiety, cardiovascular disease, infection, substance-related disorders, and hypertension), five are related to women’s health (neoplasms especially breast and ovarian, overnutrition, AIDS, mood disorders, and anxiety). Health-care providers were the second mostly frequently selected population term in mHealth research. One study (31) found that physicians were the most often engaged population after a review of 125 articles. Another survey (39) supported the research findings. Most health-care providers, almost 86%, believe that mHealth apps can increase their knowledge of patients’ conditions and reported that they use such apps in practice. Further, 50% of healthcare providers perceived that apps can increase the efficacy of patient treatment and improve the relationship with their patients, especially those with chronic medical conditions, such as diabetes and heart disease. To enhance these benefits, many health-care providers want evidence-based mHealth applications and research is actively being conducted to gather this evidence.
In this study, older adults were relatively less studied in mHealth. One review (40) found that few studies have examined older adults’ perceived attitudes toward or use of mHealth, despite the increasing adoption of digital technology by older people. Another study (41) recognized that research on the use of mHealth apps among older adults has been limited and that there is a need for well-designed studies. According to the Pew Internet Research Center (42), older adults in the U.S. have been slower than their younger counterparts to adopt mobile technology. However, use of this technology by older people is increasing over time as smartphone ownership among this population has increased. Parker et al. (43) noted that innovations in mHealth interventions have the potential to improve physical and mental well-being, to reduce the cost of health care for older adults, and to improve health outcomes for older adults. Thus, it is very important to conduct research to identify barriers to the adoption of mHealth application among older adults.
Conclusions
This study has addressed the current trends in mHealth research using text-mining techniques. The purposes were to identify the most frequently studied medical conditions, interventions, and study populations in mHealth research, as well as to determine the relationships among them. Such a quantitative approach using text-mining techniques with a relatively large amount of data may provide a more accurate overview of what research has been conducted than other approaches. However, this study is not without its limitations. Although the data analysis and interpretation of the results were based on DF—the number of unique journal articles—more than one type of medical condition, intervention, or study population may have been considered in many of the studies. The main limitation of this study was that the dataset was limited, because it contained publications written in English during a period of only 11 years and in one database, Web of Science. Articles related to mHealth have been published in journals from many different fields, such as technological science and information and communication science, because this topic is not limited to health research. Using one database may have influenced this study’s results, which could limit the generalizability of the study findings. Our future study will select published articles from a wide variety of databases and include articles published in different fields. However, the findings may help mHealth researchers to identify research trends and to determine knowledge gaps that have not been explored in previous studies and thus, should be addressed by researchers.
Acknowledgments
None.
Footnote
Ethical Statement: The authors are accountable for all aspects of the work in ensuring that questions related to the accuracy or integrity of any part of the work are appropriately investigated and resolved.
Supplementary
References
- Pew Researh Center. Mobile fact sheet. Washington, DC: Pew Research Center for Internet, Science & Technology. 2018. Available online: https://www.pewinternet.org/fact-sheet/mobile/
- Bert F, Giacometti M, Gualano MR, et al. Smartphones and health promotion: a review of the evidence. J Med Syst 2014;38:9995. [Crossref] [PubMed]
- World Health Organization (WHO) mHealth: new horizons for health through mobile technologies: second global survey on eHealth. Switzerland: Global Observatory for eHealth, 2011. Available online: http://whqlibdoc.who.int/publications/2011/9789241564250_eng.pdf
- Burke LE, Ma J, Azar KM, et al. Current science on consumer use of mobile health for cardiovascular disease prevention: a scientific statement from the American Heart Association. Circulation 2015;132:1157-213. [Crossref] [PubMed]
- Rich E, Miah A. Mobile, wearable and ingestible health technologies: towards a critical research agenda. Health Sociol Rev 2017;26:84-97. [Crossref]
- Piette JD, List J, Rana GK, et al. Mobile health devices as tools for worldwide cardiovascular risk reduction and disease management. Circulation 2015;132:2012-27. [Crossref] [PubMed]
- Gandapur Y, Kianoush S, Kelli HM, et al. The role of mHealth for improving medication adherence in patients with cardiovascular disease: a systematic review. Eur Heart J Qual Care Clin Outcomes 2016;2:237-44. [Crossref] [PubMed]
- O’Shea CJ, McGavigan AD, Clark RA, et al. Mobile health: an emerging technology with implications for global internal medicine. Intern Med J 2017;47:616-9. [Crossref] [PubMed]
- Jusoh S. A survey on trend, opportunities and challenges of mHealth apps. International Journal of Interactive Mobile Technologies 2017;11:73-85. [Crossref]
- Shah VN, Garg SK. Managing diabetes in the digital age. Clin Diabetes Endocrinol 2015;1:16. [Crossref] [PubMed]
- Nguyen AD, Baysari MT, Kannangara DR, et al. Mobile applications to enhance self-management of gout. Int J Med Inform 2016;94:67-74. [Crossref] [PubMed]
- Hardinge M, Rutter H, Velardo C, et al. Using a mobile health application to support self-management in chronic obstructive pulmonary disease: a six-month cohort study. BMC Med Inform Decis Mak 2015;15:46. [Crossref] [PubMed]
- Matthews M, Abdullah S, Murnane E, et al. Development and evaluation of a smartphone-based measure of social rhythms for bipolar disorder. Assessment 2016;23:472-83. [Crossref] [PubMed]
- Con D, De Cruz P. Mobile phone apps for inflammatory bowel disease self-management: a systematic assessment of content and tools. JMIR Mhealth Uhealth 2016;4:e13. [Crossref] [PubMed]
- Schuuring MJ, Backx AP, Zwart R, et al. Mobile health in adults with congenital heart disease: current use and future needs. Neth Heart J 2016;24:647-52. [Crossref] [PubMed]
- Zhao J, Freeman B, Li M. Can mobile phone apps influence people’s health behavior change? an evidence review. J Med Internet Res 2016;18:e287. [Crossref] [PubMed]
- Ali EE, Chew L, Yap KY. Evolution and current status of mHealth research: a systematic review. BMJ Innovations 2016;2:33-40. [Crossref]
- Ozaydin B, Zengul F, Oner N, et al. Text-mining analysis of mHealth research. MHealth 2017;3:53. [Crossref] [PubMed]
- Kim YM, Delen D. Medical informatics research trend analysis: A text mining approach. Health Informatics J 2018;24:432-52. [Crossref] [PubMed]
- Xu J, Ding Y, Song M, et al. Author credit-assignment schemas: A comparison and analysis. J Assoc Inf Sci Technol 2016;67:1973-89. [Crossref]
- Higuchi K. Kh Coder. Free software for quantitative content analysis or text mining. 2001. Available online: http://khc.sourceforge.net/en
- Medical Subject Headings 2019. Available online: https://meshb.nlm.nih.gov/search
- Schulz R, Czaja SJ, McKay JR, et al. Intervention taxonomy (ITAX): describing essential features of interventions. Am J Health Behav 2010;34:811-21. [Crossref] [PubMed]
- Darkes J, Goldman MS. Expectancy challenge and drinking reduction: Process and structure in the alcohol expectancy network. Exp Clin Psychopharmacol 1998;6:64. [Crossref] [PubMed]
- Wachs-Lopes GA, Rodrigues PS. Analyzing natural human language from the point of view of dynamic of a complex network. Expert Syst Appl 2016;45:8-22. [Crossref]
- Jaccard P. Distribution de la flore alpine dans le bassin des Dranses et dans quelques régions voisines. Bull Soc Vaudoise Sci Nat 1901;37:241-72.
- Borgatti SP. Centrality and network flow. Soc Netw 2005;27:55-71. [Crossref]
- Milligan GW. Cluster analysis for researchers. J Mark Res 1985;22:224.
- Newman ME, Girvan M. Finding and evaluating community structure in networks. Phys Rev E Stat Nonlin Soft Matter Phys 2004;69:026113. [Crossref] [PubMed]
- Feldman R, Sanger J. The text mining handbook: advanced approaches in analyzing unstructured data. New York: Cambridge University Press; 2007.
- Bassi A, John O, Praveen D, et al. Current Status and Future Directions of mHealth Interventions for Health System Strengthening in India: Systematic Review. JMIR mHealth and uHealth 2018;6:e11440. [Crossref] [PubMed]
- Tian M, Zhang J, Luo R, et al. mHealth interventions for health system strengthening in China: a systematic review. JMIR MHealth Uhealth 2017;5:e32. [Crossref] [PubMed]
- World Health Organization (WHOa). The top 10 causes of death in 2016. Available online: https://www.who.int/news-room/fact-sheets/detail/the-top-10-causes-of-death
- Anxiety and Depression Association of America. Understand the fact; Depression 2019 Available online: https://adaa.org/understanding-anxiety/depression
- Cuijpers P, Sijbrandij M, Koole SL, et al. The efficacy of psychotherapy and pharmacotherapy in treating depressive and anxiety disorders: a meta-analysis of direct comparisons. World Psychiatry 2013;12:137-48. [Crossref] [PubMed]
- Lipschitz J, Miller CJ, Hogan TP, et al. Adoption of mobile apps for depression and anxiety: cross-sectional survey study on patient interest and barriers to engagement. JMIR Mental Health 2019;6:e11334. [Crossref] [PubMed]
- Bovin MJ, Miller CJ, Koenig CJ, et al. Veterans' experiences initiating VA-based mental health care. Psychol Serv 2018. [Epub ahead of print]. [Crossref] [PubMed]
- World Health Organization (WHOb). Ten top issues for women’s health in 2015. Available online: https://www.who.int/life-course/news/commentaries/2015-intl-womens-day/en/
- Healthcare innovation. Survey: Doctors and Patients See Benefits in Mobile Apps in 2015. Available online: https://www.hcinnovationgroup.com/population-health-management/mobile-health-mHealth/news/13024830/survey-doctors-and-patients-see-benefits-in-mobile-apps
- Andrews JA, Brown LJ, Hawley MS, et al. Older adults’ perspectives on using digital technology to maintain good mental health: interactive group study. J Med Internet Res 2019;21:e11694. [Crossref] [PubMed]
- Vollmer Dahlke D, Ory M. mHealth applications use and potential for older adults, overview of. In: Encyclopedia Geropsychology. 2015:1-9.
- Pew Research Center. Technology use among seniors. Washington, DC: Pew Research Center for Internet & Technology. 2017 Sep. Available online: https://www.pewinternet.org/2017/05/17/technology-use-among-seniors/
- Parker SJ, Jessel S, Richardson JE, et al. Older adults are mobile too! Identifying the barriers and facilitators to older adults’ use of mHealth for pain management. BMC Geriatr 2013;13:43. [Crossref] [PubMed]
Cite this article as: Park H, Park MS. Capturing the trend of mHealth research using text mining. mHealth 2019;5:48.