If we build it, will they come? Issues of engagement with digital health interventions for trauma recovery
Background
Worldwide, exposure to potentially traumatic events is extremely common. Recent epidemiological research across 24 different countries revealed over 70% of respondents had experienced one or more traumatic events (1,2). Of those exposed, 8.7% have a lifetime risk of developing posttraumatic stress disorder (PTSD) and over 15% of developing subthreshold PTSD (3) along with depression, anxiety, and substance use disorders (4). According to the DSM-5, diagnosis of PTSD involves symptoms of intrusions (e.g., nightmares and flashbacks), avoidance, negative alterations to cognitions and mood, and alterations to arousal and reactivity (5). The impairment associated with these disorders can be significant and longstanding (6). Though traditional, trauma informed mental health treatments have been found to be efficacious (7), many individuals do not have access to them (8) or are reluctant to seek treatment (9). Significant barriers to treatment include logistical, geographical, financial, stigma, and other attitudinal challenges (10).
One promising approach to overcoming these barriers is the provision of mental health services via technology that can be readily standardized for wide dissemination of evidence-based care (11). However, engagement with technology-based interventions is a concern and limited participation and high attrition rates are common (12-14). This may be especially true for trauma survivors who often experience symptoms of avoidance and hyperarousal (15). Yet, there is a scarcity of literature examining engagement with technology-based interventions. As the amount of exposure to an intervention is an important prerequisite for intervention outcomes (16), understanding the factors that influence engagement is critical for improving their effectiveness.
The focus of this narrative review is on the complex issue of engagement with digital health interventions (DHIs) and the undercurrent challenge associated with this new wave of technology-based opportunity. Specifically, we review definitions of engagement, conceptual frameworks for engagement, as well as methods to comprehensively measure engagement within the context of trauma recovery. From these frameworks, we propose a testable model of engagement based on social cognitive theory (SCT) that considers the unique challenges of trauma recovery. This review is not intended to provide a systematic or exhaustive set of recommendations, rather it is intended to highlight the challenges of engagement research including its definition, measurement, and modeling.
Literature review method
An informal literature review of titles, keywords and abstracts was conducted between October 2017 and May 2018 to identify papers relating to DHI engagement; no publication date limitations were placed on the review. A search of ERIC, PsychINFO, SCOPUS, Google Scholar, Web of Knowledge, Internet Interventions, and the Journal of Medical Internet Research using different combinations of the terms ‘engagement’, ‘adherence’, ‘attrition’, ‘exposure’, ‘trauma’, ‘digital health intervention’, ‘mental health intervention’, ‘internet’, ‘mhealth’, ‘ehealth’, ‘technology’ and ‘dropout’ was performed. A number of abstracts were excluded as they referred simply to patient engagement or traditional mental health interventions (non-digital). In conducting the review, we were interested in topics pertaining to the definition, measurement, predictors, outcomes, and models of engagement. Therefore, a second search involved manually reviewing the reference lists of the identified articles for these topics. Judgments about the relevance of the articles were made by the first author.
DHIs
DHIs are defined as products or services that use computer technology to promote behavior change and have also been referred to as digital behavior change interventions (17). This technology includes mobile devices and web-based applications and encompasses electronic health (eHealth) and mobile health (mHealth) interventions. DHIs are becoming more and more accessible and as of 2015, the median global internet and smartphone use was 67% and 43%, respectively (18). Importantly, smartphone usage among patients with mental health disorders is slightly higher than the national average, suggesting mental health conditions are not a barrier to technology ownership (19).
Several systematic reviews have shown DHIs to be effective for numerous mental health conditions including anxiety and depression (20), addictions (21), bipolar disorder (22), psychotic disorders (23), panic disorders and phobias (24). Importantly, DHIs are also showing effectiveness in decreasing distress symptoms in trauma survivors (9,25-30). A systematic review of DHIs for the treatment of posttraumatic stress symptoms (N=3,832) found them to be effective but cautioned that there were variations and absences in the reporting of engagement amongst the 33 studies, making it difficult to determine whether participants received a ‘therapeutic dose’ of the DHI. Regardless, they reported small to medium improvements of PTSD symptoms in the active intervention groups independent of the comparison conditions (31). Thus, evidence is mounting supporting the clinical effectiveness of DHIs for reducing clinical symptomatology. However, this is not always the case.
Why study engagement?
Findings on DHI effectiveness are not always positive with many evaluations reporting limited beneficial effects (32-34). There is some evidence that the lack of effectiveness is related to participants not using technologies in the desired way (35). In a systematic review of DHI utilization amongst health care workers several consistent themes emerged, including a high degree of acceptability with a low degree of use (36). DHIs are often plagued by high attrition rates, with attrition rates reaching 60–80% (12,14,37). This is especially true for open access users, who are less likely than trial participants to adhere to the full treatment program (38).
The problem of engagement may be particularly evident with trauma recovery where avoidance is often a critical issue. The self-directed, anonymous usage of DHIs can increase disengagement due to lack of accountability, reactivity due to symptom profile, and lack of therapeutic alliance. The lack of alliance can be especially problematic for those who score high on the re-experiencing and avoidance clusters of PTSD (15).
Indeed, Parish et al. (39) developed a secure, social media website for combat veterans and found that veterans with PTSD demonstrated substantially less engagement (measured by number of logins) with the DHI than veterans without PTSD. During this qualitative study, some veterans reported they discontinued engagement with the site because the online conversations triggered their PTSD symptoms. The authors speculated that trauma avoidance and dissociation, along with the associated cognitive issues (concentration, memory) and comorbid symptoms (substance abuse, depression) might have reduced the likelihood of engagement. A scoping literature review of mobile apps for PTSD concluded that more studies were needed to increase the engagement of these apps by patients with PTSD (40).
Consequently, evaluating engagement has become a high priority and understanding engagement with DHIs has been identified as critical for improving their impact (35,41), yet theoretically based research in this area is sparse (42). A methodological analysis of DHI RCTs for a variety of psychiatric disorders (N=75) cautioned that research on this emerging treatment modality falls short of current standards for evaluating the efficacy of behavioral and pharmacologic therapies (43). The author’s likened the emerging field of DHI research to the era of psychotherapy efficacy research prior to the adoption of methodological standards. A major weakness reported amongst the studies was the lack of attention to engagement where few of the studies (21%) reported the extent to which participants were exposed to the DHI, making it difficult to document the level of intervention received by participants. Only by knowing what engagement is, when it occurs, and under what conditions can we understand what is effective.
What is engagement?
The term engagement has been used in a variety of ways, yielding inconsistent findings and making it difficult to synthesize reliable measures (44). The lack of consistency makes it difficult to determine dose-response relationships (i.e., effective engagement) and compare these relationships between DHIs. A major challenge continues to be the lack of a shared definition and understanding of user engagement and its measurement. This may be due to the complexity of DHI design that requires multidisciplinary input from several areas including clinical mental health expertise, computer interface design, and software development.
In the computer science and human-computer interaction literature, engagement has been conceptualized as the subjective experience of flow, a cognitive and affective state characterized by temporal presence, cognitive absorption, immersion and enjoyment (45). In contrast, the behavioral sciences have conceptualized engagement objectively, focusing on the frequency, duration and depth of usage (16). This difference has resulted in two somewhat dichotomous engagement conceptualizations with one end consisting of subjective measures of user perceptions and experiences and the other end consisting of objective measures, focused on usage and physiological responses. Methods to measure these disparate forms of engagement vary greatly (e.g., self-report questionnaires, ecological momentary assessments, system usage data, sensor data, and psychophysiological measures). Ultimately, the definition of DHI engagement remains “clunky" and lacks the ability to be meaningfully communicated across different disciplines invested in these interventions.
Engagement definition
The study of DHI engagement is a nascent science and developers of DHIs initially defined engagement simply as some form of usage (46). This definition centers on behavior and ignores the cognitive and emotional aspects of engagement. Yardley et al. [2016] (44) emphasized that a key research challenge for these innovative technology approaches is to conceptualize engagement more consistently and comprehensively across a variety of domains. A multi-dimensional definition of DHI engagement is needed that includes behavioral, cognitive and emotional components.
The eCommerce industry places a high value on engagement and defines it as: “a category of user experience characterized by attributes of challenge, positive affect, endurability, aesthetic and sensory appeal, attention, feedback, variety/novelty, interactivity, and perceived user control” (47) (p. 941). This definition focuses primarily on engagement as ‘flow’ without any links to behavior change and confounds predictors of engagement (i.e., aesthetic appeal, novelty, interactivity, feedback, perceived user control) with the experience of engagement.
Recently, a systematic review of the literature on DHI engagement resulted in the following definition: “Engagement with DHIs is (1) the extent (e.g. amount, frequency, duration, depth) of usage and (2) a subjective experience characterized by attention, interest and affect” (41) (p. 258). Focused attention is an important component of engagement and is a measure of concentration and absorption (48). Affect relates to the emotions experienced during an interaction and research has shown that engaged users are affectively involved (47). Interest is categorized by increased cognitive and affective functioning, and persistent effort (49) and has been shown to be strongly related to emotional engagement (50). The Perski et al. definition goes beyond usage to consider aspects of flow. Additionally, it does not ascribe a valence to the measure of affect (i.e., positive).
It is important, especially in trauma recovery, to assess relevant dimensions of attention, affect and interest, depending upon that DHI task. Some trauma recovery tasks appear quite different from others with respect to attention, interest, and affect. For example, when practicing relaxation skills, users may appear unengaged and show low interest, low attention, and positive (or minimal) affect, when in actuality, they are highly engaged with the task at hand (e.g., closing one’s eyes during a deep breathing exercise) (51). On the other hand, when learning how to manage triggers, highly engaged users may indicate high interest, high attention, and negative affect. Therefore, the combination of attention, affect and interest may vary. To promote clarity, we offer the following definition of engagement that expands upon the Perski et al. definition: Engagement with DHIs is (I) the extent (e.g., amount, frequency, duration, depth) of usage; and (II) a subjective experience characterized by relevant dimensions of attention, interest and affect.
Related constructs
In defining engagement, it is also important to delineate what it is not. Constructs theoretically linked to engagement, but distinct from it, include learning, and activation. In addition, predictors of engagement and important clinical outcomes of the intervention are distinct from engagement itself. Clearly distinguishing these processes is critical to clarify the conceptualization of engagement (see Figure 1).
Engagement is a latent construct consisting of objective and subjective measures of usage and experience. Predictors of engagement influence engagement but are not engagement per se. Engaging with a DHI promotes learning of the therapeutic information which can include knowledge about the disorder (e.g., psychoeducation on PTSD), processes maintaining the symptoms (e.g., avoidance), or skills for managing the symptoms (e.g., relaxation). Applying what was learned is referred to as mechanisms of action (i.e., activation) and is predicted to lead to symptom reduction (i.e., outcomes). Outcomes result after applying the new skills to one’s circumstances effectively (52). DHI outcomes are generally measured by a reduction in symptoms (e.g., PTSD).
Learning
Learning is a cognitive factor and is measured by increases in overall understanding of the mental health disorder. Learning can be actual (measured by a test) or perceived (measured by self-report). Engagement has been positively linked to learning in the educational literature (49) but has been largely ignored in the DHI literature. In trauma recovery, this could include learning how to manage triggers, the importance of social support, or what constitutes negative self-talk. Learning alone does not produce outcomes (53). Engagement affects learning which then mediates subsequent mechanisms of action and symptom improvement.
Mechanisms of action
The mechanism of action (i.e., activation) is the application of what was learned and functions as the catalyst for transformation (54). It can be defined both behaviorally and cognitively as the process through which a DHI influences change (55), thus providing a bridge between engagement and the clinical outcomes (56). For example, DHIs for trauma recovery designed to decrease distress symptoms through skills training would measure activation by increases in the practice of these skills (e.g., relaxation skills, identifying automatic thoughts, cognitive challenging, labeling feelings). Activation has also been referred to in the literature as the macro level of engagement (44), although this complicates the definition of engagement.
Based on Bandura’s social cognitive theory (SCT) (57), the proposed relationship between learning, mechanisms of action and engagement is bidirectional in nature (see Figure 1). This bidirectionality is referred to in SCT as triadic reciprocal determinism and suggests that behaviors, cognitive and other personal factors, and environmental events all operate as interacting determinants of each other. These interacting processes influence each other throughout the DHI engagement experience. For example, as learning increases, interest (and thus engagement) may initially increase, then later decrease when there is no longer a need for the intervention. Changes in activation, such as practicing relaxation skills, can also influence engagement with a trauma recovery DHI designed to help one learn to manage trauma triggers or even learn how to cultivate more social support. Individuals who experience low levels of activation may lose hope and choose to disengage. In contrast, those experiencing moderate levels of activation may be inspired by their progress, increase their outcome expectations, and thus, increase engagement.
Predictors of engagement
Predictors of engagement are numerous and can include innumerable combinations of user characteristics, DHI design components, and user perceptions (58). User attributes such as demographics (59), level of psychological distress (60), social factors (61) and technology comfort (62) have been shown to influence engagement. These user related predictors are sometimes referred to as ‘context’ (41), and, at present, it is unclear what user characteristics are related to engagement. Some studies find older age (58) and higher baseline symptoms associated with higher usage of a DHI (60,63). Yet a systematic review found lower symptoms and younger age to be associated with higher engagement (53). There is some evidence that being female and more educated is associated with greater engagement (64,65). Whereas, other studies have shown higher levels of education associated with less engagement (16). Finally, another study found no associations between key demographic factors and engagement (66).
Technical aspects of design can also influence engagement such as persuasive technology (38), tailoring (67), human support (68), and messaging (69). Carolan et al. [2017] found adding social media increased engagement (measured by number of logins) whereas Crutzen et al. [2013] found adding social presence did not increase engagement in terms of the number of pages visited or time online. A recent study found that adding gamification increased both the frequency and duration of usage (70) while another review on the effects of gamification on DHI engagement was unable to draw conclusions due to the inconsistent reporting of engagement (71). Furthermore, Lumsden and colleagues, found no effect of gamification on engagement with their digital-based study (72).
Importantly, there appears to be a unique set of a predictors associated with trauma recovery DHIs. Trauma type and frequency have been found to influence engagement where having experienced a sexually related traumatic event (e.g., rape) or repeated traumatic experiences (e.g., domestic violence, childhood abuse) positively predicted engagement (73). Additionally, PTSD symptom presentation can influence engagement. A recent study found that the PTSD symptom clusters of re-experiencing and negative cognitions were significant predictors of engagement with a trauma recovery DHI (15); whereas the avoidance and arousal PTSD symptom clusters were not significant predictors of engagement. These findings have yet to be replicated.
Engagement and outcomes
Little is known about the influence of DHI engagement on outcomes. Some studies have found greater engagement to be associated with positive change in key outcomes (63). Engagement was related to subsequent smoking cessation in a web-based DHI (67). Funk et al. [2010] found that consistently engaged users were associated with less weight regain (P=0.003) compared to less engaged users (64). Notably, non-responsive participants in this study were contacted by a staff member via telephone to encourage returning to the DHI. Couper et al. [2010] (16) measured breadth and depth of DHI engagement and found that breadth, not depth, significantly predicted positive DHI outcomes (P<0.001).
Other studies do not find such association. In a meta-analysis of 33 studies, Donkin et al. [2011] found the impact of engagement on outcomes appears to vary. They found large variations in the reporting of engagement and the relationship with DHI outcomes. For mental health DHIs, some common measures such as the number of logins, self-reported activities, time online, and pages opened did not show an association between engagement and outcomes (74). Carolan et al. found that increased engagement (defined by number of logins) in a stress management DHI did not result in improved psychological outcomes (75). Results of an RCT targeting stress-related symptoms reported high DHI engagement (number of modules visited) but found no significant differences in outcomes between the intervention group and the wait-list control (76).
These equivocal findings on the predictors of engagement and the relationship between engagement and outcomes accentuate the deficiencies of our current understanding of engagement. A consistent definition and conceptual models are needed to understand the relationship between individual characteristics, engagement, and intervention effectiveness.
Conceptual models of engagement
The primary challenge associated with the scientific exploration of engagement with DHIs is the lack of empirically supported conceptual models to help drive the research. Indeed, much of the research reviewed thus far has not included a theoretical framework or conceptual model in approaching the construct of engagement. In general, models that focus primarily on the relationship between engagement and clinical outcomes fail to consider the dynamic, multi-dimensional aspects of engagement (77). However, some researchers have offered some guidance in this area.
The following is a brief overview of the more influential models and frameworks of engagement. Each considers, to varying degrees, the influence of context, content and delivery on engagement. Some of these models are based on theory but neglect to consider a multi-dimensional definition of engagement. Others are based on the results of systematic reviews but lack a theoretical basis. The frameworks build upon each other and are comprehensive and complex. As a result of this complexity, the first three models reviewed are largely untested, and as such, their validity as cohesive frameworks is unknown. The final model reviewed is based on theory and has some empirical support. From these models, a theoretically based, testable model of engagement is proposed.
Internet intervention model (IIM)
One of the earlier models of DHI engagement offered a theoretical perspective on how user, environmental, and intervention characteristics influence engagement (52). The model, called the IIM, represented a significant advance from the earlier disjointed literature which focused on identifying individual predictors of engagement without consideration of a wider psychosocial and behavioral context. The IIM considered how various aspects including intervention design, technology, quality, user characteristics, and environmental factors influenced engagement. Under this model, engagement mediates behavior change (i.e., activation) which in turn leads to symptom improvement. A shortfall of the IIM is the limited conceptualization of engagement that drew upon research from the behavioral sciences and defined engagement simply as usage. Unfortunately, there is little, if any, empirical investigation of the efficacy of the overall model in terms of predicting engagement.
Model of user engagement (MUE)
New interdisciplinary models of engagement are emerging, but their validity is yet to be established (42). Short et al. [2015], building on previous conceptual frameworks (47,52), suggested engagement is influenced by the environment, individual characteristics, and intervention features. This model, called the MUE, highlights social cognitive influences on engagement such as self-efficacy, perceived relevance, and outcome expectations. Importantly, the MUE took a more holistic approach to engagement and expanded the conceptualization from a purely objective measure of usage to include affect (78). Again, the model is yet to be operationally tested.
Perski engagement framework
Perski and colleagues offered a conceptual framework of engagement that includes DHI technological features, content, mechanisms of action, context and outcomes (41). The proposed framework built off previous work (17,52) and was intended to help generate testable models about how to improve engagement. In this framework they describe several reciprocal processes affecting engagement that include predictors, mechanisms of action, and outcomes. Under this framework, engagement is hypothesized to be influenced by the content, delivery, and context within which it is used (i.e., predictors). Though learning is not explicitly specified in this framework, the article draws a distinction between learning (i.e., intervention receipt) and mechanisms of action. Likewise, an unmeasured third variable (e.g., treatment self-efficacy) is mentioned (though not specified in the framework) as a possible moderator of the relationship between engagement and outcomes.
The strength of the framework is the integrative definition of engagement, the incorporation of the numerous predictors of engagement, and the consideration of the mediating role of mechanisms of action between engagement and outcomes. A potential weakness is the complexity associated with the numerous context, content and delivery options which makes empirical testing challenging. Though comprehensive, empirical support of this framework is currently lacking. Parsimonious, testable models that can accurately capture aspects of this complex framework are needed. One such model, the health action process approach (HAPA), may offer such an opportunity.
HAPA
The HAPA offers a parsimonious theoretical model for understanding engagement with a DHI (79). The HAPA was originally developed to predict engagement in health behaviors and addresses both motivational and volitional processes (80). Similar to the MEU, the HAPA is based on SCT (57). Recently, this framework was adapted to explain engagement with a trauma recovery DHI with interesting results. Using a national sample of trauma survivors, Yeager et al. [2018] found the motivational predictors of outcome expectancies, pre-engagement self-efficacy (i.e., confidence in one’s ability to begin using a DHI), perceptions of need, and symptom severity (e.g., PTSD symptoms) all significantly predicted intentions to engage with a DHI (see Figure 2). Once intentions were formed, planning to use the DHI mediated the translation of intentions into engagement for those low in their perceived ability to continue to use the DHI (60).
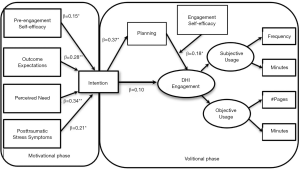
A strength of this model is the empirical support (60,81), the theoretical basis that incorporates social cognitive predictors into the engagement model, and a comprehensive conceptualization engagement. This testable model can be subsumed under the Perski framework where perceived need, outcome expectations, trauma symptoms, pre-engagement self-efficacy, and intentions could be organized under context and delivery (i.e., predictors of engagement). Engagement self-efficacy (i.e., confidence in one’s ability to continue using a DHI) may be the unmeasured third variable thought to moderate engagement referenced in their framework. Planning is considered a self-regulatory strategy that specifies when, where and how a behavior will be implemented (82). A major shortcoming of this model is the lack of consideration of delivery perceptions and outcomes. Extending this model to include delivery, learning, activation and outcomes is an important next step in developing a comprehensive and testable model for engagement.
Proposed research model of engagement
Building upon these conceptual frameworks (41,60,78) we have put forth a comprehensive and testable model for engagement with a trauma recovery DHI (see Figure 3). Fundamentally, this model is based upon the proposed multi-dimensional conceptualization of engagement. This model considers how DHI pre-engagement self-efficacy, perceived need, outcome expectations, PTSD symptom severity influence intentions to engage. Planning mediates the translation of intentions into DHI engagement, moderated by engagement self-efficacy. User perceptions associated with DHI delivery (a measure assessing ease of use, challenge, trust, satisfaction, aesthetics) is also predicted to influence engagement. This is a new SCT predictor in the HAPA model to account for perceptions of user experience with the technology. Although a simplification of previous models, it attempts to incorporate the innumerable content, context and delivery options of the previous frameworks into social cognitive predictors of engagement. We suspect the simplification will increase the testability of the model. Importantly, this model does not conflate engagement with related constructs such as learning and activation. Learning is considered an engagement outcome and an antecedent to activation. Activation is hypothesized to be moderated by levels of treatment self-efficacy where those who experience higher levels of treatment self-efficacy will experience higher levels of activation.
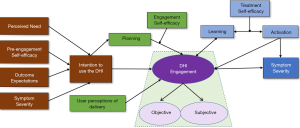
Treatment self-efficacy is intervention specific. In the case of trauma recovery, treatment self-efficacy is represented by one’s confidence in their ability to cope with trauma-related symptoms [(coping self-efficacy for trauma) CSE-T]. Significant research has shown that CSE-T is an important predictor of posttraumatic adaptation (83,84). Individuals who experience low levels of CSE-T would be more reticent to continue engaging, potentially leading to dropout. In contrast, those experiencing moderate levels of CSE-T may increase activation by setting reasonable goals for working with the intervention and gaining mastery experiences through implementing new coping behaviors (e.g., confronting avoidance by managing triggers).
The proposed model provides a means to refine and test hypotheses about how to influence engagement and how engagement impacts outcomes. Previous engagement investigations measured only partial dimensions of engagement (i.e., alternatively objective or subjective) thus failing to consider the complexity of such an experience. Critical to testing this model is a need for valid and reliable measures of engagement that go beyond the simple one-dimensional conceptualization of engagement. Understanding methods to measure the objective and subjective aspects of engagement is a crucial consideration for the scientific advancement of DHI research.
Measuring engagement
The goals of conceptualizing and increasing engagement have motivated an interest in methods to accurately measure it. Identifying and developing multi-dimensional models consisting of valid and efficient combinations of engagement measures continues to be an important challenge of engagement research (17). There is a need for usable, validated, and reliable measures that will enable consistent exploration of engagement predictors that can then inform methods for increasing engagement (47).
Historically, behavior-based metrics (i.e., objective measures) such as page views and time online have been used as indicators of engagement (85). However, intervention exposure alone fails to capture the multidimensional aspects of engagement. The following provides an overview of some of the measurement options available to comprehensibly assess DHI engagement (see Table 1).
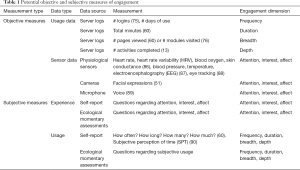
Full table
Objective engagement measures
Objective measures have the advantage of capturing continuous data non-obtrusively. In contrast to subjective measures, objective measures are always on and are independent of the users’ memory, interpretation or social desirability. Objective data can overcome temporal inaccuracies of self-report data and can accurately detect intervention disengagement in real-life environments (91). These advantages can also lead to disadvantages such as massive amounts of data to process (92), complex calculations (91), labor-intensive manually rated training data (51), and issues of confidentiality, privacy and informed consent (93). These analytical, labor, and ethical issues are recognized but are beyond the scope of this paper. Researchers have utilized usage data, sensor data, facial expressions, and voice to automatically capture aspects of DHI engagement.
Usage data
A key ingredient in determining the impact of any DHI is the extent to which participants are exposed to the program (46). Exposure is measured by intervention usage and is the most commonly collected and reported measure of engagement in DHIs. Usage can include both the amount of exposure (i.e., frequency, duration) to the DHI as well as the proportion and extent of intervention features used out of the total available (i.e., breadth, depth). These can provide a measure of physical use of the DHI and analysis can identify usage patterns associated with better outcomes. However, problems arise when users are logged in but not actually using the DHI (60).
Although usage is an essential component of engagement, usage is not always correlated with intervention outcomes (94). Heffner and colleagues examined the usage of specific features of a DHI and found only a small number of the most popular (i.e., high usage) features predicted positive outcomes (95). Surprisingly, one of the most popular features predicted poorer outcomes. The poorer outcomes could be attributable to the ineffectiveness of the feature or to inattention (or even dissociation in the case of trauma survivors) while using the feature. They concluded that popular features are not always predictive of outcomes and continued efforts to understand user engagement was critical for understanding effectiveness.
Sensor data
Another source of objective engagement data is derived from sensors. Smart wearable body sensors (SWS) provide an opportunity to monitor a person’s physical and emotional state automatically and continuously. Sensors have been used to monitor a variety of mental health symptoms (96), but sensing engagement is a nascent research area. Sensors such as skin conductance (86), heart rate, blood pressure, and HRV can monitor changes in sympathetic nervous activity that are associated with attention and emotional arousal. Although some arousal is associated with engagement, too much arousal can produce disengagement, especially for those who have PTSD (97). The optimal arousal range for engagement is often referred to as the window of tolerance (98). For trauma survivors who have difficulties with hyperarousal and avoidance, sensors can be used to determine when individuals are in their ‘window of tolerance’, and hence, most likely to be engaged.
Other physiological measures, such as electroencephalography (EEG) and eye tracking, can also be used to measure engagement. EEG records electrical activity in the brain and has been used as a measure of interest and attention (87). Eye tracking measures pupil dilation and fixation and have been used to indicate task difficulty, attention, fatigue, mental activity, and intense emotion (88). For PTSD, eye tracking may be useful in detecting dissociation.
Facial expressions
Computer vision creates the opportunity to analyze facial expressions. High resolution cameras, now standard in phones and laptops, can be used to automatically estimate real-time engagement from facial expressions. Most of the work in this area focuses on identifying facial action units (AUs) using the Facial Action Coding System (FACS). The FACS is a comprehensive framework for objectively describing facial expression measuring the intensity of over 40 distinct facial muscles (99). AUs can be used to detect emotions and engagement, however, current methods require manual coding of AUs from videos for machine learning training. The current challenge is in automatically recognizing AUs and determining which AUs correspond to engagement with DHIs.
Facial recognition of engagement with DHIs can vary depending on the intervention task or the mental health and emotional distress of the participant, making it particularly challenging. Recently our lab found this to be especially true for trauma-related DHIs (51). For example, while using a trauma recovery DHI, facial expressions expressed while learning how to manage trauma triggers will vary from those expressed while practicing relaxation skills. Traditional engagement predictions may assess AUs associated with relaxation (e.g., eyes closed) as disengaged, however, the individual is actually highly engaged. Dhamija and Boult [2017] utilized computer vision and deep learning-based techniques to predict user engagement with a trauma recovery DHI for two varying contexts (relaxation vs. triggers). Their results suggested that the engagement prediction models needed to assess relevant dimensions of attention, interest, and affect for trauma recovery DHIs, depending on the context.
Voice analysis
Analysis of voice can focus on the content of words, the tone and flow of the spoken words, or the number of words used in communication (100). We analyzed voice pulses (vocal folds vibration) and voice breaks from trauma survivors using an online DHI for trauma recovery (89). Results of this pilot study (N=12) showed that engagement levels depended upon the number of pulses, a percentage of pulses, and percentages of voice breaks (partial eta-squared range: 0.19 to 0.22). Future studies are needed to examine how voice information can be used to assess engagement, specifically attention, interest and affect.
Thus, objective data offers an increasing array of real-time physical, biological and ecologically valid information that can be obtained with little to no individual burden. By combining a variety of sources, objective data can help to detect emotions, physiological reactivity, and monitor behaviors. Importantly, objective measures do not reveal why individuals follow these patterns of usage and are not always correlated with intervention outcomes. Therefore, combining objective with subjective data may be essential to understanding overall DHI engagement.
Subjective engagement measures
Questionnaires can assess engagement experience and usage in a systematic and standardized way. Limitations include questionnaire length and a lack of valid and reliable measures designed and tested within a health context. High quality short targeted engagement questionnaires relevant to DHIs are needed.
Subjective experience
Self-report measures can provide valuable insight into a person’s subjective engagement experience with the DHI. According to the definition of engagement put forth, subjective engagement experience needs to include measures of affect, attention and interest (41).
Several self-report scales have been developed to assess subjective experience. Perhaps due to the lack of a shared definition of engagement, these scales assess one or two dimensions of experience (i.e., affect, interest, attention), but not all. Additionally, many of these scales conflate predictors of engagement with measures of engagement. For example, O’Brien and Toms [2010] developed the user engagement scale (UES) that measures aesthetic appeal, focused attention, satisfaction, and perceived usability (101). Aesthetic appeal, satisfaction, and perceived usability are not indicators of engagement per se but may increase the likelihood of engagement. Interestingly, applications of the UES have had mixed results with items factoring inconsistently on the subscales (48).
Another scale, called the mobile app rating scale (MARS) measures five dimensions related to several DHI experiences that include ‘engagement’, ‘functionality’, ‘aesthetics’, ‘information’, and ‘subjective quality’ on 5-point scales (102). The eHealth engagement scale (eES) encompasses four attributes: involving, credible, negative feelings, and amusing/friendly (103). The consumer videogame engagement scale was developed to measure flow and includes conscious attention, absorption, dedication, enthusiasm, social connection, and interaction (104). A limitation of each of these scales is the conflation of predictors of engagement (e.g., aesthetics, credibility, social connection) with engagement. Valid and reliable engagement scales that measure attention, interest, and affect are needed.
The question remains as to how these subjective engagement experiences relate to trauma recovery. In the scales above, affect is viewed as a hook to induce exploration and discovery where positive experiences bring greater engagement and negative experiences (e.g., frustration) lead to disengagement. However, this assumption must be critically evaluated with different clinical populations such as trauma survivors. Trauma recovery requires some level of confrontation with the trauma itself where accommodation and assimilation eventually occur (105). Maximizing engagement with a DHI for trauma recovery likely requires a unique recipe where critical skill enhancement is offered within a window of tolerance keeping negative affect manageable.
Similar to affect, measuring attention with technology must consider the context. For example, in a game scenario, attention is focused on absorption vs. an online shopping site which is focused on attention grabbing (104). With DHIs for trauma recovery, there may be some combination of attention associated with optimal outcomes, but this has yet to be determined. Our current work is focusing on refining these measures of affect, attention, and interest in a variety of contexts to develop a valid and reliable measure of the engagement experience. Future research which helps to disentangle these important subtleties for DHIs for trauma recovery is critically needed.
Subjective usage
Like objective measures, questionnaires can also be used to assess the amount of DHI usage. Measures for assessing behavioral aspects of DHI engagement are lacking. Regardless of whether objective measures have been captured by the DHI, adding subjective perceptions of usage can offer insight into how much an individual thought they used an intervention. Questionnaires can ask how much (duration) and how often (frequency) an individual used all or parts of the intervention (60). This information can be used to check or complement objective usage data and may help differentiate between active and passive usage. For example, questionnaires can identify when the user has left the program running in the background and not actively using it. Questionnaires can go beyond objective data by capturing information on behavioral cues at the point of engagement (e.g., “what were you doing before you used the relaxation module”?). This may provide a more comprehensive measure of usage patterns.
Moreover, subjective usage can go beyond objective data by capturing the subjective perception of time (SPT). SPT is often referred to as psychological time and can contrast with objective time (106). SPT has been used in attention research and can be an indication of the cognitive aspects of engagement (90). Comparing SPT to actual time may provide unique insights into the level of engagement with a trauma recovery DHI. For example, SPT can accelerate (i.e., flow) or decelerate (i.e., boredom), be interrupted (i.e., distracted), or vanish altogether (i.e., dissociation). Interestingly, Yeager and colleagues found small to medium correlations (r=0.05 to 0.33) between objective and subjective measures of usage, suggesting that subjective measures may be subject to reporting bias or capturing other aspects of engagement beyond usage (e.g., attention, interest, affect). To answer these questions, further studies are needed.
Ecological momentary assessments
Many of the subjective tools used to measure engagement are cumbersome and lack the temporal resolution needed to understand the interplay between engagement and behavior change. Ecological momentary assessments (EMAs) attempt to bridge this gap by capturing the dynamic experiential aspects of engagement (i.e., interest, attention, and affect). The advantage of using EMA over traditional self-report measures is the ability to capture the variability of real-time thoughts, feelings, or behaviors in a naturalistic environment, reducing reporting bias and providing a link between the immediate environment and engagement (107). Problems with EMA revolve primarily around repetition which can lead to high participant burden, practice effects, and non-compliance. Therefore, assessments must be brief and thoughtfully presented.
Chung [2012] (45), successfully used EMA to measure user engagement with a video game, but data on the use of EMAs to study DHI engagement is currently lacking. Despite the limited research, EMAs are well-suited to study engagement with a DHI and could provide real-time measures of affect, attention, and interest as well as antecedents to drop-out.
In sum, several different measures of engagement are available that can quantify the cognitive, emotional and behavioral dimensions of engagement. These measures provide real-time, ecologically valid means for assessing the complex relationship between engagement and the behavioral and technological aspects of DHIs. Investigations focused on DHI’s for trauma recovery that includes objective and subjective measures of engagement are needed. Additionally, the validity and reliability of these measures need to be established (108). The challenge is in combining heterogeneous data sources into a meaningful measure of engagement. This multi-dimensional measurement of engagement can then be used in conceptual models to understand the relationship between individual characteristics, engagement, and intervention effectiveness. Ultimately, adequate measures may provide the opportunity to automatically detect disengagement and help identify factors to improve engagement.
Conclusions
We have highlighted a host of good news and challenges associated with the potential of DHIs for trauma recovery. Although there is supportive evidence for DHIs in reducing psychological distress related to trauma, significant issues remain. According to a recent editorial on the public health impact of DHIs, a greater investment is needed in improving user engagement (35). However, to do so, issues regarding the operationalization and evaluation of engagement with DHIs must be addressed. Specifically, we argue that foundational to future studies is the need for a common multi-dimensional definition of engagement. We offer a comprehensive definition of engagement that encompasses psychology, computer science and business perspectives to address the behavioral, cognitive and emotional aspects of engagement while considering the unique challenges of trauma recovery. This definition highlights the need for in-depth mixed methods research into how people engage. Importantly, it does not confound the definition by including other constructs related to engagement such as learning and activation. This may improve the validity and reliability of engagement measurements. Future research is needed to establish the validity and reliability of this definition. With an accurate and consistent measurement of engagement, it may be possible to empirically establish the level of effective engagement (i.e., dose) required to achieve behavior change. This will allow researchers to compare dose response relationships between various types of DHIs and may help to improve the methodological quality of RCTs evaluating DHIs (43).
Additionally, a conceptual model for engagement has been offered. This model, based upon SCT and previous frameworks (41), is intended to be testable and generalizable and is therefore a simplification of some of the more complex frameworks presented. However, the parsimony of the model may increase the opportunity to establish its validity. Notably missing from this model is the specification of intervention elements and characteristics (56). However, some of these intervention attributes are hypothesized to be incorporated into other constructs such as user perceptions of delivery, self-efficacy (can I use it?), perceived need (do I need it?), and outcome expectations (will it work?). We anticipate the modification of this model as more evidence is accumulated.
Combined, the proposed definition and suggested model provide steps toward formalizing the science of engagement that may help improve the design of engaging and effective digital interventions. As O’Brien and Toms [2008] noted, “successful technologies are not just usable, they engage users.” This may be even truer for trauma recovery DHIs. With a standard conceptualization of engagement along with valid and reliable methods to measure engagement, predictors and outcomes of engagement can be more consistently investigated. Unique individual difference variables related to engagement may then emerge offering a more refined approach to intervention customization. Disengagement can be automatically recognized and responded to by DHIs, enabling interventions to maintain a connection with a user, potentially delivering a more personalized experience (109). The ultimate goal of understanding engagement is to improve the effectiveness of DHIs. A further understanding of learning and activation may also develop that will help dismantle the components contributing to overall intervention effectiveness (56).
With the adoption of more consistent standards for methodological quality, this emerging field has the potential to be widely disseminated and marketed as evidence based care. Future studies are needed to validate measures of engagement and empirically test conceptual models of engagement using multi-dimensional assessments. The future is bright for the role of DHIs in overcoming significant barriers to care for trauma survivors, but only if we are able to clarify the conceptualization and operationalization of engagement.
Acknowledgements
None.
Footnote
Conflicts of Interest: The authors have no conflicts of interest to declare.
References
- Kessler RC, Aguilar-Gaxiola S, Alonso J, et al. Trauma and PTSD in the World Health Organization world mental health surveys. Eur J Psychotraumatol 2017;8. [Crossref] [PubMed]
- World Health Organization. mhGAP intervention guide for mental, neurological and substance use disorders in non-specialized health settings: Mental Health Gap Action Programme (mhGAP). World Health Organization, Geneva, 2010.
- Brancu M, Mann-Wrobel M, Beckham J, et al. Subthreshold posttraumatic stress disorder: A meta-analytic review of DSM-IV prevalence and a proposed DSM-5 approach to measurement. Psychol Trauma 2016;8:222-32. [Crossref] [PubMed]
- Brady KT, Kileen T, Brewerton T, et al. Comorbidity of psychiatric disorders and posttraumatic stress disorder. J Clin Psychiatry 2000;61:22-32. [PubMed]
- American Psychiatric Association. Diagnostic and statistical manual of mental disorders (DSM-5). Am Psychiatr Publ. Arlington, VA, 2013.
- Koenen KC, Ratanatharathorn A, Ng L, et al. Posttraumatic stress disorder in the world mental health surveys. Psychol Med 2017;47:2260-74. [Crossref] [PubMed]
- Barry MM, Clarke AM, Jenkins R, et al. A systematic review of the effectiveness of mental health promotion interventions for young people in low and middle-income countries. BMC Public Health 2013;13:835. [Crossref] [PubMed]
- Saxena S, Thornicroft G, Knapp M, et al. Resources for mental health: scarcity, inequity, and inefficiency. Lancet 2007;370:878-89. [Crossref] [PubMed]
- Litz BT, Engel CC, Bryant RA, et al. A randomized, controlled proof-of-concept trial of an internet-based, therapist assisted self-management treatment for posttraumatic stress disorder. Am J Psychiatry 2007;164:1676-83. [Crossref] [PubMed]
- Kantor V, Knefel M, Lueger-Schuster B. Perceived barriers and facilitators of mental health service utilization in adult trauma survivors: A systematic review. Clin Psychol Rev 2017;52:52-68. [Crossref] [PubMed]
- Ruzek JI, Yeager CM. Internet and mobile technologies: addressing the mental health of trauma survivors in less resourced communities. Glob Ment Health (Camb) 2017;4. [Crossref] [PubMed]
- Eysenbach G. The law of attrition. J Med Internet Res 2005;7. [Crossref] [PubMed]
- Price M, Gros DF, McCauley JL, et al. Nonuse and dropout attrition for a web-based mental health intervention delivered in a post-disaster context. Psychiatry 2012;75:267-84. [Crossref] [PubMed]
- Lie SS, Karlsen B, Oord E, et al. Dropout from an eHealth intervention for adults with type 2 diabetes: A qualitative study. J Med Internet Res 2017;19. [Crossref] [PubMed]
- Yeager CM, Shoji K, Benight CC. The influence of posttraumatic stress disorder (PTSD) symptom clusters on engagement with a trauma recovery eHealth Intervention. Poster session presented at the annual conference of the Int Soc Res on Internet Interv, Chicago, IL, 2017.
- Couper MP, Alexander GL, Zhang N, et al. Engagement and retention: Measuring breadth and depth of participant use of an online intervention. J Med Internet Res 2010;12. [Crossref] [PubMed]
- Michie S, Yardley L, West R, et al. Developing and evaluating digital interventions to promote behavior change in health and health care: Recommendations resulting from an international workshop. J Med Internet Res 2017;19. [Crossref] [PubMed]
- Poushter J. Smartphone ownership and internet usage continues to climb in emerging economies 2016, Available online: http://www.pewglobal.org/2016/02/22/smartphone-ownership-and-internet-usage-continues-to-climb-in-emerging-economies/
- Torous J, Friedman R, Keshavan M. Smartphone ownership and interest in mobile applications to monitor symptoms of mental health conditions. JMIR Mhealth Uhealth 2014;2. [Crossref] [PubMed]
- Deady M, Choi I, Calvo RA, et al. eHealth interventions for the prevention of depression and anxiety in the general population: A systematic review and meta-analysis. BMC Psychiatry 2017;17:310. [Crossref] [PubMed]
- Gainsbury S, Blaszczynski A. A systematic review of Internet-based therapy for the treatment of addictions. Clin Psychol Rev 2011;31:490-8. [Crossref] [PubMed]
- Torous J, Powell A. Current research and trends in the use of smartphone applications for mood disorders. Internet Interv 2015;2:169-73. [Crossref]
- Gire N, Farooq S, Naeem F, et al. mHealth based interventions for the assessment and treatment of psychotic disorders: a systematic review. mHealth 2017;3:33. [Crossref] [PubMed]
- Reger MA, Gahm G. A meta-analysis of the effects of internet- and computer-based cognitive-behavioral treatments for anxiety. J Clin Psychol 2009;65:53-75. [Crossref] [PubMed]
- Benight CC, Ruzek J, Waldrep E. Internet interventions for traumatic stress: A review and theoretically based example. J Trauma Stress 2008;21:513-20. [Crossref] [PubMed]
- Ivarsson D, Blom M, Hesser H, et al. Guided internet-delivered cognitive behavior therapy for post-traumatic stress disorder: A randomized controlled trial. Internet Interv 2014;1:33-40. [Crossref]
- Kersting A, Dölemeyer R, Steinig J, et al. Brief Internet-based intervention reduces posttraumatic stress and prolonged grief in parents after the loss of a child during pregnancy: a randomized controlled trial. Psychother Psychosom 2013;82:372-81. [Crossref] [PubMed]
- Kuhn E, Kanuri N, Garvert D, et al. A randomized controlled trial of the PTSD Coach app with community trauma survivors. J Consult Clin Psychol 2017;85:267-73. [Crossref] [PubMed]
- Littleton H, Grills AE, Kline KD, et al. The from Survivor to thriver Program: RCT of an online therapist-facilitated program for rape-related PTSD. J Anxiety Disord 2016;43:41-51. [Crossref] [PubMed]
- Miner A, Kuhn E, Hoffman JE, et al. Feasibility, acceptability, and potential efficacy of the PTSD Coach app: A pilot randomized controlled trial with community trauma survivors. Psychol Trauma 2016;8:384-92. [Crossref] [PubMed]
- Simblett S, Birch J, Matcham F, et al. A systematic review and meta-analysis of e-Mental health interventions to treat symptoms of posttraumatic stress. JMIR Ment Health 2017;4. [Crossref] [PubMed]
- Kumar S, Nilsen WJ, Abernethy A, et al. Mobile health technology evaluation: The mHealth evidence workshop. Am J Prev Med 2013;45:228-36. [Crossref] [PubMed]
- Marcolino MS, Oliveira J, D'Agostino M, et al. The Impact of mHealth Interventions: Systematic Review of Systematic Reviews. JMIR Mhealth Uhealth 2018;6. [Crossref] [PubMed]
- Newman MG, Szkodny LE, Llera SJ, et al. A review of technology-assisted self-help and minimal contact therapies for anxiety and depression: Is human contact necessary for therapeutic efficacy? Clin Psychol Rev 2011;31:89-103. [Crossref] [PubMed]
- Grady A, Yoong S, Sutherland R, et al. Improving the public health impact of eHealth and mHealth interventions. Aust N Z J Public Health 2018;42:118-9. [Crossref] [PubMed]
- White A, Thomas D, Ezeanochie N, et al. Health worker mHealth utilization: A Systematic Review. Comput Inform Nurs 2016;34:206-13. [Crossref] [PubMed]
- Schubart JR, Stuckey H, Ganeshamoorthy A, et al. Chronic Health conditions and internet behavioral interventions. Comput Inform Nurs 2011;29:81-92. [Crossref] [PubMed]
- Kelders SM, Kok R, Ossebaard H, et al. Persuasive system design does matter: A systematic review of adherence to web-based interventions. J Med Internet Res 2012;14. [Crossref] [PubMed]
- Parish MB, Apperson M, Yellowlees PM. Engaging U.S. Veterans with PTSD in online therapy. Psychiatr Serv 2014;65:697. [Crossref] [PubMed]
- Rodriguez-Paras C, Tippey K, Brown E, et al. Posttraumatic stress disorder and mobile health: App investigation and scoping literature review. JMIR Mhealth Uhealth 2017;5. [Crossref] [PubMed]
- Perski O, Blandford A, West R, et al. Conceptualising engagement with digital behaviour change interventions: a systematic review using principles from critical interpretive synthesis. Transl Behav Med 2017;7:254-67. [Crossref] [PubMed]
- Ryan C, Bergin M, Wells JS. Theoretical perspectives of adherence to web-based interventions: a scoping review. Int J Behav Med 2018;25:17-29. [Crossref] [PubMed]
- Kiluk BD, Sugarman D, Nich C, et al. A methodological analysis of randomized clinical trials of computer-assisted therapies for psychiatric disorders: Toward improved standards for an emerging field. Am J Psychiatry 2011;168:790-9. [Crossref] [PubMed]
- Yardley L, Spring BJ, Riper H, et al. Understanding and promoting effective engagement with digital behavior change interventions. Am J Prev Med 2016;51:833-42. [Crossref] [PubMed]
- Chung J. Temporal presence variation in immersive computer games. Int J Hum Comput Interact 2012;28:511-29. [Crossref]
- Danaher BG, Boles SM, Akers L, et al. Defining participant exposure measures in web-based health behavior change programs. J Med Internet Res 2006;8. [Crossref] [PubMed]
- O'Brien HL, Toms EG. What is user engagement? A conceptual framework for defining user engagement with technology. J Am Soc Inf Sci Technol 2008;59:938-55. [Crossref]
- Lalmas M, O'Brien H, Yom-Tov E. Measuring User Engagement. Morgan Claypool Publishers, Chapel Hill, 2015.
- Ainley M, Hidi S, Berndorff D. Interest, learning, and the psychological processes that mediate their relationship. J Educ Psychol 2002;94:545-61. [Crossref]
- Crutzen R, Ruiter RA, de Vries NK. Can interest and enjoyment help to increase use of Internet-delivered interventions? Psychol Health 2014;29:1227-44. [Crossref] [PubMed]
- Dhamija S, Boult T. Exploring contextual engagement for trauma recovery. IEEE Conf Comp Vision Pattern Recognition 2017:19-29.
- Ritterband LM, Thorndike F, Cox D, et al. A behavior change model for internet interventions. Ann Behav Med 2009;38:18-27. [Crossref] [PubMed]
- Christensen H, Griffiths K. M, Farrer L. Adherence in internet interventions for anxiety and depression: Systematic review. J Med Internet Res 2009;11. [Crossref] [PubMed]
- Kazdin AE. Mediators and mechanisms of change in psychotherapy research. Annu Rev Clin Psychol 2007;3:1-27. [Crossref] [PubMed]
- Michie S, Carey RN, Johnston M, et al. From theory-inspired to theory-based interventions: A protocol for developing and testing a methodology for linking behaviour change techniques to theoretical mechanisms of action. Ann Behav Med 2018;52:501-12. [PubMed]
- Mohr DC, Schueller SM, Montague E, et al. The behavioral intervention technology model: An integrated conceptual and technological framework for eHealth and mHealth interventions. J Med Internet Res 2014;16. [Crossref] [PubMed]
- Bandura, A. Self-efficacy: The exercise of control. Freeman, New York, 1997.
- Beatty L, Binnion CA. Systematic review of predictors of, and reasons for, adherence to online psychological interventions. Int J Behav Med 2016;23:776-94. [Crossref] [PubMed]
- Neil AL, Batterham P, Christensen H, et al. Predictors of adherence by adolescents to a cognitive behavior therapy website in school and community-based settings. J Med Internet Res 2009;11. [Crossref] [PubMed]
- Yeager CM, Shoji K, Luszczynska A, et al. Understanding engagement with a trauma recovery eHealth intervention explained with the Health Action Process Approach (HAPA): Longitudinal study. JMIR Ment Health 2018;5. [Crossref] [PubMed]
- Wang Z, Wang J, Maercker A. Program use and outcome change in a web-based trauma intervention: Individual and social Factors. J Med Internet Res 2016;18. [Crossref] [PubMed]
- Yeager CM, Shoji K, Benight CC. Role of eHealth Readiness and coping self-efficacy on the effectiveness of a trauma recovery eHealth intervention for reducing symptoms of posttraumatic distress. Poster session presented at the annual conf of the Int Soc Res on Internet Interv, Chicago, IL, 2017.
- Fuhr K, Schröder J, Berger T, et al. The association between adherence and outcome in an Internet intervention for depression. J Affect Disord 2018;229:443-9. [Crossref] [PubMed]
- Funk KL, Stevens V, Appel L, et al. Associations of internet website use with weight change in a long-term weight loss maintenance program. J Med Internet Res 2010;12. [Crossref] [PubMed]
- Geraghty AW, Torres LD, Leykin YA, et al. Understanding attrition from international internet health interventions: a step towards global eHealth. Health Promot Int 2013;28:442-52. [Crossref] [PubMed]
- Glasgow RE, Christiansen S, Kurz D, et al. Engagement in a diabetes self-management website: usage patterns and generalizability of program use. J Med Internet Res 2011;13. [Crossref] [PubMed]
- Strecher VJ, McClure J, Alexander G, et al. The role of engagement in a tailored web-based smoking cessation program: Randomized controlled trial. J Med Internet Res 2008;10. [Crossref] [PubMed]
- Baumeister H, Reichler L, Munzinger M, et al. The impact of guidance on Internet-based mental health interventions: A systematic review. Internet Interv 2014;1:205-15. [Crossref]
- Alkhaldi G, Modrow K, Hamilton F, et al. Promoting engagement with a digital health intervention (HeLPDiabetes) using email and text message prompts: Mixed-methods study. Interact J Med Res 2017;6. [Crossref] [PubMed]
- Pramana G, Parmanto B, Lomas J, et al. Using mobile health gamification to facilitate Cognitive Behavioral Therapy skills practice in child anxiety treatment: Open clinical trial. JMIR Serious Games 2018;6. [Crossref] [PubMed]
- Brown M, O'Neill N, van Woerden H, et al. Gamification and adherence to web-based mental health interventions: A systematic review. JMIR Ment Health 2016;3. [Crossref] [PubMed]
- Lumsden J, Skinner A, Coyle D, et al. Attrition from web-based cognitive testing: A repeated measures comparison of gamification techniques. J Med Internet Res 2017;19. [Crossref] [PubMed]
- Yeager CM, Shoji K, Benight CC. Role of trauma type and self-efficacy on eHealth intervention engagement. Poster presented at the APA Tech, Mind Soc Conf, Washington, D.C. 2018.
- Donkin L, Christensen H, Naismith S, et al. A Systematic review of the impact of adherence on the effectiveness of e-Therapies. J Med Internet Res 2011;13. [Crossref] [PubMed]
- Carolan S, Harris PR, Greenwood K, et al. Increasing engagement with an occupational digital stress management program through the use of an online facilitated discussion group: Results of a pilot randomised controlled trial. Internet Interv 2017;10:1-11. [Crossref] [PubMed]
- Ashford MT, Olander E, Rowe H, et al. Feasibility and acceptability of a web-based treatment with telephone support for postpartum women with anxiety: Randomized controlled trial. JMIR Ment Health 2018;5. [Crossref] [PubMed]
- Riley WT, Rivera D, Atienza A, et al. Health behavior models in the age of mobile interventions: are our theories up to the task. Transl Behav Med 2011;1:53-71. [Crossref] [PubMed]
- Short C, Rebar A, Plotnikoff R, et al. Designing engaging online behaviour change interventions: A proposed model of user engagement. Eur Health Psychol 2015;17:32-8.
- Schwarzer R, Luszczynska A, Ziegelmann JP, et al. Social-cognitive predictors of physical exercise adherence: Three longitudinal studies in rehabilitation. Health Psychol 2008;27:S54-63. [Crossref] [PubMed]
- Schwarzer R. Modeling health behavior change: How to predict and modify the adoption and maintenance of health behaviors. Appl Psychol 2008;57:1-29.
- Zarski AC, Berking M, Reis D, et al. Turning good intentions into actions by using the Health Action Process Approach to predict adherence to Internet-based depression prevention: Secondary analysis of a Randomized Controlled Trial. J Med Internet Res 2018;20. [Crossref] [PubMed]
- Craciun C, Schüz N, Lippke S, et al. A mediator model of sunscreen use: A longitudinal analysis of social-cognitive predictors and mediators. Int J Behav Med 2012;19:65-72. [Crossref] [PubMed]
- Benight CC, Bandura A. Social cognitive theory of posttraumatic recovery: The role of perceived self-efficacy. Behav Res Ther 2004;42:1129-48. [Crossref] [PubMed]
- Luszczynska A, Benight CC, Cieslak R. Self-efficacy and health-related outcomes of collective trauma. Eur Psychol 2009;14:49-60. [Crossref]
- Lehmann J, Lalmas M, Yom-Tov E, et al. Models of User Engagement. In: Masthoff J, Mobasher B, Desmarais M, et al. editors. User Modeling, Adaptation, and Personalization. Berlin: Springer, 2012.
- Leonard NR, Silverman M, Sherpa D, et al. Mobile health technology using a wearable sensorband for female college students with problem drinking: An acceptability and feasibility study. JMIR Mhealth Uhealth 2017;5. [Crossref] [PubMed]
- Takeda Y, Inoue K, Kimura MS, et al. Electrophysiological assessment of driving pleasure and difficulty using a task-irrelevant probe technique. Biol Psychol 2016;120:137-41. [Crossref] [PubMed]
- Jennett C, Cox AL, Cairns P, et al. Measuring and defining the experience of immersion in games. Int J Hum Comput Stud 2008;66:641-61. [Crossref]
- Shoji K, Benight CC, Mullings A, et al. Measuring Engagement into the Web-Intervention by the Quality of Voice. Poster session presented at the annual conference of the Int Soc Res on Internet Interv, Seattle, WA, 2016.
- Attfield S, Kasai G, Lalmas M, et al. Towards a science of user engagement (Position Paper). Proc IEEE Int Conf Data Min 2011.
- Saleheen N, Ali AA, Hossain SM, et al. puffMarker: A multi-sensor approach for pinpointing the timing of first lapse in smoking cessation. Proc ACM Int Conf Ubiquitous Comput 2015;2015:999-1010.
- Yamato Y. Proposal of vital data analysis platform using wearable sensor. Proceedings of the 5th IIAE International Conference on Industrial Application Engineering 2017.
- Prentice JL, Dobson KS. A review of the risks and benefits associated with mobile phone applications for psychological interventions. Can Psychol 2014;55:282-90. [Crossref]
- Van Ameringen M, Turna J, Khalesi Z, et al. There’s an app for that! The current state of mobile applications (apps) for DSM-5 obsessive-compulsive disorder, posttraumatic stress disorder, anxiety and mood disorders. Depress Anxiety 2017;34:526-39. [Crossref] [PubMed]
- Heffner JL, Vilardaga R, Mercer LD, et al. Feature-level analysis of a novel smartphone application for smoking cessation. Am J Drug Alcohol Abuse 2015;41:68-73. [Crossref] [PubMed]
- Cornet VP, Holden RJ. Systematic review of smartphone-based passive sensing for health and wellbeing. J Biomed Inform 2018;77:120-32. [Crossref] [PubMed]
- Foa E, Hembree E, Rothbaum B. Prolonged Exposure Therapy for PTSD. New York: Oxford University Press, 2007.
- Corrigan FM, Fisher J, Nutt D. Autonomic dysregulation and the Window of Tolerance Model of the effects of complex emotional trauma. J Psychopharmacol 2011;25:17-25. [Crossref] [PubMed]
- Whitehill J, Serpell Z, Lin YC, et al. The faces of engagement: Automatic recognition of student engagement from facial expressions. IEEE Trans Affect Comput 2014;5:1-14. [Crossref]
- Gravenhorst F, Muaremi A, Bardram J, et al. Mobile phones as medical devices in mental disorder treatment: An overview. Pers Ubiquit Comput 2015;19:335-53. [Crossref]
- O'Brien HL, Toms EG. The development and evaluation of a survey to measure user engagement. J Am Soc Inf Sci Technol 2010;61:50-69. [Crossref]
- Stoyanov SR, Hides L, Kavanagh DJ, et al. Mobile App Rating Scale: A New Tool for Assessing the Quality of Health Mobile Apps. JMIR Mhealth Uhealth 2015;3. [Crossref] [PubMed]
- Lefebvre R, Tada Y, Hilfiker S, et al. The assessment of user engagement with eHealth content: The eHealth engagement scale. J Comput Mediat Commun 2010;15:666-81. [Crossref]
- Abbasi AZ, Ting DH, Hlavacs H. Engagement in games: Developing an Instrument to measure consumer videogame engagement and its validation. Int J Comp Games Tech 2017;2017:1-10. [Crossref]
- van der Kolk BA, McFarlane AC, Weisaeth L. Traumatic stress: The effects of overwhelming experience on mind, body, and society. New York: The Guilford Press, 1996.
- Sucala M, Scheckner B, David D. Psychological Time: Interval length judgments and subjective passage of time judgments. Curr Psychol Lett 2011;26:1-10.
- Burke L. E, Shiffman S, Music E, et al. Ecological momentary assessment in behavioral research: Addressing technological and human participant challenges. J Med Internet Res 2017;19. [Crossref] [PubMed]
- Martey RM, Kenski K, Folkestad J, et al. Measuring Game Engagement: Multiple methods and construct complexity. Sim Gaming 2014;45:528-47. [Crossref]
- Rich C, Ponsler B, Holroyd A, et al. Recognizing engagement in human-robot interaction. Proc IEEE Int Conf Human-Robot Inter 2010:375-82.
Cite this article as: Yeager CM, Benight CC. If we build it, will they come? Issues of engagement with digital health interventions for trauma recovery. mHealth 2018;4:37.