A systematic digital approach to implementation and dissemination of eating disorders interventions to large populations identified through online screening: implications for post-traumatic stress
Introduction
In this paper, we describe an approach to implementation and dissemination that focuses on changing outcomes variables within a large, defined population and attempts to provide cost-effective opportunities and resources—which might include the provision of both digital and traditional interventions—to address individual needs and interests. In this model, teams within organizations invested in improving outcomes use moderator, process, and outcome data to improve quality of care. The model differs from traditional implementation and dissemination models in focusing on a range of potential options and changes for the whole defined population, rather than ensuring that a single effective intervention for a given subset of the population is provided consistent with best practices, and in using intervention outcomes and monitoring teams tasked with helping to ensure the best outcomes for the population. Such teams are now commonplace in most commercial enterprises to increase customer satisfaction and sales. The approach is designed to be dynamic and iterative in the sense that the prevention and intervention system and opportunities are continuously revised to increase reach, engagement, effectiveness, and personalization of interventions. Such models also lend themselves to modern analytic and intervention design methods. We refer to this as a systematic digital approach to defined population-based interventions.
A systematic digital approach to defined population-based interventions
A ‘defined population’ refers to any population with shared characteristics, such as gender, disease, geography, or combinations of such factors. In public health, “defined populations” have outcome targets, such as increasing the number of individuals who are vaccinated, who have reduced cardiovascular risk factors, or who no longer meet criteria for being a “case” and/or a combination of these.
A defined population model does not mean that all individuals within the population are provided the same intervention. In fact, it is assumed that there will be multiple pathways for different individuals and subpopulations, depending on their level of need and interest. ‘Defined population’ targets are particularly useful when they map onto organizations or systems responsible for, or invested in, achieving the desired outcomes, such as a public health department or programs that cover medical coverage for enrolled groups.
As will be described in this paper, the “systematic approach” refers to frequent review of the success of the program in achieving the desired aims and iterating new strategies, as needed, to improve outcomes. We assume that population monitoring and many interventions provided to individuals will be digital, although not exclusively so. We focus on a defined eating disorder population identified through online screening. Many individuals with post-traumatic stress (PTS) are likewise identified through screening. In the following we: (I) discuss how aspects of this model have been applied to one defined population—individuals with eating disorders identified by a nationally disseminated screen, (II) explain how the model could be applied to PTS populations, and (III) conclude with a discussion of strengths and limitations of the model.
Case example: providing better access to effective treatments for eating disorders identified through a national screen
Eating disorders are common and disabling problems, negatively impacting quality of life. Eating disorders affect an estimated 3.5–6.5% and 3–3.5% of women and men, respectively, in the Western world (1,2). Eating disorders are associated with high medical and psychiatric comorbidity and increased mortality with anorexia nervosa having the highest mortality rate of all mental disorders (3). Like post-traumatic stress disorder (PTSD), eating disorders are comorbid with several other problems, and follow a chronic course. Furthermore, risk factors for eating disorders have been identified and shown to be modifiable, with reduction in risk factors associated with reduced eating disorder onset (4,5). Also, like PTSD, effective treatments are available but reach only a small percentage of the affected population. Indeed, less than 20% of individuals with eating disorders report receiving treatment (6). As such, systematic approaches to preventing and treating eating disorders have relevance to those experiencing PTS symptoms and preventing and treating PTSD.
Over the past 10 years, we have been involved in a number of studies to integrate preventive and treatment approaches to populations with high rates of eating disorders (7). The following section describes a case example of applying a digitally-based systematic approach to disseminating and implementing interventions for eating disorders identified through screening. The approach is digital in the sense that the screening and feedback are provided in a digital format and data monitoring is digital as are many of the interventions. This approach builds on a program of research in which we have helped identify risk factors for eating disorders (8), developed and examined interventions to reduce eating disorders, and shown that reduction in eating disorder risk reduces disease onset (4,5). We have also developed an evidence-based screen that can sort users into categories of no risk, low risk, or high risk for an eating disorder, or eating disorder diagnosis groups [i.e., the Stanford-Washington University Eating Disorders Screen (SWED)] (9). These groups can then be linked to relevant interventions for an estimated cost-benefit analysis of this model (10). Our general model for screening and delivering interventions can be seen in Figure 1. One advantage of a population-based approach is that both populations at risk for, or with clinical symptoms, can be identified simultaneously and prevention and intervention programs can be provided as appropriate (7). However, in this paper we only focused on those with clinical symptoms.
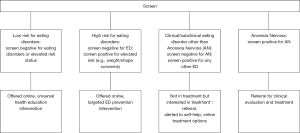
For the purposes of the following discussion, the defined population represents all individuals who completed a national screen who are found to screen positive for an eating disorder but are not currently in treatment (see asterisk for in Figure 1). Defined population reach is the number of these individuals who complete the screen and begin a program of their choosing (i.e., uptake into an intervention). For purpose of clarity, we will use the term ‘reach/uptake’ rather than merely reach and, since individuals can click on a link without beginning it, we define uptake as using >1 session. In this model, targets are established for each phase of identification and screening. The targets are arbitrary and should change as the population recruited changes, rates of uptake, engagement and outcome are determined and new interventions/opportunities added. In theory, everyone who screens positive for an eating disorder and is interested in treatment should begin an intervention. In reality, the available treatment options, cost and other factors make it unlikely that most would do so. In a recent study, about 50% of students who screened positive for an eating disorder clicked on an on-line program (the only option available) (9). Engagement can be defined in various ways, but the most common one, and one we use, is the number of sessions completed. However, as discussed below, early engagement is probably more important than later engagement, and our primary metric is the number of individuals who begin a program (>1 session) and go on to complete 50% of sessions. This is also arbitrary, but for an on-line program, it indicates that students have at least opened the program and moved to the second session. A UK study found that about 50% of individuals referred to face-to-face treatment never initiate treatment (11) so that 50% reach/engaged would seem to be a reasonable target rate for both online and face-to-face interventions. The outcomes we focus on are a significant reduction of symptoms (>50% reduction from baseline) and/or no longer meet case criteria. These criteria are based, in part, of those developed for the VA training program in evidence-based interventions for depression (12) and the effect sizes of the online and face-to-face interventions. Our long-term goal is a significant reduction in the prevalence of eating disorders in this population that would occur through a combination of prevention (not discussed in this paper) and intervention (10). One of the advantages of the model is that it identifies upstream issues (non-acceptance of referrals, for instance, or not showing up) that need to be addressed to provide a significant benefit for the defined population of interest.
The work is ongoing and we discuss how we have been trying to address providing evidence-based treatment to the individuals identified through a national screening effort.
Methods
Screening
In this model, individuals with a potential eating disorder are identified with an evidence-based screen, the SWED (Stanford Washington University Eating Disorder Screen). The screen has been shown to have acceptable sensitivity and specificity (9). Starting in 2016, our research teams based at Stanford University, Palo Alto University, and Washington University in St. Louis partnered with the National Eating Disorders Association (NEDA) to make the SWED screen freely available on their websites in 2017. Individuals are made aware of the screen via NEDA through a variety of social media sites and activities and a weeklong campaign (NEDA awareness week) many US colleges and universities. The screen partitions individuals into no or low risk, high risk of ED onset, or possible ED as per Wilfley et al. (7). (The actual screen can be found at: www.nationaleatingdisorders.org.)
Table 1 lists potential interventions offered for individuals within the defined population. As noted by Munoz (13), interventions can be characterized as (I) self-help (digital/bibliotherapy), (II) coached/guided, (III) teletherapy, (IV) blended therapy (digital/text/e-mail and face-to-face) and V) pure face-to-face (Table 1).
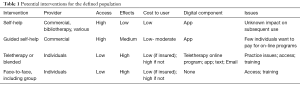
Full table
Briefly
Self-help
Pure self-help programs have the advantage of being inexpensive and readily available as books and online but are associated with high dropout rates and low to moderate effect sizes (14). Little is known about how one might best benefit from a self-help program, if there are downsides, and the possible consequences of failure to improve following self-help and effects on subsequent uptake of more intensive forms of assistance (face-to-face therapy). For instance, individuals who fail to improve with self-help may be reluctant to proceed with more intensive approaches and/or feel like ‘treatment failures’. Likewise, little is known about moderators and mediators that predict better outcomes. Such issues illustrate the potential benefit of a systems model of defined population-level interventions. Moderator and mediator analyses tied to engagement and dropout levels of individuals choosing self-help could be used to identify those who might most benefit from this type of intervention and suggest subpopulations where further trialing could help improve outcomes.
Guided online interventions
Guided online self-help interventions are associated with success rates that are higher than unguided programs and comparable to face-to-face interventions for many mental health disorders (15) including eating disorders (16). Guided online self-help programs for eating disorders are available through commercial companies at a cost of about $50/month per user.
Teletherapy
Teletherapy, as used here, is the provision of psychological services via digital means, including the telephone, text messaging, video or combinations therefore. To reach our widespread population, teletherapy would seem to be a reasonable approach. Teletherapy is often limited by practice guidelines (e.g., therapists restricted from practicing across state lines). In the VA, teletherapy has demonstrated initial efficacy and feasibility (17). In theory, users interested in teletherapy could be given names of providers vetted through some type of quality assurance/best practice model. Teletherapy might be particularly useful when some type of specialized intervention is necessary, such as expertise in dealing with issues relevant to a subgroup, language, disability or diagnosis.
Other options included blended therapy, face-to-face therapy and stepped care models.
Of the options, NEDA currently offers the following for individuals who screen positive for a possible eating disorder: (I) self-help via Recovery Record (18), (II) commercially available guided self-help (www.golantern.com), (III) access via a helpline to a treatment provider database to find a referral to a therapist trained in evidence based practice, and/or (IV) access to a chatline. Respondents are also encouraged to review the information and resources provided on the NEDA website.
Results
From about February 2017 to April 2018, the screen has been completed by over 200,000 individuals. The majority of those completing the screen were classified as being at high risk for eating disorders (ED) onset or having a clinical/subclinical eating disorder (96%). Further, the majority (86%) of individuals screening positive for a clinical/subclinical eating disorder were not currently in treatment. Screens were completed from individuals in most counties of the US and in many places unlikely to have practitioners with training in eating disorder treatment.
Uptake has varied relative to the population screen and the options available, but overall, less than 10% of individuals clicked on one of the options. Of these about 2/3s clicked on the self-help option and about 12% on the guided self-help program. Unfortunately, data are not yet available on the proportion of those who clicked on one of the options who actually meaningfully engaged with the option (e.g., self-help or guided self-help program) or who engaged in in-person treatment as a result of accessing the helpline or chatline. From other sources we can estimate, that for all interventions the numbers would all be below 50%.
Discussion
The first year of our joint efforts working with NEDA suggest that efforts to reach a large number of individuals with eating disorders who are not currently in treatment has been very successful. However, the preliminary data also suggest that few individuals who might benefit from treatment engage in even minimal interventions, such as clicking to learn more about a self-help program [although because of the large reach, large numbers of individuals (i.e., over 10,000) have done so]. In the next phase of our partnership, we are considering evaluating ways to increase reach as seen in line two of Table 2. This is not an exhaustive list. Other analyses will compare (e.g., using ROC analyses) those who choose the various options based on baseline demographics, diagnoses, geographic region, and other variables to help us gain a better understanding of what alterations need to be considered that might improve engagement. Of particular importance, the program does not provide post recommendation motivational interviewing, program selling (e.g., testimonials, promotions), or other interventions that might increase uptake. A broader range of interventions also needs to be considered. If even the low cost of the Lantern program proves to be an obstacle, would free, semi-automated programs increase uptake? While such programs may have smaller effect sizes than guided self-help programs guided by a human coach, if more individuals use them, they may have great value when considering their effects on a population. Since thousands of screens are completed each month, it will be possible to conduct a series of mini-experiments to examine options that might increase uptake such as using machine learning to determine how the helpline can be more effective in motivating individuals to seek online therapy who have access and resources to do so.
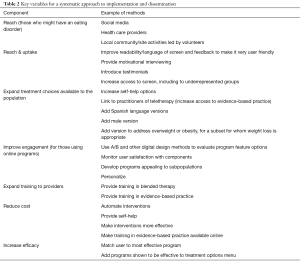
Full table
In parallel studies we have been examining ways to improve engagement in individuals using the new online program. Followed a series of changes based on user feedback and analyses we increased early engagement from 69.5% (n=105/151) to 78.7% (n=70/89). As with engagement, outcome data can be examined in more conventional trials. For eating disorders, as discussed above, self-help programs have been found to have low to medium effect sizes, guided self-help programs moderate to large effect sizes. However, it is important to determine if these effects are maintained when programs are offered to larger populations and NEDA is now collecting program use data from their two main digital referral resources (i.e., Recovery Record, GoLantern). It will also be important to consider other options, such as incorporating face-to-face groups alone or in combination with consumer-led and/or moderated on-line groups. On-going data monitoring should also help us to determine the relative cost/benefit and even harm of the different approaches and to help inform recommendations. Of note, the system we propose should continuously try to improve reach/engagement and outcomes towards the targets of the organization with cost-effectiveness.
Critical components to the model
To the extent this systematic defined population model proves viable, it is worth considering the components of the delivery system that might have led to our success thus far (Table 3). First, the partnering team is diverse and has expertise in areas critical to the design and refinement of interventions. Second, the model is supported by a large, accessible database and the partners have expertise in big data analysis and intervention design. The biggest weakness is that the project’s success depends on the goodwill and working relationships of the partners since it is minimally funded. Furthermore, users need to volunteer to share their data. The model uses baseline, process and outcome data to improve reach, engagement and outcome. While data on users’ intentions to act on referrals is collected, except for the small subsample willing to provide contact information for follow-up, we can only estimate from other programs we provide if respondents began the program, how much of it they used and what the outcomes were. Another option is to partner with companies that select therapists based on their evidence-based training and requires therapists in the network to provide periodic progress data on clients. Another limitation of the defined population model is having a group or organization with adequate resources committed to achieving the population level outcomes. For lack of a better term, we call this the “Outcomes Optimization Team”—those who manage the components of the digital interventions to achieve better outcomes in this population. In our case, it is comprised of the participating teams from Washington University, Palo Alto University, University of Buffalo and NEDA in association with their partners who provide specific expertise. For PTS, it could be national organizations, including health care systems or emergency response agencies.
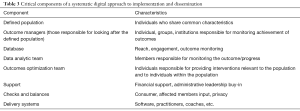
Full table
Universal, targeted/selected prevention and intervention
Although we have focused on the systematic digital approach as applied to clinical cases, the model lends itself to a broader approach that combines universal, targeted/selected prevention and intervention, as illustrated in Figure 1. A strategy that combines both prevention and intervention is most likely to achieve reduction of prevalence of a disorder in a population, which is the most difficult, but perhaps the most important outcome for a population (10). Social media could also be leveraged effectively in this model for such things as increasing the reach of the screen, providing education around relative issues, encouraging activism around important issues, and creating supportive networks.
Application to PTS
The population-based strategy described above for treating eating disorders could be applied to assisting those experiencing problems related to trauma exposure and PTS. An advantage of the strategy is its operational definition of the population in terms of individuals who have completed a screening process, and this same approach might usefully be extended to PTS initiatives. Many existing traumatic stress response systems invoke implicit systems models applied to populations. The Veterans Health Administration (VHA) focuses much of its mental health resources on Veterans exposed to combat and other war-related stressors, and brief screening for PTSD is a standard practice in primary care settings and elsewhere in VA and the Department of Defense. Disaster mental health responses typically focus on large groups of survivors of a specific traumatic event (e.g., 9/11 attacks, Hurricane Katrina), and screening processes taking place online or in crisis counseling settings could be used to identify populations of interest. Below, we discuss how a screen-based defined population approach could be directed to individuals with PTS enrolled in a health care system. Similar methods could be used with other traumatized populations, such as those affected by a disaster within a defined geographic area.
Systematic interventions for individuals with PTS within a health care system
In this example, the focus would be on individuals with PTS served by a health maintenance organization (HMO), characterized as a health system with a defined network of providers and services. The defined population would be identified by a trauma screen, such as the PC-PTSD (19), made available to members of a health care system through screening at health care appointments, provider websites, and even notifications to members. As with the eating disorder case example, the defined population would be those people screening positive for PTS. Reach would be defined as the number of eligible members who engage in the intervention, and the outcome measures related to effectiveness would focus on reduction in PTS symptoms 6 months post screening.
The set of interventions considered for implementation and study would be like those for eating disorders: self-help, guided/coached self-help, teletherapy, blended therapy, and face-to-face. Initial intervention selections would likely include self-help, guided self-help, teletherapy, and face-to-face interventions. Many websites provide trauma survivors with information and self-care recommendations, and there are a number of self-help manuals available. Increasingly, phone apps and internet interventions designed for self-management are being made available. For example, the national center for PTS within the US Department of Veterans Affairs has developed a range of smartphone apps (e.g., PTSD Coach, PTSD Family Coach, PE Coach) designed to assist Veterans (and others) with PTSD. Guided, coached interventions are also available for PTS and have been shown to be effective (20,21). Research supports comparability of face-to-face and teletherapy-delivered treatments provided to PTS populations (17) and teletherapy has been widely implemented for PTSD in VA. By contrast, blended therapies have as yet seen little application and their widespread implementation, at least initially, would be challenging.
As with eating disorders, face-to-face treatments remain the dominant model of service delivery for PTS, and as with eating disorders, there are a variety of limitations of this treatment modality at present. First, although evidence-based interventions have been developed and specified in clinical practice guidelines, they are not routinely available and most practitioners have not been trained in these interventions. Costs may limit their accessibility for low-income groups. The treatments themselves often require around 12 weeks to deliver, and may be inconvenient or unpersuasive for some users. These treatments are typically individualized and therefore cannot be delivered more cost-effectively to larger groups. Many of those with problems are unwilling to seek care due to stigma and other factors, and once initiated, dropout rates from face-to-face treatment are high.
For an HMO population, a team would need to be responsible for enacting and monitoring the interventions. Data analytic, intervention(s) monitoring, outcomes, and training teams would need to be established, and first line interventions would need to be chosen based on evidence, institutional resources, and other factors (e.g., patient preferences). As with eating disorders, stages of implementation might occur sequentially. Initial implementation might include delivery of a specific combination of screening, unguided self-help, guided self-help, teletherapy/blended therapy, or face-to-face treatment.
Reach/engagement and effectiveness rates would be monitored and improved for each intervention based on ongoing reach, engagement and outcomes data, with interventions modified and new treatment options and supportive activities added as appropriate (e.g., asynchronous, synchronous on-line groups, face-to-face groups, family psychoeducational resources). Such monitoring data are likely to be eye opening. For instance, in an older study of 20,284 veterans newly diagnosed with PTSD, 50% (n=10,127) were prescribed a psychotropic medication but only 39% (n=7,980) received some counseling. Only 24% (n=1,909) of those who received any counseling had at least eight counseling sessions. In all, 33% (n=6,616) of those who received a diagnosis of PTSD received a minimally adequate treatment trial in the subsequent 6 months (22). If this population had been enrolled in a defined population model, then the focus might be on increasing counseling, which might have necessitated including other approaches more amenable to the population. For instance, increasing the number of individuals receiving any counseling might be achieved by adding teletherapy and blended programs to make access easier for enrollees. Such findings have led to attempts to increase the reach of evidence-based treatments in the VA (23).
An HMO might engage in a more ambitious program of trying to reduce PTS prevalence in the population. In this case, preventive and educational resources would be added to screening and other activities, as in the model shown in Figure 1. Screening itself could focus on exposure to recent traumatic events as well as PTS reactions. For those with no PTS symptoms, general information about how to be supportive of family members and other services would be provided. For those at risk for PTS (i.e., those recently exposed to traumatic events such as life-threatening illness, accidents, assault, or sudden death of a loved one), PTS prevention and brief intervention programs could be offered in emergency rooms (24) or in primary care settings. In theory, the effects of an integrated prevention and treatment program on the prevalence of PTS could be monitored by routine screening provided to the population.
We note that reach is a major issue in any consideration of the broad effectiveness of an intervention intended to serve a population (25). A problem of many interventions for trauma survivors, and especially of research studies conducted to evaluate interventions, is that only a very few members of the population of interest can participate in the interventions. For example, Shalev et al. (26) contacted 4,224 individuals within 3 weeks of experiencing a traumatic event. Individuals reporting distress who could attend traditional psychotherapy and did not have conflicting medical conditions were invited to treatment. Of those contacted, 73% were not eligible, 18% refused to participate, and 9% were invited to treatment. Price et al. (27) pointed out that a strength of technology-based interventions is the greater potential reach of services and relatively small number of exclusion criteria for participation. In their work with survivors of Hurricane Ike (2008) in Texas, their population was defined as those who were eligible (e.g., had an internet connection) and consented to the intervention (reach/inclusion). Of 5,536 individuals who were contacted, 43% were not eligible, 4% refused to participate, and 23% of the total number of contacted individuals were invited to treatment. Thus, a major advantage of defined populations interventions that incorporate digital technologies is to increase reach and engagement.
For PTS, within a digital strategy within a defined population that focuses on secondary prevention (e.g., education, well-being) of PTS problems for those exposed to trauma, interventions would be appropriate along with resources and activities to provide family support and education and other services to the population. By viewing the entirety of the population exposed to traumas, it becomes possible to envision a range of services and activities that may work synergistically to enhance outcomes.
Benefits, challenges, and key questions for a systematic digital defined population approach
The systematic digital approach has the potential benefit of increasing the provision of accessible, affordable, and evidence-based intervention resources to populations in need, as is evident in our work with eating disorders and the model of how it might be applied to a PTS population. The model takes advantage of databases and other digital resources to continuously expand and adapt approaches based on reach, engagement, efficacy, cost and other factors. In fact, groups working in a variety of different settings with similar populations might be able to share resources, observations, ideas, and approaches to improve programs and outcomes more quickly. There also are challenges to this approach, and we touch on some of the major ones here.
A top-down approach
The model assumes that some group of individuals is managing the population toward the stated goals, and that the group has authority to modify various aspects of the system as a whole. This assumes an altruism that may not be realistic in many settings where the goal may be to reduce, rather than increase, utilization, and it may also assume a degree of managerial power and decision-making ability that may not be present. In the consumer world, where these models are widely practiced, increased sales are desired outcomes but there is often the assumption that this occurs through satisfied customers. The model we are proposing should have consumer and user input built into all aspects of decision-making and program deployment. In many mental health treatment systems, outcomes, engagement, and other key variables are not routinely monitored so that the impact of policy changes on effectiveness, reach, and engagement of treatment and prevention activities may remain unclear. Until the potential advantages of the system can be demonstrated—better population-wide outcomes and happier consumers—the model is unlikely to be adopted.
Expense
The defined population model assumes that consumers would be provided resources based on interest. It is possible that the more expensive options (e.g., face-to-face therapy) would be preferred and population-wide screening is likely to generate large numbers of individuals who could overwhelm the system, stressing already limited services and increasing costs. An alternative is to use a stepped care model in which less expensive options are provided first (7). However, we believe there is a right to accessible, affordable, evidence-based care and that, as providers and researchers, we need to find ways to deliver these interventions.
Privacy
The model is based on HIPAA protected information. Protecting participant privacy is essential, and any member of the defined population who wishes to opt out should be able to do so easily, with his/her data removed from the system. A number of measures would need to be taken to ensure that privacy rules and compliance with HIPPA are both followed.
Software
Another major issue is the lack of standard software systems that facilitate rapid authoring and revision of interventions based on lessons learned during the monitoring process. One approach is to work with software developers to create management platforms that allow the outcomes optimization team to author and upload new content on an ongoing basis. However, this approach may warrant greater upfront costs than can be allocated, and still requires expenses over time to maintain and improve the technology.
Defined populations and outcomes
As specified throughout, a key factor is defining the population and outcomes. For example, does the group want to focus on reducing symptoms or another potentially relevant outcome, such as improving functioning? In the case of populations of trauma survivors, the primary needs might include not only symptom reduction, but also a focus on empowerment, employment, social support, political activism, or safety. Changes to interventions and the structure of the treatment system might differentially impact different kinds of outcomes.
Additionally, in responding to traumatized populations, as with those affected by eating disorders, we are challenged to think about designing a model that addresses multiple, co-occurring problems, as is often the case for individuals with PTS. Using a systems approach to address this challenge means that defining the population includes: (I) identifying which groups of users are important to target (e.g., individuals with eating disorders, major depression, and generalized anxiety disorder, but not alcohol use disorder), (II) determining which interventions to offer and whether the model needs ordering rules for the timing of intervention delivery, and (III) agreeing on which outcomes will define success.
Management of defined populations intervention systems. The approach advocated for here requires a centrally directed systematic approach to collaborative actions among interacting teams. Data showing a range of outcomes associated with different screening and intervention components must be analyzed. Teams must use those data to make changes in various aspects of intervention delivery. Such changes may require training of providers as well as changes to software content. The effects of these changes on effectiveness, reach, and engagement must then also be assessed, and additional changes made in an iterative process. It is evident that this process is complex, involves significant personnel resources, and requires leadership buy-in and support. It will be necessary to assemble teams with the requisite expertise, and establish procedures by which ongoing processes of data analysis and intervention redesign can be accomplished in the service of treatment improvement. The conditions necessary to assemble and operate such teams seems most likely to be achieved in health care systems that offer a comprehensive set of services that includes screening and multiple mental health treatment options.
In disasters, most helping efforts go into secondary prevention, in which an attempt is made to assist an affected population in ways that prevent development of PTSD and other problems. In part because major disasters often affect large populations, disaster mental health response currently includes multiple aspects of a stepped care approach, including broad assistance for those affected via outreach programs and delivery of Psychological First Aid (PFA) (28), intermediate intensity crisis counseling services for those needing more support (29), and referrals for those requiring mental health treatment. These outreach, PFA, crisis counseling, and tertiary mental health treatments may be provided by a range of agencies contracted to mount a response in an affected area, with response capabilities distributed across a range of helping organizations rather than located within a single organization. Such a distribution means that systems of multi-organization collaboration will need to be established in order to implement systematic digital defined population interventions. It will likely be necessary to designate and structure a leadership team charged with implementing the intervention system and an additional challenge will be to provide a suite of digital interventions across multiple organizational settings.
It is also important to note that there have been many examples of defined population interventions for PTSD, though none to our knowledge have applied a systematic, outcome-oriented approach. For instance, Zatzick et al. (30) used a medical record to identify acutely injured trauma survivors who were then randomized to an intervention or usual care and followed-for 6 months. Zatzick et al. (31) also examined reach and effect sizes to predict population-level benefits for two PTSD prevention approaches. Engel et al. (32) linked screening for PTSD in primary care settings to collaborative care facilitated by a nurse care manager. The VA has been at the forefront of providing training to providers in evidence-based care (33)—expanding the provider pool is a key step in any defined population intervention. Other studies have examined components of a defined intervention model such as the effects of documenting PTSD best practices templates (34), the effectiveness of self-help (35), and many others. However, to our knowledge none have focused on the use of digital technology to enhance reach, engagement, and outcome in a dynamic way.
Technology is important because of its capacity to increase the scope and efficiency of population-based interventions. It can enable rapid, continuous evaluation that includes outcomes data, but also detailed information about process variables. For example, it will be possible to determine when and where in technology-based interventions that dropout occurs. It can greatly increase the reach of services by more easily including low intensity interventions that can be accessed by large numbers of trauma-affected individuals. It enables cost-effective online screening of large numbers of individuals across geographic areas (36). It makes possible more rapid modification of interventions themselves and speeds processes of application of modified interventions by practitioners with less training burden. Technology also enables inclusion of a larger set of intervention types (e.g., blended interventions) and by strengthening delivery of self-help interventions, makes possible more easily delivered and effective stepped care models. The model we are describing is certainly compatible with the RE-AIM (Reach, Effectiveness, Adoption, Implementation and Maintenance model) (37) and other implementation models, but also should make it easier to apply them.
Conclusions
Advantages of the population-based approach outlined here include a simultaneous focus on effectiveness, engagement, and reach; potential for identification and customization of interventions for specific subpopulations; and improved cost-effectiveness of services. The approach also offers potential for more thoughtful selection and testing of alternative treatment improvement strategies, focusing on aspects of engagement, screening methods, alternative interventions, and different combinations of elements of treatment. Overall, the approach is a way of bringing “measurement-based care” to the larger treatment system management enterprise—that is, to enable measurement-based management.
Such an approach also presents significant obstacles. Health care organizations must reorganize aspects of their operations and assemble multidisciplinary teams to accomplish the various components. Top leadership must strongly support the system because it will be necessary to implement a series of practice changes in multiple sections of the organization as ongoing monitoring efforts lead to redesign of interventions. Implementation of the approach is likely to require significant personnel resources, as well as access to novel software systems. To some degree, large health care organizations may already contain many of the personnel resources and functional capabilities required for the defined populations methods suggested here, but they must be identified and brought together in a coordinated system of care. Despite these significant obstacles, we believe that a systematic digital population-based approach to mental health interventions holds promise for establishing significantly more effective treatment delivery systems, and most importantly, creating a more rapid and effective process of treatment improvement in health care systems and other delivery environments.
Acknowledgements
We want to thank Sarah E. Siegel. This work was supported in part by: (R01 MH100455), (T32 HL007456), (T32 HL130357), and (F32 HD089586).
Footnote
Conflicts of Interest: The authors have no conflicts of interest to declare.
Ethical Statement: The engagement data reported in this paper was covered by Stanford University School of Medicine, IRB2826. Other data was obtained from the public domain.
References
- American Psychiatric Association. Diagnostic and Statistical Manual of Mental Disorders, Arlington, 2013:338-54.
- Hudson JI, Hiripi E, Pope HG Jr, et al. The prevalence and correlates of eating disorders in the National Comorbidity Survey Replication. Biol Psychiatry 2007;61:348-58. [Crossref] [PubMed]
- Klump KL, Bulik CM, Kaye WH, et al. Academy for eating disorders position paper: Eating disorders are serious mental illnesses. Int J Eat Disord 2009;42:97-103. [Crossref] [PubMed]
- Taylor CB, Bryson S, Luce KH, et al. Prevention of eating disorders in at-risk college-age women. Arch Gen Psychiatry 2006;63:881-8. [Crossref] [PubMed]
- Taylor CB, Kass AE, Trockel M, et al. Reducing eating disorder onset in a very high risk sample with significant comorbid depression: A Randomized controlled trial. J Consult Clin Psychol 2016;84:402-14. [Crossref] [PubMed]
- Eisenberg D, Nicklett EJ, Roeder K, et al. Eating disorder symptoms among college students: Prevalence, persistence, correlates, and treatment-seeking. J Am Coll Health 2011;59:700-7. [Crossref] [PubMed]
- Wilfley DE, Agras WS, Taylor CB. Reducing the burden of eating disorders: A model for population-based prevention and treatment for university and college campuses. Int J Eat Disord 2013;46:529-32. [Crossref] [PubMed]
- Jacobi C, Fittig E, Bryson SW, et al. Who is really at risk? Identifying risk factors for subthreshold and full syndrome eating disorders in a high-risk sample. Psychol Med 2011;41:1939-49. [Crossref] [PubMed]
- Fitzsimmons-Craft EE, Firebaugh ML, Kass AE, et al. Implementing an Internet-Based Platform for Eating Disorder Screening, Prevention, and Treatment on College Campuses: Preliminary Results from a State-Wide Initiative. Psychol Serv. In Press.
- Kass AE, Balantekin KN, Fitzsimmons-Craft EE, et al. The economic case for digital interventions for eating disorders among United States college students. Int J Eat Disord 2017;50:250-8. [Crossref] [PubMed]
- Muir S, Newell C, Griffiths J, et al. MotivATE: A Pretreatment Web-Based Program to Improve Attendance at UK Outpatient Services Among Adults With Eating Disorders. JMIR Res Protoc 2017;6. [Crossref] [PubMed]
- Karlin BE, Brown GK, Trockel M, et al. National dissemination of cognitive behavioral therapy for depression in the department of veterans affairs health care system: Therapist and patient-level outcomes. J Consult Clin Psychol 2012;80:707-18. [Crossref] [PubMed]
- Muñoz RF. The Efficiency Model of Support and the Creation of Digital Apothecaries. Clin Psychol Sci Pract 2017;24:46-9. [Crossref]
- Beintner I, Jacobi C, Taylor CB. Effects of an internet-based prevention programme for eating disorders in the USA and Germany: A Meta-analytic Review. Eur Eat Disord Rev 2012;20:1-8. [Crossref] [PubMed]
- Andrews G, Basu A, Cuijpers P, et al. Computer therapy for the anxiety and depression disorders is effective, acceptable and practical health care: An updated meta-analysis. J Anxiety Disord 2018;55:70-8. [Crossref] [PubMed]
- Melioli T, Bauer S, Franko DL, et al. Reducing eating disorder symptoms and risk factors using the internet: A meta-analytic review. Int J Eat Disord 2016;49:19-31. [Crossref] [PubMed]
- Turgoose D, Ashwick R, Murphy D. Systematic review of lessons learned from delivering tele-therapy to veterans with post-traumatic stress disorder. J Telemed Telecare 2017:1357633X17730443.
- Sadeh-Sharvit S, Kim JP, Darcy AM, et al. Subgrouping the users of a specialized app for eating disorders. Eat Disord 2018.1-12. [Crossref] [PubMed]
- Prins A, Bovin MJ, Smolenski DJ, et al. The Primary Care PTSD Screen for DSM-5 (PC-PTSD-5): Development and evaluation within a veteran primary care sample. J Gen Intern Med 2016;31:1206-11. [Crossref] [PubMed]
- Knaevelsrud C, Maercker A. Internet-based treatment for PTSD reduces distress and facilitates the development of a strong therapeutic alliance: a randomized controlled clinical trial. BMC Psychiatry 2007;7:13. [Crossref] [PubMed]
- Kuester A, Niemeyer H, Knaevelsrud C. Internet-based interventions for postraumatic stress: A meta-analysis of randomized controlled trials. Clin Psychol Rev 2016;43:1-16. [Crossref] [PubMed]
- Spoont MR, Murdoch M, Hodges J, et al. Treatment receipt by veterans after a PTSD diagnosis in PTSD, mental health, or general medical clinics. Psychiatr Serv 2010;61:58-63. [Crossref] [PubMed]
- Ruzek JI, Karlin BE, Zeiss A. Implementation of evidence-based psychological treatments in the Veterans Health Administration. In: McHugh RK, BarlowDH (eds). Dissemination and Implementation of Evidence-Based Psychological Interventions, New York: Oxford University Press, 2012:78-96.
- Ruzek JI, Follette VM. Cognitive-Behavioral Therapies for Trauma. 2nd edition. New York: Guilford Press, 2006:433-62.
- Koepsell TD, Zatzick DF, Rivara FP. Estimating the population impact of preventive interventions from randomized trials. Am J Prev Med 2011;40:191-8. [Crossref] [PubMed]
- Shalev AY, Ankri YL, Peleg T, et al. Barriers to Receiving Early Care for PTSD: Results From the Jerusalem Trauma Outreach and Prevention Study. Psychiatr Serv 2011;62:765-73. [Crossref] [PubMed]
- Price M, Gros DF, McCauley JL, et al. Nonuse and Dropout Attrition for a Web-Based Mental Health Intervention Delivered in a Post-Disaster Context. Psychiatry 2012;75:267-84. [Crossref] [PubMed]
- Vernberg EM, Steinberg AM, Jacobs AK, et al. Innovations in Disaster Mental Health: Psychological First Aid. Prof Psychol Ref Prac 2008;39:381-8. [Crossref]
- Berkowitz S, Bryan R, Brymer M, et al. Skills for Psychological Recovery: Field Operations Guide. Available online: https://www.ptsd.va.gov/professional/manuals/manual-pdf/SPR_Manual.pdf
- Zatzick D, O'Connor SS, Russo J, et al. Technology-Enhanced Stepped Collaborative Care Targeting Posttraumatic Stress Disorder and Comorbidity After Injury: A Randomized Controlled Trial. J Trauma Stress 2015;28:391-400. [Crossref]
- Zatzick DF, Koepsell T, Rivara FP. Using Target Population Specification, Effect Size, and Reach to Estimate and Compare the Population Impact of Two PTSD Preventive Interventions. Psychiatry 2009;72:346-59. [Crossref] [PubMed]
- Engel CC, Oxman T, Yamamoto C, et al. RESPECT-Mil: Feasibility of a Systems-Level Collaborative Care Approach to Depression and Post-Traumatic Stress Disorder in Military Primary Care. Mil Med 2008;173:935-40. [Crossref] [PubMed]
- Karlin BE, Ruzek JI, Chard KM, et al. Dissemination of evidence-based psychological treatments for posttraumatic stress disorder in the Veterans Health Administration. J Trauma Stress 2010;23:663-73. [Crossref] [PubMed]
- Sripada RK, Bohnert KM, Ganoczy D, et al. Documentation of Evidence-Based Psychotherapy and Care Quality for PTSD in the Department of Veterans Affairs. Adm Policy Ment Health 2018;45:353-61. [Crossref] [PubMed]
- Kuhn E, Kanuri N, Hoffman JE, et al. A randomized controlled trial of a smartphone app for posttraumatic stress disorder symptoms. J Consult Clin Psychol 2017;85:267-73. [Crossref] [PubMed]
- Leykin Y, Muñoz RF, Contreras O. Are consumers of internet health information “cyberchondriacs”? characteristics of 24,965 users of a depression screening site. Depress Anxiety 2012;29:71-7. [Crossref] [PubMed]
- Glasgow RE, Vogt TM, Boles SM. Evaluating the public health impact of health promotion interventions: the RE-AIM framework. Am J Public Health 1999;89:1322-7. [Crossref]
Cite this article as: Taylor CB, Ruzek JI, Fitzsimmons-Craft EE, Graham AK, Balantekin KN. A systematic digital approach to implementation and dissemination of eating disorders interventions to large populations identified through online screening: implications for post-traumatic stress. mHealth 2018;4:25.