Effect of mHealth on modifying behavioural risk-factors of non-communicable diseases in an adult, rural population in Delhi, India
Introduction
With non-communicable diseases (NCDs) accounting for an estimated 68% of all deaths and about half of the premature deaths in 2012, it is evident that NCDs are reaching epidemic proportions (1). This has led to a “dual burden” in low and middle-income (LAMI) countries like India which are still battling with high prevalence of communicable diseases. NCDs accounted for 53% of the deaths and 44% of DALYs in India in 2004, and it is predicted that they will be responsible for almost three-quarters of all deaths in India by 2030 (2,3). The incorporation of a target (Target 3.4) specially dedicated to NCDs within the goal 3 of the newly adopted Sustainable Development Goals indicates the importance the world now accords to prevention and control of NCDs (4).
Four modifiable behavioural risk factors are associated with increased risk of NCDs. These are tobacco use, physical inactivity, unhealthy diet and harmful use of alcohol. Primary prevention of NCDs is directed towards modifying these risk factors. This paper focuses on two of the above risk factors, namely, physical inactivity and unhealthy diet. These risk factors are widely prevalent in India. An NCD Risk Factor survey conducted in several states of India by the Integrated Disease Surveillance Project (IDSP) in 2009 found that the prevalence of low physical activity (less than 600 MET-min per week) ranged from 42% in the state of Madhya Pradesh to 81% in the state of Maharashtra. Unhealthy diet was even more widely prevalent with the proportion of population consuming less than the recommended five servings of fruits and vegetables per day ranged from 76% in the state of Maharashtra to 99% in the state of Tamil Nadu (5).
Behaviour change communication (BCC) is an important strategy for modification of behavioural or lifestyle-associated risk factors of NCDs. Mobile phone technology is viewed as a promising communication channel that offers the potential to improve healthcare delivery and promote behaviour change among vulnerable populations. A major advantage of mHealth interventions is the fact that they can be delivered to many individuals in a cost effective manner and in a relatively shorter time.
There is a paucity of studies demonstrating the effect of mHealth in lifestyle modifications for NCDs, especially in LAMI countries and evidence for effectiveness of these interventions comes from reviews which have not included such countries (6,7). However, a systematic review conducted to investigate the effectiveness of mHealth interventions to promote physical activity and healthy diets reviewed 15 studies conducted in LAMI countries across the globe, concluded that the majority of the studies demonstrated that mHealth interventions were effective in promoting physical activity and healthy diets (8).
The current study was undertaken which the objective of assessing the effect of mHealth intervention in bringing about changes in all four behavioural risk factors of NCDs in a rural population in Delhi, India. However, in this paper, we will discuss the effect of mHealth intervention on two behavioural risk factors, namely, unhealthy diet and physical inactivity.
Methods
Study design
We conducted a community-based, “before and after” intervention study with control over a period of one year from January 2016 to December 2016. The study site was Barwala Village, located in the north-west district of Delhi, India. The village is served by the Rural Health Training Centre (RHTC) under Maulana Azad Medical College, New Delhi, India. We obtained the approval of the Institutional Ethics Committee of the college prior to commencement of the study. The study was registered with the Clinical Trials Registry of India with the registration number CTRI/2017/03/008264.
Participants
We recruited the participants using a two-staged sampling method. First, systematic random sampling was done at household level using the household list available at RHTC as a sampling frame. Next, we selected one resident fulfilling the inclusion criteria from each household by simple random sampling using lottery method (by numbered chits) to avoid clustering effect. If the person selected by the lottery method was absent, we requested the family members to ask the person to be present on the next holiday and a visit was made on that day. If the person could not be contacted after 3 successive visits, that household was not considered and another was chosen.
We included adults aged 18 to 64 years, who had been residing in the village for at least 6 months, had access to mobile phones with Short Message Service (SMS) facility in Hindi (local language) and could read the messages. Individuals with impaired sight, hearing, inability to talk and those with severe mental retardation were excluded from the study. We also excluded those individuals who could not be contacted after 3 successive visits and those women who were pregnant at the time of the study.
Participants were enrolled in the study after obtaining written and informed consent.
Sample size, allocation and blinding
With the assumption of a 17% prevalence of tobacco smoking in Delhi (least prevalence out of the four behavioural risk factors, to obtain the maximum sample size), at 95% confidence with 80% power, 144 participants per group were needed for a 10% reduction in risk factors to be detected (9). Assuming an attrition rate of 20%, 174 participants were needed for each group. We rounded up the sample size and aimed to recruit 200 participants in each group.
The participants were allocated to intervention and control groups in the ratio of 1:1. Barwala village is divided into two parts—north and south—by a 30 m wide road traversing through it. The intervention and control groups were selected from the southern and the northern part respectively. Thus, a geographically stratified allocation procedure was applied. This was done to avoid contamination due to interaction between the two groups.
Due to the nature of the intervention, it was not possible to blind the investigator and the participants to the allocation. However, the laboratory personnel were blinded to the allocation.
Procedure
We conducted the study in 3 phases—a 2 months long Pre-Intervention Phase followed by an 8 months long Intervention Phase, which was in turn followed by a 2 months long Post-Intervention Phase. In the Pre-Intervention Phase, we collected baseline data from both groups using a pre-tested, semi-structured interview schedule based on the WHO STEPS approach for NCD risk factor surveillance, which has been modified and validated in the Indian context under the Integrated Disease Surveillance Project (IDSP). We collected personal and socio-demographic data and detailed information about behavioural risk factors of NCDs (improper diet, physical inactivity, tobacco and alcohol use). This was followed by physical and anthropometric examination whereby height, weight, BMI, waist and hip circumference and blood pressure was measured. Finally, we collected blood samples for measurement of biochemical parameters-fasting blood glucose and fasting lipid profile.
In the Intervention Phase, which lasted 8 months, we contacted the subjects in the intervention group on their mobile phone via telephone call once a month. Each telephone call lasted for about 20 minutes during which we reiterated the importance of modifying behavioural risk factors of NCDs, addressed queries and provided positive reinforcement. The intervention package also included weekly text messages via SMS. The text messages included short but catchy slogans and rhymes on the importance of modification of behavioural risk factors. Each message had an average of 25–30 words. The schedule of the messages was prepared addressing each behavioural NCD risk factor and messages were sent every week in rotation. At a time, only one risk factor was addressed. No such mHealth intervention package was given to the control group.
In the Post-Intervention Phase, we visited the participants and carried out the interview, and physical examination again using the same schedule and collected blood samples for repeat measurement of biochemical parameters. The Post-Intervention Phase was carried out during similar climatic conditions as the baseline, to eliminate effect of seasonal differences in behaviour pattern.
Outcome and other measures
We expressed physical activity in terms of Metabolic Equivalent-minutes (MET-min) and weekly activity level of 600 or more MET-min was considered to be adequate, in accordance with WHO recommendations (10). Diet was assessed in terms of number of servings of fruits or vegetables in a day. Standard definition of ‘serving’ was used as per WHO STEPS approach and a daily consumption of 5 or more servings of fruits or vegetables was considered to be adequate (10).
Our primary outcome was the percentage change in behavioural risk factors at the end of the study in the intervention group as compared to control group.ie change in the proportion of subjects engaged in low physical activity (less than 600 MET-min per week) and proportion consuming inadequate diet (less than 5 servings of fruits or vegetables per day).
Our secondary outcomes were changes in physiological risk factors (BMI, Blood Pressure-systolic and diastolic) and biochemical risk factors (Fasting blood glucose, Total cholesterol, LDL and HDL cholesterol). These variables were expressed quantitatively and difference in means was assessed.
Statistical methods
Data analysis was done per protocol. The data was entered and cleaned in Ms Excel and analysed using SPSS version 22. Descriptive tables were made expressing qualitative variables in terms of percentages and quantitative variables in terms of mean and standard deviation. We checked for normality of quantitative data using Shapiro-Wilk test and examining the histogram and frequency polygon and found that the quantitative risk factor data was all non-normally distributed. Difference in baseline characteristics between control and intervention groups were tested using chi-square test for qualitative and Mann-Whitney U test for quantitative risk factor data. Wilcoxon sign-rank test was used to determine significance of difference between pre-intervention and post-intervention values in both control and intervention groups. A P value of less than 0.05 was taken to be statistically significant.
Results
Figure 1 depicts the flow of participants. We approached 408 individuals fulfilling the inclusion criteria and asked them to enrol for the study. Of these, 1.5% (n=6) were unavailable after 3 consecutive visits and 0.5% (n=2) declined to participate. The remaining 400 subjects were allocated to control group (n=200) and intervention group (n=200) in a non-random way based upon the geographic location of the household in the village. Among the control group, 4% (n=8) were lost to follow-up. Two women became pregnant during the study period and 3 women got married and moved out of the study area, hence becoming ineligible for the study. Three participants declined to continue the study. Among the intervention group, 5% (n=10) were lost to follow up. Three women became pregnant during the study period and 2 women got married and moved out of the study area, hence becoming ineligible for the study. Three participants declined to continue the study and 3 were unavailable at follow-up visits. Hence, of the 400 enrolled participants, 382 completed the study, thus the completion rate was 95.5%.
Table 1 shows the socio-demographic characteristics of the participants in control and intervention groups. Chi square test was used to analyse differences in proportions of the characteristics of the two groups and no significant differences were found. Thus, the two groups were similar in terms of socio-demographic characteristics such as age, sex, education, occupation and marital status at baseline.
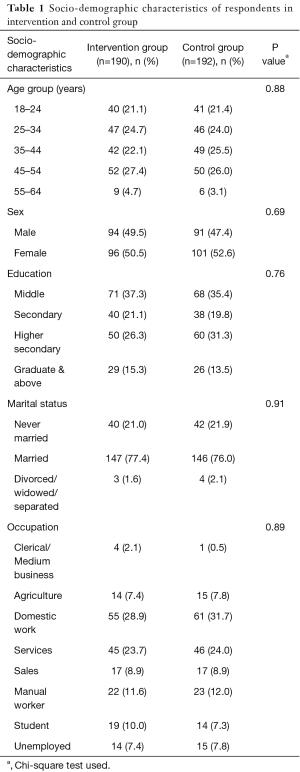
Full table
Table 2 shows the baseline data regarding behavioural, physiological and clinical risk factors of NCDs in control and intervention groups. As the data was non-normal, Mann-Whitney U test was used to analyse the difference in the baseline levels of risk factors between control and intervention group. No significant difference was found in any of the risk factors and the two groups had similar risk factor profiles at baseline.
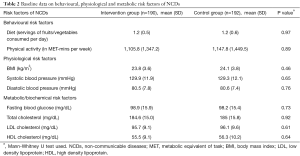
Full table
In categorical terms, inadequate diet was found to be universally prevalent in both groups at baseline. All the participants in both groups consumed less than 5 servings of fruits and vegetables per day. Insufficient physical activity of less than 600 MET-min per week was seen in 41.6% (n=79) of the intervention group and 39.6% (n=76) of the control group at baseline.
Figure 2 depicts the primary outcome i.e., percentage change in behavioural risk factors at the end of the study in the intervention group as compared to control group. At the end of the intervention period, the percentage of participants consuming inadequate diet reduced from 100% (n=190) to 94.7% (n=180) in the intervention group. In the control group, this change was from 100% (n=192) at baseline to 97.9% (n=188) at the end of the study. Insufficient physical activity reduced from 41.6% (n=79) to 35.3% (n=67) in the intervention group at the end of the study while no change was observed in terms of percentage in the control group.
Table 3 shows the mean change in risk factors (behavioural, physiological and biochemical) after mHealth intervention in control and intervention groups. Wilcoxon sign-rank test was used to determine the significance of the difference of the paired, non-normally distributed data. Mean number of servings of fruits and vegetables per day increased significantly in both intervention group and control group. In the intervention group, the mean number of daily servings of fruits and vegetables increased from 1.2 to 2.8 (Z=‒11.961, P=0.000) while in control group, it increased from 1.2 to 2.2 (Z=‒12.041, P=0.000). Physical activity was found to increase from an average of 1105.8 to 1166.5 MET-min per week in the intervention group which was found to be statistically significant (Z=‒4.430, P=0.000). This amounts to an increase of approximately 15 minutes of moderate activity per week. No statistically significant change in physical activity was found in the control group.
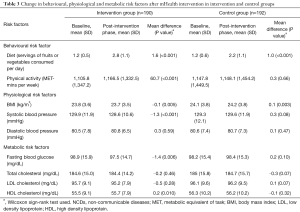
Full table
Significant reductions were also seen in the physiological risk factors in the intervention group as compared to control group. Mean BMI in intervention group reduced from 23.8 to 23.7 at the end of the intervention period (Z=‒2.834, P=0.005). In the control group, there was a significant increase in the mean BMI from 24.1 to 24.2 kg/m2 at the end of the intervention period (Z=‒2.934, P=0.003). Mean systolic blood pressure reduced from 129.9 to 128.6 mmHg in the intervention group which was found to be statistically significant (Z=‒3.824, P=0.000). There was a marginal increase in mean systolic blood pressure in the control group from 129.3 to 129.6 mmHg which was not found to be significant (Z=‒1.761, P=0.08). No significant difference was seen mean diastolic blood pressure in either group.
Among the biochemical risk factors, statistically significant changes were seen in fasting blood glucose and HDL cholesterol level in the intervention group. Mean fasting blood glucose reduced significantly from 98.9 to 97.5 mg/dL in the intervention group (Z=‒2.730, P=0.006) and mean HDL cholesterol increased from 55.5 to 55.7 mg/dL (Z=‒2.579, P=0.01) in the same group. No statistically significant changes in these risk factors were seen in the control group. Changes in total cholesterol and LDL cholesterol were found to be insignificant in both intervention and control groups.
Discussion
Our study showed that using mHealth interventions such as phone call and SMS for BCC can be effective in reducing inadequate diet and insufficient physical activity- two of the behavioural risk factors of NCDs. Other studies, conducted in developed countries, have also demonstrated usefulness of SMS-based interventions in increasing physical activity and improving diet. A parallel-group randomised control trial (RCT) conducted in the United Kingdom found that SMS-based intervention significantly improved the physical activity level of the intervention group as compared to control group. The study concluded that text messages aid the recall of, and could enhance interventions that target, plans and goals related to increased physical activity (11). A study from Sweden evaluated the effectiveness of a weight loss program delivered via SMS. The program addressed regulation of diet as well physical activity and reported that by 12 months the experimental group had lost significantly more weight than the control group (12). A systematic review published in 2016 reviewed 15 studies evaluating mHealth and eHealth interventions for promotion of healthy diets and physical activity in LAMI countries. The results of this systematic review suggested that eHealth & mHealth interventions could be effective in improving physical activity and diet quality in LAMI countries. Overall, the review showed that 50% of the eHealth & mHealth interventions were effective in increasing physical activity, and 70% of the identified interventions were effective in improving diet quality (8).
It is not possible to assess whether the phone calls or the text messages contributed more towards the change observed in this study. However, the feature of phone call in the mHealth package provided a gateway for two-way communication. The text messages provided continuous input of health messages and the phone calls served to reiterate those messages, follow-up on progress and provide motivation and positive reinforcement to the subjects.
The strength of our study is that we evaluated behavioural, physiological as well as biochemical risk factors for NCDs. Since behavioural risk factors are self-reported and based on recall, they are prone to bias. Anthropometric measurement, blood pressure measurement and measurement of fasting blood glucose and lipid profile in our study provides objectivity and reduces bias. Further, there was a geographic separation between control and intervention group (by a 30 m wide road running through the village) to prevent contamination. Participants living further apart are less likely to interact and pass on the health promotion messages to each other. Finally, our study was conducted at community level in a randomly selected population. This enhances the external validity of our study.
Our study had some limitations. Firstly, allocation to control and intervention group was non-randomised and this may introduce selection bias. Secondly, blinding of the participants and investigator was also not possible in this study design and this may be a source of bias. Thirdly, behavioural risk factors were self-reported and hence prone to recall and self-desirability bias. Finally, since the sample size of the study was calculated based on the primary outcome (change in behavioural risk factors), it might be underpowered to detect the changes in the physiological and the biochemical risk factors which have lesser prevalence in the community.
Most of the studies on mHealth in LAMI countries have focussed on management of NCDs and adherence to treatment. A few studies evaluating its effectiveness on health promotion have been conducted in diabetic or hypertensive patients (13). Our study has demonstrated the usefulness of mHealth for health promotion and lifestyle modification at community level in a LAMI country. However, there is a need for more such studies, preferably large-scale RCTs to be conducted to generate good quality evidence.
This study was carried out to evaluate the effectiveness of BCC delivered via mobile phones. Although inter-personal communication is the most effective tool for BCC, it is expensive to deliver in terms of time, money and man-power. An attractive feature of mHealth that makes it a promising alternative is its cost effectiveness. With the growing burden of NCDs in the community, such cost effective and innovative measures will be needed that can easily reach the masses.
Acknowledgements
The authors would like to thank the administrative and technical staff of Maharishi Valmiki Hospital, Pooth Khurd, Delhi, India for providing laboratory support for this study.
Footnote
Conflicts of Interest: The authors have no conflicts of interest to declare.
Ethical Statement: We obtained the approval of the Institutional Ethics Committee of the college prior to commencement of the study. The study was registered with the Clinical Trials Registry of India with the registration number CTRI/2017/03/008264 and written informed consent was obtained from all the participants.
References
- World Health Organisation. Global status report on noncommunicable diseases 2014. Available online: http://www.who.int/nmh/publications/ncd-status-report-2014/en/
- Srinath Reddy K, Shah B, Varghese C, et al. Responding to the threat of chronic diseases in India. Lancet 2005;366:1744-9. [Crossref] [PubMed]
- Patel V, Chatterji S, Chrisholm D, et al. Chronic diseases and injuries in India Lancet 2011;377:413-28.
- Sachs JD. From millennium development goals to sustainable development goals. Lancet 2012;379:2206-11. [Crossref] [PubMed]
- IDSP Non-communicable disease risk factors survey, Phase-I States of India, 2007-08. Available online: http://www.icmr.nic.in/final/IDSP-NCD%20Reports/Phase-1%20States%20of%20India.pdf
- Vandelanotte C, Müller AM, Short CE, et al. Past, present and future or e- & mHealth research to improve physical activity and dietary behaviors. J Nutr Educ Behav 2016;48:219-28.e1. [Crossref] [PubMed]
- Burke LE, Ma J, Azar KM, et al. Current science on consumer use of mobile health for cardiovascular disease prevention: a scientific statement from the American Heart Association. Circulation 2015;132:1157-213. [Crossref] [PubMed]
- Müller AM, Alley S, Schoeppe S, et al. The effectiveness of e-& mHealth interventions to promote physical activity and healthy diets in developing countries: A systematic review. Int J Behav Nutr Phys Act 2016;13:109. [Crossref] [PubMed]
- Garg A, Anand T, Sharma U, et al. Prevalence of risk factors for chronic non-communicable diseases using WHO steps approach in an adult population in Delhi. J Family Med Prim Care 2014;3:112-8. [Crossref] [PubMed]
- World Health Organization. The WHO STEPwise approach to surveillance of non-communicable diseases (STEPS). Geneva: WHO; 2003.
- Prestwich A, Perugini M, Hurling R. Can implementation intentions and text messages promote brisk walking? A randomized trial. Health Psychol 2010;29:40-9. [Crossref] [PubMed]
- Haapala I, Barengo NC, Biggs S, et al. Weight loss by mobile phone: a 1-year effectiveness study. Public Health Nutr 2009;12:2382-91. [Crossref] [PubMed]
- Stephani V, Opoku D, Quentin W. A systematic review of randomized controlled trials of mHealth interventions against non-communicable diseases in developing countries. BMC Public Health 2016;16:572. [Crossref] [PubMed]
Cite this article as: Sharma M, Banerjee B, Ingle GK, Garg S. Effect of mHealth on modifying behavioural risk-factors of non-communicable diseases in an adult, rural population in Delhi, India. mHealth 2017;3:42.