Evaluating clinicians’ user experience and acceptability of LearnTB, a smartphone application for tuberculosis in India
Introduction
Tuberculosis (TB) is the highest infectious disease killer worldwide (1). It is an airborne infectious disease, caused by Mycobacterium tuberculosis, leading to 10.4 million cases worldwide and causing 1.8 million deaths (1,2). The End TB strategy by the World Health Organisation (WHO) aims to reduce TB deaths, as well as incidence rate by 95% and 90% respectively. Additionally, the number of families facing high costs due to treatment are aimed to be reduced to 0% (3). The current rate of decline of TB incidence is 1.5% annually; however this rate must increase to 4–5% per year to achieve the End TB strategy goals.
India, the highest TB-burden country, accounts for 27% (2.8 million) of the world’s 10.4 million TB cases (4). India accounts for 29% of the 1.8 million TB deaths globally, and also for 16% of the estimated 480,000 new cases of multidrug-resistant TB (MDR-TB). Approximately 40% of the Indian population is estimated to be latently infected with TB (5). Research continues to highlight the constant neglect and mismanagement of TB patients in India, contributing to the high burden of disease (6,7). Additional challenges include low financial investments, HIV-coinfection, poverty, malnutrition, and an unregulated private health sector that is not engaged in TB control efforts (8).
The health care system in India is divided into two different sectors, public and private. The public sector (i.e., government services), encourages the Directly Observed Treatment Short-course (DOTS) strategy, and is implemented by the Revised National Tuberculosis Programme (RNTCP). This strategy provides basic TB diagnosis and treatment to all patients in India, and encourages patients to take proper medication and adhere to treatment regimens. Furthermore, it offers free TB care, including diagnosis and treatment (6). Despite the availability of free TB care in the public sector, a majority of patients in India prefer to seek care in the private sector.
The private sector, largely unregulated by the Indian government, has grown immensely over the past 20 years and now manages 82% of the general patient population as well as 50% of India’s TB patients (9). The National Health Survey-3 reported 70% of households in urban areas and 63% of households in rural areas visit the private sector as their primary source of healthcare (10). Patients prefer private clinics as they are of closer proximity to vulnerable populations residing in remote areas, and offer a reduced wait time (7). Approximately 6 million (75%) of clinicians practice in the private sector, and 0.31% implement RNTCP regulations (11). The Indian private sector is highly heterogenous, with qualified formal doctors, as well as unqualified/informal providers, and practitioners of alternative systems of healthcare.
Studies show complicated, long care seeking pathways and extensive diagnostic delays (12), widespread empirical management (13), and poor adherence to established standards of care in the private sector (14). Often this is due to poor knowledge about TB, especially among informal care providers, inaccessibility to proper training, and inadequate supervision and re-training. The new era of digital technologies in health may have the potential to provide a solution, especially in India, a country where mobile telephony has exploded in the past decade.
Mobile health (mHealth), is defined as the delivery of health care services and information through mobile phones and other wireless telecommunication technologies (15). It has the potential to play a vital role in delivering health care to remote populations lacking human resources (15). mHealth technologies are not limited to mobile phones, they also include: personal digital assistants (PDA), portable media players (MP3), video-game consoles (Nintendo), smartphones, and ultra-portable computers (tablets) (16). They are often consumer centered and consumer driven, and aim to provide support to health care providers through education, patient management and support in diagnosis (17,18). India is the second highest mobile phone consumer base in the world, due to its low-cost handsets and affordable calling plans (19). mHealth and electronic health (eHealth) strategies have been increasing steadily in India with the biggest example being NIKSHAY (http://nikshay.gov.in), the TB case-based notification system. Implemented in 2012, it has increased India’s TB case notification by 34%, affecting total TB estimates worldwide (4). The success of this ehealth strategy demonstrates the potential of other ehealth and mHealth interventions to be accepted in the Indian healthcare environment.
Previous mHealth interventions
User experience studies are important influencers to mHealth interventions as they can potentially increase uptake and acceptability of the intervention (20,21). Despite their high importance, few scientific studies have reported evaluation of user experience regarding mHealth interventions. In the literature, user experience is often quantified through the System Usability Scale (SUS). This scale has been used in over 600 studies and has proved to be highly reliable (20). Previous studies have used the SUS to evaluate user experience of an mHealth smartphone application once the final version of the application was ready for diffusion (21,22). However, user experience studies are best suitable for applications which are presented in their beta version (prototype version) to further improve the application prior to dissemination to the public (20-22).
Previous research has evaluated the acceptability of mHealth interventions amongst a specific population. Studies have concentrated their efforts in improving their interventions prior to evaluating the acceptability (23,24) and others investigated the acceptability of mHealth interventions demonstrating positive results (25-28). Each of these studies evaluated interventions other than smartphone applications, such as short messaging service (SMS), telecommunication interfaces as well as ehealth interventions emphasizing protocol compliance and treatment adherence respectively. Authors reported lack of knowledge to be the biggest limitation regarding the mHealth intervention. This impeded proper assessment of the application, as participants did not feel comfortable using it (25-28). Based on a systematic review conducted by Gagnon et al., most mHealth studies evaluating acceptability of smartphone applications have been conducted amongst health care professionals such as nurses, pharmacists and health workers (18). Furthermore, studies have been largely concentrated in the western hemisphere: Canada, United States of America and Europe (18). Amongst studies evaluating factors influencing mHealth adoption, the Technology Acceptance Model (TAM) has been used with different groups of health care professionals, including doctors and nurses, university faculty members, university students, nursing home residents and inpatient diagnostic groups (29-33).
A systematic review by Iribarren et al. identified 1,332 health applications amongst three major application stores, of which 24 were TB related (34). Most applications targeting clinicians were not formally tested. There were numerous problems with the applications such as inability to open the app, and lack of up to date information (34). This systematic review underscored the need for acceptability studies for mobile applications targeting TB.
In summary, the knowledge gap regarding mHealth interventions in India is evident through the limitations present in the aforementioned studies. Primarily, most studies were conducted in the western hemisphere or in countries other than low and middle income countries (LMIC), where delivery of healthcare is needed the most. Secondly, very few studies evaluated mHealth interventions targeting tuberculosis. Thirdly, there were few studies conducted on doctors and clinicians, the population with the greatest ability to influence healthcare. Finally, most studies reported their biggest limitation to be inability to understand the mHealth intervention. To our knowledge, a scientific study evaluating the user experience and acceptability of an mHealth intervention targeting TB amongst health care clinicians in India has not yet been documented. Our study aims to overcome the barriers listed by previous studies by focusing on: (A) India, the highest TB burden country; (B) tuberculosis, a disease known to be a public health threat in India; (C) clinicians, who are influential players in TB diagnosis and management; and (D) conducting a two-part study evaluating user experience and then acceptability of a prototype version of an mHealth smartphone application.
Objectives
Our study aimed to understand the user experience and acceptability of a newly-developed smartphone application, LearnTB, amongst private sector academic clinicians in India. Specifically, we aimed to (I) assess user experience with the aim of improving the LearnTB mobile application and (II) identify, based on the TAM, the factors influencing adoption of LearnTB by the private sector academic clinicians.
For our study purposes, the term “acceptability” is defined as the intention to use (IU), “clinician” refers to resident and academic clinicians practicing at Kasturba Hospital Manipal and “mobile application” refers to smartphone applications only, specifically the prototype version of the LearnTB application.
Theoretical framework
Part 1
Based on previous studies, the most common, free and easy to use framework for usability studies is the SUS, a Likert scale, developed by J. Brooke in 1996 (20) (Figure 1). The SUS was developed by presenting a series of 50 questions to respondents; only statements soliciting extreme agreement or extreme disagreement were selected to be included in the final SUS scale (20). Brooke et al. emphasize the inaccuracy of ambiguous questions in determining a participants’ attitude towards a specific technology. For this reason, the SUS, is largely used in usability studies to measure user experience, the utility which includes the efficacy and satisfaction with which users accomplish specific tasks (18). Usability studies assess participants’ immediate reaction to the use of a specific technology, prior to any discussion with the researcher (20). The SUS comprises of 10 statements evaluating the user experience. However, our study used a modified version of the SUS comprising of 9 statements preceding the acceptability assessment.
Part 2
The proposed theoretical framework for the second part of our study is the widely used TAM. The TAM, proposed by Davis in 1989, is considered to be influenced by the Theory of Reasoned Action (18). This framework is used to evaluate the acceptability of an information technology by users in an organisational setting. The TAM suggests that the acceptance [behavioral intention to use (IU)] of a new information technology is affected directly and indirectly by a user’s attitude towards use, and two internal individual beliefs: perceived usefulness (PU) and perceived ease of use (PEU). PU is defined to be “the user’s subjective probability that using a specific application system will increase his or her job performance within an organizational context” (18), whereas PEU is defined as “the degree to which the prospective user expects the target system to be free of effort” (18). Behavioural IU measures the likelihood of a person employing the intervention (18). One of the advantages of the TAM is that it can be generalized amongst all populations, however one of its disadvantages is that it does not consider environmental factors such as institutional or social factors which can influence technology acceptance. Based on TAM studies, PU has a direct effect on IU whereas PEU has an indirect effect on IU but a direct effect on PU. For our study purposes, we used an adapted version of TAM, comprising of PEU and PU as the independent variables and IU as the sole dependant variable (Figure 2).
Study hypothesis
Based on the theoretical framework presented above, the study hypothesis are listed below. They have been validated through previous TAM studies as well (35).
- The usability of the LearnTB application can influence the user experience of the private sector academic clinicians
- The TAM constructs will explain a significant proportion of clinician IU the LearnTB application:
- PU is positively correlated with IU;
- PEU is positively correlated with IU;
- PEU is positively correlated with PU.
Methods
LEARNTB mobile application
The smartphone application of interest for this study, LearnTB (Figure 3), was inspired by the contents of the Let’s Talk TB, a free, online handbook aimed for general practitioners (GP) in India (available http://www.letstalktb.org). The handbook was specifically created for the Indian medical context, and many chapters have been co-authored by Indian physicians. This application, a collaboration between Mayo Clinic Center for Tuberculosis (Rochester, USA) and McGill International Tuberculosis Centre (Montreal, Canada), aims to educate Indian clinicians regarding the definition, diagnosis, treatment, management and counselling practices available for TB. The primary version of the application was created by Global Innovative Services Inc. (Maryland, USA). This study tested the pilot website version of the application.
LearnTB presents numerous different sections regarding TB management, such as diagnosis of TB, treatment of TB, management of childhood TB, latent TB infection, extra pulmonary TB, common pitfalls of TB management and counselling practices in India (Figure 4). Each section was based on International Standards of TB Care, as well as WHO guidelines and Standards for TB Care in India (36,37). When clicking on a specific section (i.e., Childhood tuberculosis), participants were presented with subsections involving suspecting, diagnosing and treating TB for each respective case (Figure 5). Dosage tables, and specific names of drugs were also included to facilitate understanding. A subsection regarding chest X-rays for TB presented figures of different types of TB lesions to help visualize the extent and manifestations of TB disease (Figure 6). The section focusing on the TB HIV co-infection also included frequently-asked questions to help clinicians asses specific/special cases. In addition to reference material, most sections presented short quizzes to assure proper comprehension and to emphasize educational learning from the application.
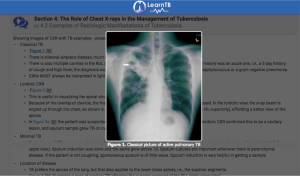
Study setting and design
The study was conducted at Kasturba Hospital Manipal, a tertiary teaching hospital affiliated with Manipal University, located in the south Indian state of Karnataka. It has approximately 1,500 practicing doctors including senior faculty members, junior faculty members, lecturers, postgraduate students and interns, and over 1,500 medical students (38,39). Both parts of the study were conducted successively, following a cross sectional study design respectively.
Participant recruitment
For the first part of our study, five participants were recruited in February 2017 and for the second part of our study, 101 participants were contacted between February 2017 and March 2017. A sample size of 5 was chosen for the first part of the study, as previous studies have indicated that most errors (mean 85.5%) in technologies are identified by five participants (40,41). Furthermore, user experience studies aiming to assess prominent problems in prototype technologies, such as LearnTB, often do not require large sample sizes (41). A minimum sample size of 100 participants was sufficient for the second part of the study, as the calculation was based on the theoretical outcome of a standard deviation (SD) of 1.07, evaluated by previous studies (29,32). Theoretical values for type 1 error (α) of 5% and a margin of error of 3% were used. The lowest non-response rate of 40%, was reported in a previous study using the TAM amongst clinicians, thus this was taken into consideration during the sample size calculation (29). A minimum sample size of 100 participants was adequate to achieve all theoretical values of SD, type 1 error and the margin of error used in the sample size calculation.
Both parts of the study recruited participants through a convenience non-probabilistic sampling approach. Additionally, for the second part of the study, snowball sampling was also used. Participants were asked to respond to the questionnaire and share the objectives of the study with their peers. Furthermore, participants were contacted by faculty members of Kasturba Hospital Manipal and organized a meeting time with the lead researcher, TP. All participants were required to have completed their Bachelor of Medicine, Bachelor of Surgery (MBBS) degree, and be working at Kasturba Hospital Manipal. All participants were asked to sign a consent form indicating their understanding of the research study and its objectives. All questionnaires were in English as well as confidential and anonymous.
Data collection
Part 1
Data was collected through a 9-statement paper-based self-administered questionnaire involving SUS for the first part of our study. One statement, “the application was easy to use”, was omitted as it would be repeated in the second part of our study. The content validity of the questionnaire was justified, as the statements were from the original version of a SUS questionnaire which is highly reliable [Cronbach alpha (α) =0.91] (42). Participants were given the application in its website format, on an iPad and asked to complete two tasks. Upon completion of the tasks, they were asked to immediately rate 9 statements on a 5-point SUS scale ranging from “strongly disagree” to “strongly agree”. Participants were asked to address their questions and concerns after completing the questionnaire, to best capture the user experience.
Part 2
Data was collected via an adapted version of the TAM questionnaire (29,30). Participants having participated in the first part were not asked to participate in the second part of the study. A total of 101 medical employees of Kasturba Hospital, Manipal were contacted and 100 (99%) responded. Participants were given English paper-based questionnaires during individual sessions and group sessions with the lead researcher, TP. The self-administered questionnaires, comprised of 15 items, were answered following a brief presentation of the LearnTB application (Table 1). Each individual session lasted approximately 10 minutes, and each group session lasted 20 minutes. Participants were asked to rate each item through a 7-point Likert scale ranging from “totally disagree” to “totally agree”. The mean scores of each individual item were computed and the mean of means of each variable (PU, PEU, and IU) were used to perform statistical analysis. Sociodemographic variables such as age, sex, position, title, years of clinical experience, previous use of mobile application, and willingness to use mobile applications, were also collected in both parts of the study. Years of clinical experience were defined as years after graduating from the MBBS degree (an undergraduate medical degree in India).
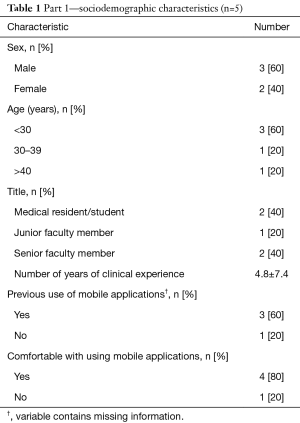
Full table
Statistical analysis
Part 1
The statistical analysis was completed through Excel 2016 for the first part of the study. Theoretically, the 10 statement SUS responses lead to values ranging from 0–40, which are then multiplied by a factor of 2.5 resulting to values ranging from 0–100, thus facilitating comprehension (20). Our study included 9 statements, thus decreasing our range of response values to 0–36. To permit values ranging from 0-100, our response values were multiplied by a factor of 2.78. One was subtracted from responses corresponding to odd numbered questions, and those corresponding to even number questions were subtracted by 5, as per the theoretical formula of SUS (20). A theoretical value of 68 was assumed as the threshold for above average results (43).
Part 2
The statistical analysis software (SAS 9.4) was used to compute descriptive and inferential statistics of the data. The Cronbach alpha (α) values of the three theoretical values, PEU, PU and IU were calculated to assure reliability and validity of the TAM questionnaire (44,45). We computed descriptive statistics such as mean, and SD of the theoretical variables; PU, PEU, and IU. Correlations between the three theoretical values, age, sex, and title were evaluated using the Pearson’s coefficient of correlation (r). All variables followed a normal distribution (Gaussian distribution), thus multiple linear regression analysis was used to determine a predictive model for IU (46). A predictive model was evaluated for all theoretical variables (PU, PEU, IU), as well as age, sex and title. The 95% confidence intervals (CIs) were calculated, and a statistical significance of 0.05 was used. Ad hoc analyses such as path analysis and logistic regression were also performed. Path analysis helped confirm the direct and indirect relationships between theoretical variables, PU, PEU and IU (46). Logistic regression analysis was used to target specific items which influence IU, to help guide future studies and public health recommendations regarding mHealth strategies. The dependent variable, IU was dichotomized by the mean; participants with an score less than or equal to 6 were qualified as “low to moderate” IU, and those greater than 6 were considered as “high” IU.
Ethics approval
This study was approved by the ethics review board of Université Laval (ref: 2016-165), in Québec City, Canada as well as the MUEC, Manipal University Ethics Committee (ref: MUEC/020/2016-17), in Manipal, India.
Results
Part 1
The sociodemographic characteristics of the participants in the user experience study are shown in Table 1. Amongst the 5 participants of this part of the study, 3 were male and 2 were females. Three participants were aged below 30 years. Two participants were medical residents/interns, two were senior faculty members and one was a junior faculty member. Three participants had previously used mobile applications for clinical use, and four felt comfortable using mobile applications. The mean years of clinical experience was 4.8 with a SD of 7.4. The average SUS score was 94.4 with a SD of 2.7. The individual SUS scores and SD for each characteristic are shown in Figure 7.
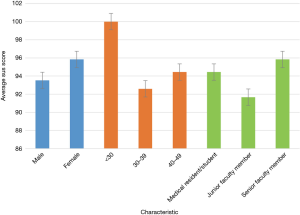
Part 2
Characteristics of the population
The sociodemographic characteristics of the population for the second part of the study are presented in Table 2. Of the 101 people who were asked to participate in the study, 100 responded to the paper-based questionnaires (99%). There were 60 males (60%) and 40 females (40%) who responded to the questionnaires. A great majority of participants (94%) were under the age of 30 years, 4 (4%) were between 30–39, 1 (1%) between 40–49 and 1 (1%) over the age of 50. The average years of clinical experience was 2.88 with a SD of 4.20, and 82 participants (82.8%) were medical residents/interns. Fifteen participants were junior faculty members and 3 were senior faculty members. Overall, 86% of participants had previously used mobile applications for clinical use, and 92 (95.8%) felt comfortable using mobile applications.
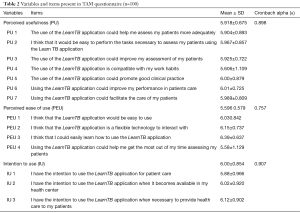
Full table
Internal reliability
The content validity of the adapted TAM questionnaire was justified as the Cronbach alpha (α) values were higher than 0.70 justifying the reliability of TAM (Table 3) (45). Originally the Cronbach alpha (α) was low for PEU. An acceptable value of the Cronbach alpha (α=0.75) was obtained by eliminating the item PEU_5 “The use of the LearnTB application could interfere with the usual follow up of my patients”.
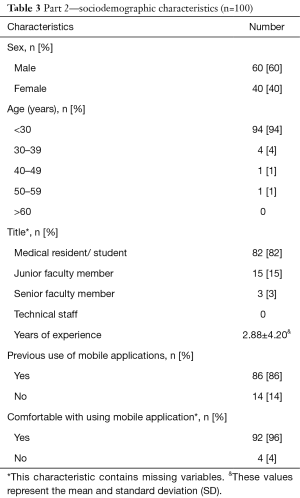
Full table
Descriptive statistics and multiple linear regression analysis
The descriptive statistics of the TAM constructs are presented in Table 3, including the mean and SD of each of the theoretical variables. The Pearson’s correlation (r) between PEU, PU, IU, age, sex, and title are presented in Table 4. There was a statistically significant correlation between PU and IU, as well as PEU and PU. Other variables, such as sex, age, title did not present statistically significant correlations with IU.
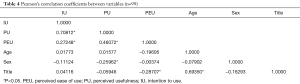
Full table
The multiple linear regression analysis presented in Table 5 reports a significant variance (R2) of 50.5%. PEU is not a significant predictor of IU, however, PU has a large significant effect on IU. Upon adding the other variables—sex, age, and title—to the equation, the model did not change. However, the variance (R2) increased to 52.6%. Path analysis, aiming to understand direct and indirect relationships between the theoretical variables confirmed that PU has a significant direct relationship to IU (0.94; P<0.0001), whereas PEU has a significant indirect relationship to IU (0.51; P<0.0001).
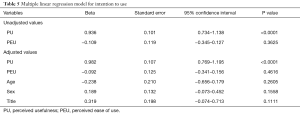
Full table
In order to identify potential targets for future interventions, we conducted a logistic regression analysis of each of the items within PU and PEU, on IU. The items most strongly predictive of IU are: item PEU 4 “Using the LearnTB application could help me get the most out of my time assessing my patients” with a significant odds ratio (OR) =2.393 (1.346–4.253; P<0.003); item PU 4 “The use of the LearnTB application is compatible with my work habits” [OR =3.201 (1.041–9.840); P=0.0042]; and item PU 5 “The use of the LearnTB application could promote good clinical practice” [OR =5.243 (1.354–20.297); P=0.0164].
Discussion
Due to the vast knowledge gap discussed previously, our study aimed to overcome many barriers addressed in previous studies. This study was conducted to understand the user experience and acceptability of a smartphone application amongst private sector academic clinicians in India. Based on the results presented in part 1, the overall user experience was high (94.4). This is known to be a very good user experience score, as the threshold is 68 (22). Academic clinicians were excited to see an application for TB, one of the clinicians mentioned “A similar app with various other medical conditions would be very helpful”, thus emphasizing the high user experience score. Contrary to other studies (21,22), there were very few barriers noted during data collection regarding incapability of using the application, or difficulty completing the tasks. Furthermore, the participants responded unanimously (strongly disagree) to the two questions addressing unfamiliarity; “I think I would need support of a technical person to be able to use the LearnTB application” and “I need to learn a lot of things before I could get going with the LearnTB application”. This is a positive note for the LearnTB application as studies have shown that the rate at which a new technology is accepted often increases when a user can learn how to use the technology on their own (44). Nevertheless, upon informal discussions with the participants, some provided recommendations such as including “education videos” and “adding a checklist for diagnostic and treatment techniques”. As this study was done on the website version (prototype version) of the application, each of these recommendations can be taken into consideration for improving the application.
The second part of the study aimed to understand the acceptability of the LearnTB application amongst the private sector academic clinicians. In concordance with previous studies, our study reported a significant correlation between PEU and PU, confirming our third hypothesis (47,48). This is supported by the original TAM, as PEU has a direct influence on PU, and an indirect influence on IU (49). Our results also showed that PU of the LearnTB application is strongly correlated to IU, whereas PEU is not. The strong correlation between PU and IU supports our first hypothesis and has been avidly shown in previous studies having used TAM or a derivation of TAM as their theoretical framework (48-51). This is in concordance with the original TAM model, where PU has a direct influence on IU (49). This was also presented through the path analysis performed in our study, where PU had a significant direct relationship to IU. Furthermore, the high regression coefficient for the linear relationship between PU and IU is also explained by the strong correlation between the two variables. Although participants’ PU of the application was quite high, their main concerns were regarding data requirements and internet connectivity while using the application. Many clinicians addressed the connectivity issues present in large parts of India, and the lack of wireless internet connection thus limiting use of certain applications. Such factors must be taken into consideration when creating future versions of the LearnTB application as well as other mHealth studies.
Unlike certain previous studies, such as Cajita et al. and Zhang et al. (48,51,52), our results did not report a correlation between PEU and IU, which rejects our second hypothesis. Nevertheless, this is consistent with previous findings as it is possible that increased experience with mobile phones reduces the impact of PEU on IU (50,53). Chen et al. hypothesize that the PU of an application may be of greater importance to a medical practitioner than the PEU (50), however, Omar et al. hypothesize the opposite (52). The lower impact of PEU on IU in our results can be supported through the increased use of mobile technologies in India. As mentioned previously, there are 877 million mobile subscriptions (28), thus ease of use of the mobile technologies may not be as important to clinicians, as they are accustomed to them. The fact that the item “The use of the LearnTB application is compatible with my work habits” was significantly associated with IU further emphasizes this point as clinicians who find that using the LearnTB application is compatible with their work habits are 3.2 times more likely to have a high IU it. Factors such as sex, age and title did not change the linear regression model significantly, contrary to previous hypotheses (51). This solicits further investigation as a “non-uniform” population could help further analyze their effect on the relationship between PEU, PU and IU.
Our study presents numerous strengths. Primarily, to our knowledge, it is the first to evaluate the user experience and acceptability of a smartphone mHealth intervention for TB amongst clinicians in India. It is also amongst the few studies which test a pilot version of an mHealth application prior to creating the final version. This is one of the biggest strengths of our study. Secondly, the questionnaires used in both parts of the study were valid and reliable. Both questionnaires had previously been tested and had Cronbach alpha’s greater than 0.7 (29,32,54). Thirdly our study achieved a response rate of 100% and 99% for part 1 and part 2 respectively, increasing our study power as well as study credibility. Finally our study assessed TB which is a prominent issue in India, once again increasing the credibility of our study.
Limitations
Despite the numerous strengths of our study, the results must be interpreted while taking certain limitations into consideration. Primarily, our study has a degree of selection bias as participants were sampled using a non-probabilistic sampling method, specifically convenience and snow-ball sampling. Due to time constraints, this was the most feasible for our study, however future studies should adapt a probabilistic approach which could further increase generalizability of the results. Additionally, future studies should be done on a heterogeneous population of numerous hospitals in India.
Our study was conducted on a homogeneous population consisting only of academic clinicians at Kasturba Hospital Manipal, a top ranked private medical school, and therefore does not reflect the heterogeneity of private sector providers in India. Secondly, participants could have been subject to social desirability bias, as the researcher administering the questionnaires, TP, had been a part of the application development process. Thirdly, the study design was cross sectional, thus permitting analysis of only one time point. The study was designed in two parts to allow for improvement of the application prior to the acceptability analysis, however due to time constraints both parts were done simultaneously at one time point. Future studies should use longitudinal designs to; (I) allow time between the user experience study and the acceptability study and (II) observe acceptance rates of users, perhaps using the diffusion of innovation theory as a theoretical framework (44). Finally, the SUS scale used in our study comprised of only 9 statements as compared to the theoretical model of 10 statements. The mathematical procedure using a factor of 2.78 to analyse SUS scores has not been previously validated, to our knowledge, thus a validated model would have been favorable.
Conclusions
To our understanding, our study was the first to formally evaluate user experience and acceptability of a mHealth intervention for tuberculosis amongst private sector clinicians in India. Our results indicated that the IU an application is largely dependent on the PU. The overall user experience of the LearnTB application was high as well. These results show positive IU of the LearnTB application and encourage further development for public use. As the application used in this study was presented as a preliminary website version, the final application will need further testing amongst a larger, heterogeneous, population of Indian private and public sector clinicians. Although, the website version was well received by the academic clinicians, many concerns regarding accessibility, network problems and data usage were raised. It is important to address these issues prior to dissemination of the final LearnTB application. Developers have indicated that the fully developed version of the LearnTB application will not require internet connection. Despite being the second highest mobile phone consumer base in the world, India presents numerous network problems and data usage concerns. Future mHealth applications should take such factors into consideration to allow for better uptake and usage. Our exploratory study has provided sufficient information to allow eventual dissemination of an improved version of the LearnTB application with the aim of helping proper diagnosis and treatment of TB, eventually decreasing the high burden of TB in India.
Acknowledgements
This study is part of a master of science thesis project of TP. We would like to thank all participants of the study and all staff members of Kasturba Hospital who helped in participant recruitment.
This study was funded by the TMA Pai Endowment Fund.
Footnote
Conflicts of Interest: The authors have no conflicts of interest to declare.
References
- World Health Organization. Global tuberculosis report 2016. 2016. Available online: http://www.who.int/tb/publications/global_report/gtbr2016_executive_summary.pdf
- World Health Organization. Private sector contributions and their effect on physician emigration in the developing world 2013. Available online: http://www.who.int/bulletin/volumes/91/3/12-110791/en/
- World Health Organization. The End TB Strategy. 2015. Available online: http://www.who.int/tb/End_TB_brochure.pdf
- World Health Organization. Global Tuberculosis Report 2015. Available online: http://www.who.int/tb/publications/global_report/en/
- Kanabus A. Information about tuberculosis 2016. Available online: http://www.tbfacts.org/
- Bhargava A, Pinto L, Pai M. Mismanagement of tuberculosis in India: causes, consequences, and the way forward. Hypothesis 2011;9:e7. [Crossref]
- Satyanarayana S, Subbaraman R, Shete P, et al. Quality of tuberculosis care in India: a systematic review. Int J Tuberc Lung Dis 2015;19:751-63. [Crossref] [PubMed]
- Sandhu GK. Tuberculosis: current situation, challenges and overview of its control programs in India. J Glob Infect Dis 2011;3:143-50. [Crossref] [PubMed]
- Sengupta A, Nundy S. The private health sector in India: Is burgeoning, but at the cost of public health care. BMJ 2005;331:1157-8. [Crossref] [PubMed]
- Salve S, Sheikh K, Porter JD. Private Practitioners' Perspectives on Their Involvement With the Tuberculosis Control Programme in a Southern Indian State. Int J Health Policy Manag 2016;5:631-642. [Crossref] [PubMed]
- WHO Report on Global Tuberculosis Control: Epidemiology, Strategy, Financing. Geneva: World Health Organization; 2013.
- Sreeramareddy CT, Qin ZZ, Satyanarayana S, et al. Delays in diagnosis and treatment of pulmonary tuberculosis in India: a systematic review. Int J Tuberc Lung Dis 2014;18:255-66. [Crossref] [PubMed]
- McDowell A, Pai M. Treatment as diagnosis and diagnosis as treatment: empirical management of presumptive tuberculosis in India. Int J Tuberc Lung Dis 2016;20:536-43. [Crossref] [PubMed]
- Das J, Kwan A, Daniels B, et al. Use of standardised patients to assess quality of tuberculosis care: a pilot, cross-sectional study. Lancet Infect Dis 2015;15:1305-13. [Crossref] [PubMed]
- Ajzen I. The theory of planned behaviour: reactions and reflections. Psychol Health 2011;26:1113-27. [Crossref] [PubMed]
- Free C, Phillips G, Felix L, et al. The effectiveness of M-health technologies for improving health and health services: a systematic review protocol. BMC Res Notes 2010;3:250. [Crossref] [PubMed]
- Akter S, Ray P. mHealth - an Ultimate Platform to Serve the Unserved. Yearb Med Inform 2010.94-100. [PubMed]
- Gagnon MP, Ngangue P, Payne-Gagnon J, et al. m-Health adoption by healthcare professionals: a systematic review. J Am Med Inform Assoc 2016;23:212-20. [Crossref] [PubMed]
- Elangovan R, Arulchelvan S. A Study on the Role of Mobile Phone Communication in Tuberculosis DOTS Treatment. Indian J Community Med 2013;38:229-33. [Crossref] [PubMed]
- Brooke J. SUS-A quick and dirty usability scale. Usability Evaluation in Industry 1996;189:4-7.
- Uddin AA, Morita PP, Tallevi K, et al. Development of a Wearable Cardiac Monitoring System for Behavioral Neurocardiac Training: A Usability Study. JMIR Mhealth Uhealth 2016;4:e45. [Crossref] [PubMed]
- Gunter R, Fernandes-Taylor S, Mahnke A, et al. Evaluating Patient Usability of an Image-Based Mobile Health Platform for Postoperative Wound Monitoring. JMIR Mhealth Uhealth 2016;4:e113. [Crossref] [PubMed]
- Ginsburg AS, Delarosa J, Brunette W, et al. A customized m-Health system for improving tuberculosis treatment adherence and follow-up in south India. Health and Technology. 2014;4:1-10. [Crossref]
- Ginsburg AS, Delarosa J, Brunette W, et al. mPneumonia: Development of an Innovative mHealth Application for Diagnosing and Treating Childhood Pneumonia and Other Childhood Illnesses in Low-Resource Settings. PLoS One 2015;10:e0139625. [Crossref] [PubMed]
- Modi D, Gopalan R, Shah S, et al. Development and formative evaluation of an innovative mHealth intervention for improving coverage of community-based maternal, newborn and child health services in rural areas of India. Glob Health Action 2015;8:26769. [Crossref]
- Gautham M, Iyengar MS, Johnson CW. Mobile phone-based clinical guidance for rural health providers in India. Health Informatics J 2015;21:253-66. [Crossref] [PubMed]
- Florez-Arango JF, Iyengar MS, Dunn K, et al. Performance factors of mobile rich media job aids for community health workers. J Am Med Inform Assoc 2011;18:131-7. [Crossref] [PubMed]
- DeSouza SI, Rashmi MR, Vasanthi AP, et al. Mobile phones: the next step towards healthcare delivery in rural India? PLoS One 2014;9:e104895. [Crossref] [PubMed]
- Gagnon MP, Orruño E, Asua J, et al. Using a modified technology acceptance model to evaluate healthcare professionals' adoption of a new telemonitoring system. Telemed J E Health 2012;18:54-9. [Crossref] [PubMed]
- Alharbi S, Drew S. Using the technology acceptance model in understanding academics’ behavioural intention to use learning management systems. International Journal of Advanced Computer Science and Applications 2014;5:143-55. [Crossref]
- Huang F, Chang P, Hou IC, et al. Use of a mobile device by nursing home residents for long-term care comprehensive geriatric self-assessment: a feasibility study. Comput Inform Nurs 2015;33:28-36. [Crossref] [PubMed]
- Park SY. An Analysis of the Technology Acceptance Model in Understanding University Students' Behavioral Intention to Use e-Learning. Educational Technology & Society 2009;12:150-62.
- Hennemann S, Beutel ME, Zwerenz R. Drivers and Barriers to Acceptance of Web-Based Aftercare of Patients in Inpatient Routine Care: A Cross-Sectional Survey. J Med Internet Res 2016;18:e337. [Crossref] [PubMed]
- Iribarren SJ, Schnall R, Stone PW, et al. Smartphone Applications to Support Tuberculosis Prevention and Treatment: Review and Evaluation. JMIR Mhealth Uhealth 2016;4:e25. [Crossref] [PubMed]
- Wilson EV, Lankton NK. Modeling patients' acceptance of provider-delivered e-health. Journal of the American Medical Informatics Association 2004;11:241-8. [Crossref] [PubMed]
- Hopewell PC, Pai M, Maher D, et al. International standards for tuberculosis care. Lancet Infect Dis 2006;6:710-25. [Crossref] [PubMed]
- Mohan A. International standards of tuberculosis care. Natl Med J India 2006;19:301-5. [PubMed]
- Manipal KMC. About KMC 2017. Available online: https://manipal.edu/kmc-manipal/hospital/services.html
- Manipal University. History 2016. Available online: http://manipal.edu/mu/about-us/history.html
- Nielsen J, Landauer TK. editors. A mathematical model of the finding of usability problems. Proceedings of the INTERACT'93 and CHI'93 conference on Human factors in computing systems; 1993: ACM.
- Six JM, Macefield R. How to Determine the Right Number of Participants for Usability Studies 2016. Available online: http://www.uxmatters.com/mt/archives/2016/01/how-to-determine-the-right-number-of-participants-for-usability-studies.php
- Garcia A. UX Research, Standardized Usability Questionnaires 2013. Available online: http://chaione.com/ux-research-standardizing-usability-questionnaires/
- Sauro J. Measuring usability with the system usability scale 2011. Available online: https://measuringu.com/sus/
- Rogers EM. Diffusion of innovations. New York: Simon and Schuster; 2010.
- Tavakol M, Dennick R. Making sense of Cronbach's alpha. Int J Med Educ 2011;2:53-55. [Crossref] [PubMed]
- Land KC. Principles of path analysis. Sociological Methodology 1969;1:3-37. [Crossref]
- Kwak ES, Chang H. Medical Representatives' Intention to Use Information Technology in Pharmaceutical Marketing. Healthc Inform Res 2016;22:342-350. [Crossref] [PubMed]
- Zhang X, Han X, Dang Y, et al. User acceptance of mobile health services from users' perspectives: The role of self-efficacy and response-efficacy in technology acceptance. Inform Health Soc Care 2017;42:194-206. [Crossref] [PubMed]
- Davis FD. Perceived usefulness, perceived ease of use, and user acceptance of information technology. MIS Quarterly 1989;13:319-40. [Crossref]
- Chen J, Park Y, Putzer GJ. An examination of the components that increase acceptance of smartphones among healthcare professionals. e-Journal of Health Informatics 2010;5:16.
- Cajita MI, Hodgson NA, Budhathoki C, et al. Intention to Use mHealth in Older Adults With Heart Failure. J Cardiovasc Nurs 2017. [Epub ahead of print]. [Crossref] [PubMed]
- Omar A, Ellenius J, Lindemalm S. Evaluation of Electronic Prescribing Decision Support System at a Tertiary Care Pediatric Hospital: The User Acceptance Perspective. Stud Health Technol Inform 2017;234:256-261. [PubMed]
- Hsu HM, Chang IC, Lai TW. Physicians’ perspectives of adopting computer-assisted navigation in orthopedic surgery. Int J Med Inform 2016;94:207-14. [Crossref] [PubMed]
- Bangor A, Kortum PT, Miller JT. An emeirical evaluation of the system usability scale. Intl Journal of Human–Computer Interaction 2008;24:574-94. [Crossref]
Cite this article as: Pande T, Saravu K, Temesgen Z, Seyoum A, Rai S, Rao R, Mahadev D, Pai M, Gagnon MP. Evaluating clinicians’ user experience and acceptability of LearnTB, a smartphone application for tuberculosis in India. mHealth 2017;3:30.