Community-based field implementation scenarios of a short message service reporting tool for lymphatic filariasis case estimates in Africa and Asia
IntroductionOther Section
The Global Programme to Eliminate Lymphatic Filariasis (GPELF) has two main components: to interrupt the transmission of lymphatic filariasis (LF) through mass drug administration (MDA), and to manage morbidity and prevent disability (MMDP) for those individuals suffering from the clinical manifestations of the disease (1). As the GPELF moves towards the elimination goal of 2020, many countries are scaling-up surveillance and morbidity management activities to satisfy the WHO dossier components required for certification of LF elimination as a public health problem. For the MMDP aspects of certification, country programmes must report information on the following: (I) the number of LF patients in implementation units (IU), usually defined as a district (2); (II) the number of facilities providing the recommended package of care to IUs with known patients; and (III) assessments of the readiness and quality of care in these facilities (3). In 2014, only 24 out of 73 of endemic countries (33%) reported having active MMDP components in their LF programmes and only 30 endemic countries (41%) reported data on the number of lymphoedema patients (4). As there are limited resources available, there is a pressing need for a rapid and adaptable tool for obtaining patient estimates so that country programmes can appropriately forecast, plan and deliver a basic package of care to those suffering from the disabling and debilitating clinical manifestations of LF in an affordable manner.
There are a number of different methods available for obtaining patient estimates in endemic IUs; these include house-to-house censuses, health facility surveys, cluster surveys, health worker and community informants as well as MDA and Transmission Assessment Survey (TAS) registrations (3,5). The recently developed mHealth ‘MeasureSMS-Morbidity’ tool offers a rapid and scalable data reporting method which can be utilised to report data collected in any of the aforementioned methods and can be adapted to meet country-specific requirements (6). The MeasureSMS-Morbidity tool was developed at the Liverpool School of Tropical Medicine specifically to improve and enhance national filarial disease patient estimates (6). Initially designed for use in cross-sectional population surveys, the tool could also be used for ongoing reporting by health facilities.
MeasureSMS-Morbidity enables trained health workers to use their own mobile phones to send patient data in the form of a simple short message service (SMS) to a smartphone, which is locally situated and acts as a server. Provided the smartphone is connected to a mobile phone network, health workers will receive an automated response to the data received; once the smartphone is connected to the internet via WIFI or a local network connection, this patient information is then relayed to a central cloud-based server (6). By empowering health workers, this tool gives country programmes a rapid method of collecting and collating information on LF patients including their location, age, gender, clinical condition (hydrocele, lymphoedema, or both), severity of the condition (mild, moderate, or severe) and episodes of acute attacks. First piloted in Malawi and Ghana (7), this tool has now been refined and scaled-up for programmatic use in various settings to search and report cases in endemic areas across Africa and Asia, covering a population of over 22 million people. The aim of this communication is to use our experiences in implementing community-based patient searching in Africa and Asia to present implementation scenarios for the tool that could be utilised by national LF programmes in order to scale-up searching and reporting of LF clinical cases.
MethodsOther Section
Checklist of factors
Several key development factors have been used when considering the design and planning of the MeasureSMS-Morbidity survey in any given setting. The five key factors (feasibility of the tool, endemicity, population demography, health system structure, and integration with other diseases) are summarised with corresponding questions in Table 1.
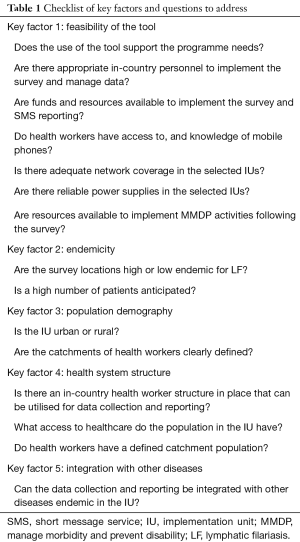
Full table
Feasibility of the tool
LF programme acceptability
In-country support and logistic capacity are critical factors in deciding the feasibility and usage of an mHealth tool such as MeasureSMS-Morbidity for estimating patient numbers. At the country level, MeasureSMS-Morbidity must support the programme needs and a technical capacity must be present in-country to manage the survey for it to be both scalable and cost-effective. The availability of funds and resources is also an influencing factor in this decision due to the number of personnel that will be required to take part in the surveys.
Related questions to determine the acceptability of the tool are:
- Does the use of the tool support the programme needs?
- Are there appropriate in-country personnel to implement the survey and manage data?
- Are funds and resources available to implement the survey and SMS reporting?
Community access and ownership
As a community-led mobile phone technology tool, health worker access to and knowledge of mobile phones is essential for implementation of MeasureSMS-Morbidity. Mobile phone ownership at the health worker level both country-wide and in specific IUs should be anticipated. For the survey data to be successfully reported, the availability of network coverage in survey areas is an important factor in deciding how the reporting system will be structured. For instance, is it feasible to send SMS from all survey locations, or is a central reporting system needed?
Like network coverage, access to a reliable power supply is crucial in deciding the feasibility of the tool as data reporters must be able to charge their mobile phones in order to send the SMS. In areas where prolonged power cuts lasting several days are common, an mHealth tool may not be the most appropriate method of obtaining patient estimates in a pre-defined time period.
Related questions are:
- Do health workers have access to, and knowledge of mobile phones?
- Is there adequate network coverage in the selected IUs?
- Are there reliable power supplies in the selected IUs?
Endemicity
In order to utilise available resources effectively and equitably within the LF programme, the IUs with a higher level of historic endemicity should be prioritised, so that patient care can be targeted effectively within these areas. Data may be collected and reported in two ways, either a one-tier system in which the health worker both collects and reports the data by SMS, or a two-tier system in which a community health worker is the data collector, and collects the data on paper forms; the paper forms are delivered to a health worker (supervisor) who then sends the data via SMS. If a high number of patients are anticipated in an IU, a one-tier reporting system may be the most appropriate method due to the high number of SMS that will be required to be sent; meaning one health worker will act as both the data collector and data reporter. A two-tier system where a centrally located health worker collates the data from multiple data collectors to send the SMS for all patients in a defined area may result in a high work load for the data reporters.
In areas where the prevalence of clinical disease is likely to be low, a house-to-house survey will not be cost-effective (cost per case identified). If MDA has not been implemented in these low endemic IUs, then patient registration during a campaign is not possible. Therefore, a less intensive method is appropriate in these areas where fewer patients are anticipated, and it may be possible to conduct a survey using a team of data reporters who visit the IU and gather information through a combination of health facility data, health worker informants and community informants. In low endemic IUs where other clinical diseases are being mapped, it may be possible to integrate the surveys so that a house-to-house census can be utilised, thus reducing the risk of under-reporting.
The key questions relating to endemicity are:
- Are the survey locations high or low endemic?
- Is a high number of patients anticipated?
Population demography
The scale and density of the population in an IU will impact the nature of the survey to obtain patient estimates. In short, irrespective of endemicity, it will determine the number of data collectors and/or reporters, and the length of time required to survey the population.
A large, urban population may result in health facilities having sizeable catchment populations that require a vast number of man-hours to cover the catchment population. By engaging data collectors as an additional tier of the reporting system, it will be possible to reach the whole population within a shorter time-frame. In rural settings, where populations are more dispersed, but catchments of health workers are more defined, the survey time will be dependent on the length of time required to physically reach the population.
Key questions related to population demography are:
- Is the IU urban or rural?
- Is the catchment of health workers clearly defined?
Health system structure
Human resources
In planning the use of the MeasureSMS-Morbidity tool it is important to consider if there is an in-country healthcare structure in place that can be utilised for data collecting and reporting, such as a community health worker (CHW) network, and if so, how can this be harnessed. In both one- and two-tier reporting systems, it is important to identify the most appropriate data collector and data reporter to ensure case identification and reporting is accurate.
The key question when considering which personnel should be defined as the data collectors and reporters is:
- Is there an in-country health worker structure in place that can be utilised for data collection and reporting?
Community access to healthcare
The population demographics may also influence the survey design based on the relationships of the health workers with the population. In urban settings, the population will have access to a greater number of healthcare providers, and may access a range of healthcare facilities, in comparison to rural settings, where a limited number of healthcare facilities are available to the population (8). Hence, in rural areas, people are more likely to have a close relationship with the health workers in their local area due to repeated access. This may influence the selection of the health workers who will take part in the survey as it is important to select the health workers that will have the greatest knowledge of patients’ conditions to act as the data collector. Additionally, where health workers have defined catchment areas, these should be utilised to harness the existing relationships between health workers and the catchment population, as well as the pre-existing knowledge of conditions with said population. Where health workers do not have a defined catchment, or catchments overlap, catchments should be assigned based on population size and the timeframe for the survey to ensure the entire population is covered and that there is no duplication of reporting of cases.
The key questions when considering the healthcare system structure in the IU are:
- What access to healthcare do the population in the IU have?
- Do health workers have a defined catchment population?
Integration with other diseases
Co-endemicity
Integrated mapping of clinical disease can be cost-effective and allow for efficient use of resources. Appropriate examples include LF and leprosy co-endemic areas and; LF and podoconiosis co-endemic areas as exemplified in the integrated disease mapping of LF and podoconiosis in Ethiopia (9). In co-endemic areas, it allows the disease to be more precisely identified. This is particularly important for diseases in which the same clinical symptoms arise; for example, lymphoedema as a clinical manifestation for both LF and podoconiosis patients.
The key question is:
- Can the data collection and reporting be integrated with other diseases endemic in the IU?
Field implementation
The checklist has been utilised as a programmatic tool to develop LF clinical case estimates in a total of 17 IUs in five LF endemic countries, to survey a total of 22 million people (Table 2). The methods of implementation from these IUs will be reviewed.
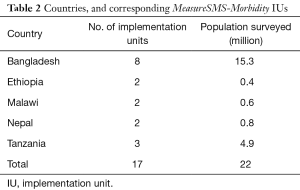
Full table
ResultsOther Section
Implementation scenarios
Four implementation scenarios were identified and the suitable approaches for using the MeasureSMS-Morbidity tool; this being based on experiences of programmatic implementation in 17 IUs in Bangladesh, Ethiopia, Malawi, Nepal and Tanzania. Scenarios 1–3 use a house-to-house census data collection method in endemic IUs, and scenario four is implemented in low endemic IUs using active case finding to locate patients. Within the four scenarios, data collection and reporting may be conducted using either one- or two-tier reporting.
Scenario 1: high endemic, rural, one-tier reporting
In rural, endemic IUs with small, sparsely distributed populations in which a high number of patients are expected to be reported, a one-tier system can be implemented (Figure 1). This scenario was implemented in selected IUs in Malawi and Ethiopia. In such a system, one person acts as both the ‘data collector’ and ‘data reporter’. This system can be used in the absence of an established, tiered CHW system, whereby the health workers at the facility level both collect and report the data.
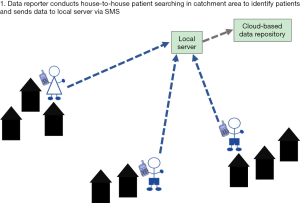
A one-tier system can also be implemented in IUs where CHWs have access to mobile phones with adequate network and power supplies and thus are able to collect and report data by SMS. A one-tier system will improve efficiency of data reporting as the CHWs will not need to report to a central location with patient information.
Scenario 2: high endemic, rural, two tier reporting
In rural, endemic areas where there is an appropriate hierarchical health worker structure in place, a two-tier method of reporting can be implemented. Additionally, in some IUs, it may become apparent when planning, designing and piloting the survey that there are issues with limited mobile phone and SMS use for the CHWs, limited literacy or that there are network issues or power issues that limit the ability of the data collectors to send the data from the field. Such scenarios require two cadres of health workers to be involved in the patient searching; those who ‘identify’ patients in the communities (data collectors) and those who use SMS to ‘report’ the identified patients (data reporters), resulting in a two-tier reporting system (Figure 2). This scenario was selected for implementation in Nepal, where an existing tiered network of health workers exists.
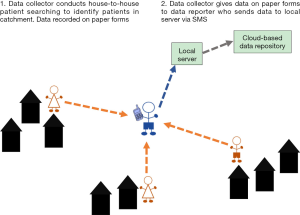
A two-tier system can be employed where a health worker who is centrally located and has consistent access to power and network coverage is more appropriate to report the data by SMS. If, however, high numbers of patients are identified, in a two-tier system with a centrally located data reporter, they would have a large workload sending the SMS for all patients. This should be considered and accounted for when planning the survey, for example, increased regularity of reporting of cases by the CHW data collectors to the data reporter will reduce the number of SMS that need to be sent each day by the data reporters during the survey. Alternatively, increasing the number of centrally located data reporters will reduce the workload of each reporter.
Scenario 3: high endemic, urban, two tier reporting
In endemic urban IUs with large populations in which a high number of LF patients are expected to be identified, there is a need for a two-tier reporting system due to the high number of data collectors that are required to cover the large population (Figure 3). This scenario was selected and implemented in Tanzania (10). This enables the population to be mapped in a reasonable timescale, with a reasonable workload distributed between the data collectors and data reporters, and reduced training expenses due to a smaller number of health workers requiring training in reporting.
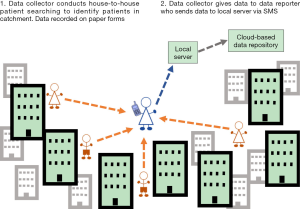
If there is not an established system of CHWs, then selected health facility workers within the IU can be trained as data reporters and report cases collected by other health workers from the health facility. Training a selected number of health workers to act as data reporters and oversee the work of the data collectors reduces training time and costs, while ensuring the survey remains effective. It is appropriate to use a two-tier reporting system in urban areas to increase the reach of the survey in an efficient way.
Scenario 4: low endemic, urban and rural, active case finding and one tier reporting
In low endemic areas where few patients are anticipated, there is a need to make the patient searching both more targeted, and more cost effective. Use of a smaller team of data reporters who conduct ‘active case finding’ using health workers and community members as key informants to identify patients in the IU, is the most appropriate model (Figure 4). This model can be implemented in both urban and rural settings, and was selected and implemented in low endemic IUs in Bangladesh.
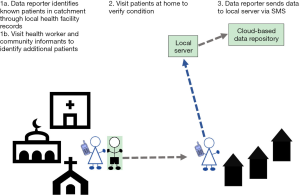
DiscussionOther Section
Following the pilot of MeasureSMS-Morbidity in Malawi and Ghana (7), the use of a checklist of key factors and questions enabled planning and design of the most appropriate scenarios of programmatic implementation of patient searching and reporting using MeasureSMS-Morbidity in 17 IUs in five countries. Based on experiences of utilising the checklist to design and implement the four scenarios, a stepwise framework has been developed using the first four key factors (Figure 5). The framework can be used to determine the most appropriate method of implementation in other countries or IUs, and highlights the adaptability of the tool.
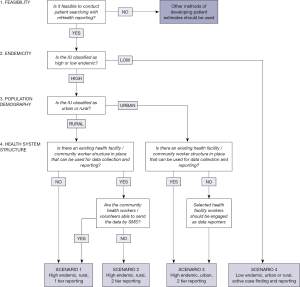
Integration with other disease conditions, the fifth factor, may not impact on the implementation scenario as data on other conditions within the survey population can be recorded at the same time as LF clinical conditions and only data for LF sent in SMS by the data reporters. When the survey is integrated, the type of information that will be collected as well as the decisions that will be informed by the survey data should be considered. For example, in collecting data on lymphoedema cases in LF-podoconiosis co-endemic areas if the aim is to determine the prevalence of clinical disease for morbidity management activities then lymphoedema only needs to be recorded and the CHWs are appropriate data collectors in any implementation scenario. However, if the cause of the lymphoedema is also of interest then a clinical officer may be required to make a differential diagnosis of the lymphoedema and provide appropriate treatment for infection. If mapping of cases of clinical disease due to LF is integrated with leprosy, the complexity of diagnosing leprosy means that CHWs would be able to report suspect cases that would require follow up from a trained clinical officer, or a clinical officer would need to be the data collector; if the latter is the selected model of implementation, then the survey costs may increase. In cases where the data collectors and implementation scenario is not impacted, integration may increase cost-effectiveness and efficiency of the patient estimate surveys. Future development of the tool to enable additional diseases to be reported by SMS will increase the efficiency and effectiveness of integration. Additionally, data collected through an integrated survey using different approaches to those described here can be extracted and sent by SMS by the data collectors.
Following the framework and considering four of the key factors, there are two main methods of data collection and reporting that may be used. Firstly, the one-tier system in which the health worker both collects and reports the data with SMS. Secondly, a two-tier system in which a community health worker is the data collector, and collects the data on paper forms; the paper forms are delivered to a health worker (supervisor) who then sends the data via SMS.
Implementation of patient searching and reporting using MeasureSMS-Morbidity is not limited to the four scenarios described, however these scenarios were the most appropriate and effective mechanisms for implementation in the five countries tested to date. For example, where MDA is implemented using house-to-house delivery, it may be possible to collect patient data during the MDA registration or delivery (3,11). However, in IUs where community distribution posts are used to deliver treatments to the community, using the MDA to record patient data may result in under-reporting as it relies on the patients presenting at the distribution posts and reporting their conditions.
A one-tier data collection and reporting mechanism, such as scenario one is the simplest form of MeasureSMS-Morbidity. When considering the population demographics and relationships with health workers, CHWs or community volunteers will often be the most appropriate personnel to act as the data collector due to the closer relationships with the community. CHWs across the world play a crucial role in health systems achieving their potential, regardless of a countries development status (12). Integrating patient estimate surveys into these pre-existing health system structures is a strength of the implementation of the MeasureSMS-Morbidity tool and is crucial for its feasibility and success. As health workers are usually already overburdened by community health activities (13), it is important that the survey is timed appropriately so as not to compete with other health activities and needs.
This system is feasible in rural IUs in which health workers have a defined population within the catchment of their health facility. In rural scenarios, such as scenario 1, there are several factors that are linked to community ownership and access which will influence whether it is appropriate for health workers to be the data reporters, or to simply be the data collectors. Firstly, how familiar are the local health workers with sending an SMS? Experience has shown that in more rural settings, SMS use is less common than in urban settings (14), and personnel may therefore need to be trained in sending SMS, in addition to specifically reporting LF data through SMS. Secondly, how reliable is the phone network coverage? In remote rural areas, the coverage may be limited, restricting the frequency of data sending. Thirdly, how reliable is the power supply? If the survey is being implemented in an IU which experiences frequent power cuts, and access to generators is limited, the opportunities for charging a mobile phone will also be limited, again restricting the frequency of data sending. If any of these factors are likely, then it is necessary to identify additional personnel who will be more appropriate to act as the data reporters. As access to mobile phones is generally considered to be greater in urban areas (15), health workers located in a more ‘urban’ area within an IU with greater access to mobile phones and mobile phone network would be the appropriate data reporters.
Two-tier reporting mechanisms such as scenarios 2 and 3 will reduce the number of people that need to be trained in SMS reporting, as data collectors will only need to be trained in the identification of LF clinical conditions and only data reporters trained on sending the SMS. Additionally, implementing a two-tier reporting system reduces the burden of the survey on healthcare services by sharing the workload of data collection and reporting between health workers. This is especially important in areas with large population such as urban IUs, as implementing a two-tier reporting system will reduce the number of households that each CHW will need to visit and therefore reduce the length of time for the survey.
House-to-house census methods used in highly endemic areas provide an accurate estimate of patient numbers in an IU which enables countries to effectively plan and target resources equitably. However, in low endemic IUs, in which low patient numbers are anticipated, it is important to have a more cost- and time-effective implementation scenario, such as scenario 4. Use of patient records, health worker and community informants are all alternative methods for developing LF patient estimates. Scenario 4 combines these approaches using a small team of data reporters to enable more efficient data collection. This scenario is appropriate for use in IUs in which low numbers of patients are anticipated. While this method is not as vigorous as house-to-house patient searching, it will enable the programmes to determine the level of access to care that is required within each IU. In highly endemic IUs in which high numbers of patients are anticipated, this method may lead to under-reporting which may lead to inadequate levels of care being planned and provided.
ConclusionsOther Section
The MeasureSMS-Morbidity tool can be used with different approaches to obtain patient estimates. Using the data sent through SMS, an LF programme is able to map prevalence of clinical disease and identify priority areas in need of MMDP interventions, thus ensuring equitable access to care. Through experiences in five countries, four recommended implementation scenarios and a framework for effective application of the tool have been developed. To date, the tool has been used to report LF clinical case data obtained through house-to-house census, and active case finding using community and health worker informants.
Key factors have been described that should be considered when planning surveys in order to determine the most appropriate and effective method for each IU. While the scenarios have been developed based on experiences in African and Asian LF programmes, application of MeasureSMS-Morbidity is not limited to these four scenarios; it is feasible to utilise the tool to report patient information obtained through other survey types.
With only 41% of LF endemic countries reporting data on LF patients, and only 14% monitoring MMDP activities at the IU level [3], mechanisms to support country programmes to collect and report such data at the IU level, as required to meet elimination criteria, are essential to scale up MMDP activities. Reporting of LF clinical cases using MeasureSMS-Morbidity is an adaptable and rapid reporting system that can support country programmes to develop databases of patient estimates at any geographical level. As countries scale-up surveillance and MMDP activities, a tool such as MeasureSMS-Morbidity provides a mechanism to develop patient estimate databases within LF endemic areas, thus fulfilling one component of the elimination requirements.
AcknowledgementsOther Section
The authors would like to thank the country partners in Bangladesh, Ethiopia, Malawi, Nepal and Tanzania for their support in the implementation of MeasureSMS-Morbidity surveys which has led to the development of these scenarios. This work was supported by grants from the UK Department for International Development to the Centre of Neglected Tropical Diseases, Liverpool School of Tropical Medicine for the Lymphatic Filariasis Elimination Programme.
FootnoteOther Section
Conflicts of Interest: The authors have no conflicts of interest to declare.
Ethical Statement: The study was approved by the Research Ethics Committee at the Liverpool School of Tropical Medicine, UK.
ReferencesOther Section
- WHO. Lymphatic filariasis: Progress report 2000–2009 and strategic plan 2010–2020. World Health Organization, 2010.
- WHO. Preparing and implementing a national plan to eliminate filariasis in countries where onchocerciasis is not co-endemic. World Health Organization, 2000.
- WHO. Lymphatic filariasis: managing morbidity and preventing disability. World Health Organization, 2013.
- 489 Global Programme to eliminate lymphatic filariasis: progress report, 2014. Wkly Epidemiol Rec 2015;90:489-504. [PubMed]
- Mathieu E, Amann J, Eigege A, et al. Collecting baseline information for national morbidity alleviation programs: different methods to estimate lymphatic filariasis morbidity prevalence. Am J Trop Med Hyg 2008;78:153-8. [PubMed]
- Stanton M, Molineux A, Mackenzie C, et al. Mobile Technology for Empowering Health Workers in Underserved Communities: New Approaches to Facilitate the Elimination of Neglected Tropical Diseases. JMIR Public Health Surveill 2016;2:e2. [Crossref] [PubMed]
- Stanton MC, Mkwanda SZ, Debrah AY, et al. Developing a community-led SMS reporting tool for the rapid assessment of lymphatic filariasis morbidity burden: case studies from Malawi and Ghana. BMC Infect Dis 2015;15:214. [Crossref] [PubMed]
- WHO. The World Health Report 2006 - working together for health. World Health Organization, 2013.
- Sime H, Deribe K, Assefa A, et al. Integrated mapping of lymphatic filariasis and podoconiosis: lessons learnt from Ethiopia. Parasit Vectors 2014;7:397. [Crossref] [PubMed]
- Mwingira U, Chikawe M, Mandara WL, et al. Lymphatic filariasis patient identification in a large urban area of Tanzania: An application of a community-led Mhealth system. PLoS Negl Trop Dis 2017;11:e0005748. [Crossref] [PubMed]
- Smith EL, Mkwanda SZ, Martindale S, et al. Lymphatic filariasis morbidity mapping: a comprehensive examination of lymphoedema burden in Chikwawa district, Malawi. Trans R Soc Trop Med Hyg 2014;108:751-8. [Crossref] [PubMed]
- Perry HB, Zulliger R, Rogers MM. Community health workers in low-, middle-, and high-income countries: an overview of their history, recent evolution, and current effectiveness. Annu Rev Public Health 2014;35:399-421. [Crossref] [PubMed]
- Maes KC, Kohrt BA, Closser S. Culture, status and context in community health worker pay: pitfalls and opportunities for policy research. A commentary on Glenton et al. (2010). Soc Sci Med 2010;71:1375-8; discussion 1379-80. [Crossref] [PubMed]
- Bigna JJ, Noubiap JJ, Plottel CS, et al. Barriers to the implementation of mobile phone reminders in pediatric HIV care: a pre-trial analysis of the Cameroonian MORE CARE study. BMC Health Serv Res 2014;14:523. [Crossref] [PubMed]
- GSMA. The mobile economy: Sub-Saharan Africa 2014. 2014. Available online: https://www.gsma.com/mobileeconomy/archive/GSMA_ME_SubSaharanAfrica_2014.pdf
Cite this article as: Mableson HE, Martindale S, Stanton MC, Mackenzie C, Kelly-Hope LA. Community-based field implementation scenarios of a short message service reporting tool for lymphatic filariasis case estimates in Africa and Asia. mHealth 2017;3:28.