The fitness of apps: a theory-based examination of mobile fitness app usage over 5 months
Introduction
Combining the popularity of mobile devices with the on-going search for fitness, thousands of fitness applications (apps) are available for free or low cost. Apps allow users to set fitness goals, track activity, gather workout ideas, and share progress on social media (1). Characteristics such as a user-friendly interface, automatic tracking, and security are desired app characteristics among fitness app users (2,3). Studies have examined desirable app characteristics at one point in time (2,3), but long-term app usage and subsequent fitness behavior have not been adequately studied. There is also uncertainty if these apps help individuals achieve and maintain personal fitness long term. To further complicate, technology usage attrition in studies of eHealth technology is an issue that can negatively affect results (4,5).
Despite knowing these helpful app attributes to users, the standard in effective health behavior change is supported by theory-based interventions (6). Research suggests that apps based in health behavior theory, behavior change techniques, and evidence-based practices are lacking (7-11). This makes it difficult to determine usefulness of traditional health behavior change methods on mobile technologies (12). Furthermore, apps that do utilize constructs of health behavior theories may not apply all constructs of a particular theory (13,14), whether known by the app developer or not, making it not possible to determine the efficacy of the theory.
Behavior change techniques have been used in some fitness apps. An examination of the most highly reviewed health apps in the iTunes Apple Store determined that all 23 apps were lacking in theory-based behavior change strategies (15). Regarding behavior change techniques, a review of popular apps determined that most app descriptions described fewer than four behavior change techniques (16). Most common techniques included instruction on performance of exercises, modeling of exercises, feedback on performance, activity goal setting, and planning for social support and behavior change (16). Interestingly, more costly apps tend to be higher quality in terms of usability (11) and include some behavior change techniques (17).
Despite a lack of theory and behavior change techniques, some fitness technology strategies for behavior change have been found to be effective. For example, technology that tracks behavior over time and allows the user to see their own behavior trends in a visual format have been found to positively impact health behavior (18). Not surprisingly, apps that lack the ability to engage users lessen the impact of the app effectiveness on behavior change (1). Lastly, online advice from a personal coach was more influential on user behavior than peer support (18). It has also been suggested that apps utilize expertise from health professionals for monitoring and feedback as well as provide the user with a more personalized experience to increase adherence to health behaviors (13). In fact, a review of health apps suggested a lack of trustworthiness of apps could be ameliorated by having a behavior change expert aid in app development (19). The apps in the present study have several of these attributes.
The longevity of app usefulness on behavior change is unclear. In a literature review of physical activity-related smartphone apps, it appears that apps can be useful in promoting modest increases in physical activity for the short term (20), but long term studies are needed to determine ongoing lifestyle changes. For longer-term behavior change, users preferred the automatic tracking as well as help with tracking goal progress for a variety of activities (20). Tailored feedback to coach and motivate was also viewed as important (20). In another study, runners who used an app to train for a future running event reported improved self-esteem, feeling athletic, losing weight, and promoting running among others (21). These results suggest the potentially useful role of apps for longer-term behavior change. However, beyond the running event, the effect of this particular app is unknown.
Another problem area with apps is their lack of connection to national physical activity guidelines (22). In a review of 379 apps (22), no apps adhered to aerobic physical activity guidelines, though one app referenced these guidelines. Seven apps adhered only to guidelines for resistance training physical activity. Without a reference to the national physical activity guidelines, individuals may not be able to set goals for what is considered adequate physical activity (22).
Health, technology, information tracking of health behaviors, and the value of health behavior theory and behavior change techniques are important areas to better understand especially as technology becomes more prevalent in daily life. The Theory of Planned Behavior (TPB) (23) is an appropriate theory to use to understand technology and health behaviors for a couple reasons. For one, a validated TPB and exercise survey exists (24). Secondly, all constructs of TPB can be applied to exercise behavior. TPB considers the constructs of attitude, subjective norm, perceived behavioral control, and behavioral intention to impact behavior change. Attitude refers to beliefs about a behavior once expected outcomes are evaluated. Subjective norm pertains to what one considers to be a typical behavior and to what extent one is willing to comply. Perceived behavioral control considers control beliefs and ability to influence these beliefs. Lastly, behavioral intention is an individual’s perceived likelihood of engaging in a particular behavior. This intention may or may not lead to the actual behavior. In terms of application of TPB to the present study, each construct of the theory is relevant. First, anticipated outcomes from engaging with technology impact attitudes toward technology. Next, motivation to use technology is based on normative beliefs about technology, which is influenced by peers. Perceived behavioral control examines control over behavior and ability to influence behavior. In the case of technology, technology may be either a positive or negative control variable in that it may encourage or prevent exercise. Lastly, behavioral intention examines how attitude, subjective norm, and perceived behavioral control contribute to intention to engage in the behavior. In addition to the applicability of this theory to technology, a validated exercise and TPB survey already existed, which was modified to include exercise with technology (apps) along with exercise.
The present study examined usage of three fitness apps and its effect on TPB constructs and perceived fitness over 5 months. By understanding app usage, guidelines can be developed to create apps based in health behavior research to promote long-term physical activity. This study also examined attrition of technology usage by continuing to collect data from participants who stopped using the apps, but remained in the study.
Methods
The three fitness apps selected for this study were based on the Functional Triad (25) as well as from a focus group with fitness professionals to ensure each app fit strongly with one of the three types of technology in the Functional Triad. The Functional Triad suggests that there are three functions of technology in the way people react or use them: (I) a medium provides an experience; (II) a tool increases capability; and (III) a social actor creates relationships (25). Each of the three apps in the present study had a primary function as a medium that provided the user an experience in terms of a dashboard coordinating fitness efforts, a tool that increased capability in terms of prefabricated workouts, or social actor that created relationships with others seeking to improve fitness via a social media platform. Apps were available for free on Android and iOS.
A one-group pre-posttest design was utilized since a goal of this study was to examine technology usage attrition in addition to effectiveness of apps. Participants were allowed to use all three apps simultaneously to determine which app functions, as outlined in the Functional Triad (medium, tool, social actor), were utilized and found to be effective. After receiving IRB approval, 64 participants (17 men, 47 women) aged 18 or older were recruited in-person from a Midwest suburban fitness center between June 2014 and January 2015. An already active population was selected for the study because effects of fitness apps were being tested against exercise without technology. At recruitment, participants downloaded the three apps, but were not told the purpose of each app as a medium, tool, and social actor. Participants were told to use the apps however they preferred, as if they had found and downloaded them on their own.
Participants completed a validated TPB and exercise survey (24) at pretest and posttest regarding attitude, subjective norm, perceived behavioral control, and behavioral intention over exercise and exercise with apps. The bipolar adjective scales measured exercise attitude by descriptive paired adjective categories including useless/useful, foolish/wise, harmful/beneficial, unenjoyable/enjoyable, unpleasant/pleasant, boring/interesting, and stressful/relaxing. Subjective norm measured peer support and peer approval of exercise and exercise with apps. Perceived behavioral control examined control over and barriers to exercise and exercise with apps. According to the authors of the instrument (24), a subscale total score is calculated by summing scores of individual items (each ranging from 1 to 7) in each construct. Lastly, behavioral intention to exercise and to exercise with apps asked about the number of times the participant intended to exercise and exercise with apps over the next 2 weeks in six groups (0, 1–3, 4–6, 7–9, 10–12, and 12 times or more).
Participant app usage was tracked at months 1, 3, and 5 after download to determine usage and perceived fitness. Participants received an email with a checkpoint survey that inquired about times per month of app usage for each app and minutes of usage per each time for each app. The amount of app use was calculated by multiplying the frequency of app use per month and the minutes of use each time. At month 5, participants also received the posttest survey via email. Additionally, fitness perception was measured at pretest, checkpoint one, checkpoint two, and checkpoint three (posttest). Measurements of perceived fitness on a 12-point scale included cardiovascular fitness, muscular strength, muscular endurance, flexibility, and body composition. If participants did not respond to the initial checkpoint email, they were sent one reminder email.
Survey data were analyzed using statistical software Stata (26). Our analysis focused on comparisons of pre-post differences and comparisons of those who used apps (users) and those who did not (non-users). Paired sample t-tests analyzed data for pretest to posttest comparisons of (I) individual item scores for constructs of attitude, subjective norm, and perceived behavioral control; (II) subscale total scores for attitude, subjective norm, and perceived behavioral control for each exercise and exercise with apps; and (III) perceived fitness scores. A pre-post difference in behavioral intention over exercise and exercise with apps was examined using a sign test for matched pairs. A sign test is appropriate for ordinal variables because the non-parametric test compares scores of matched or paired samples without requiring the outcome’s distribution to be normal or symmetric (27). The sign test produces the results of three hypothesis tests: whether the median of the differences between paired observations is positive, negative, and zero (26).
Baseline characteristics of users and non-users were examined to identify types or statuses of individuals who are more likely to use mobile apps for exercise. Fisher’s exact tests were used for categorical (e.g., gender, race, marital status) and ordinal (e.g., behavioral intention) independent variables, and simple logistic regression was used for continuous independent variables (e.g., subscale total scores of TPB constructs) with app use status as the dependent variable. In addition, we examined whether app use is associated with TPB constructs at posttest. Independent sample t-tests were used to examine differences between users and non-users in subscale total scores for attitude, subjective norm, and perceived behavioral control at posttest. Fisher’s exact tests were used to compare behavioral intention over exercise and exercise with apps at posttest between users and non-users. T-tests were used to examine whether the amount of app use (minutes of use per month) was different between two different checkpoints for each app. Simple linear regression was used to examine whether the amount of app use (minutes of use per month) is associated with TPB constructs or perceived fitness at posttest.
Results
Sample characteristics
Among the 64 individuals who participated in the pretest, 47 completed the posttest for the 5-month study (73.4%). Table 1 describes the characteristics of the sample that participated in both the pretest and the posttest. Approximately three quarters of the participants were female (74.5%) and most were white (91.5%) and aged less than 55 (80.8%). A majority was college educated (82.8%), married (85.1%), and worked 30 or more hours per week (55.3%).
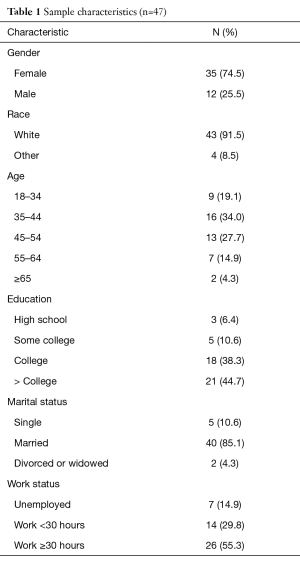
Full table
App use patterns
Overall, the amount of app use per month declined between checkpoint one and checkpoint three for all three apps (Figure 1). For both app 1 and app 2, the mean of app use in minutes decreased significantly between checkpoint one and checkpoint two (P<0.05) with no significant difference between checkpoints two and three. For app 3, the mean of app use in minutes was not significantly different between each checkpoints one and two and checkpoints two and three. However, the mean of app use in minutes at checkpoint three was significantly lower than that of checkpoint one (P<0.01). The amount of app use in minutes was significantly different between app 2 and app 3 at the first checkpoint only (56 vs. 14, P<0.05). There was no significant difference in the amount of app use between any apps at other checkpoints.
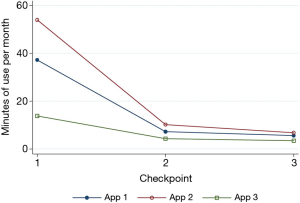
Pre-posttest comparison
From pre to posttests, there were significant decreases in several individual item scores and three subscale total scores of TPB constructs.
Individual item scores of TPB constructs
Overall, there was no significant change in individual item scores between pre and posttest with several exceptions (Table 2). A significant decrease was observed in five items: usefulness of using apps for exercise (attitude), peer influence on exercise (subjective norm), peer influence on exercise with apps (subjective norm), perceived difficulties in exercising with apps (perceived behavioral control), and the expected frequency of exercise with apps over the next 2 weeks (behavioral intention). The decrease in individual item scores ranged from 0.51 to 1.29. For example, the mean score for peer influence on exercise decreased by 0.51 point (P<0.05). Behavioral intention to exercise with apps significantly lowered (P<0.0001 in a sign test). At posttest, among 33 individuals, the expected frequency of exercise with apps over the next 2 weeks decreased, while it increased among only five individuals. Nine individuals showed no change in their intention between pre and posttest.
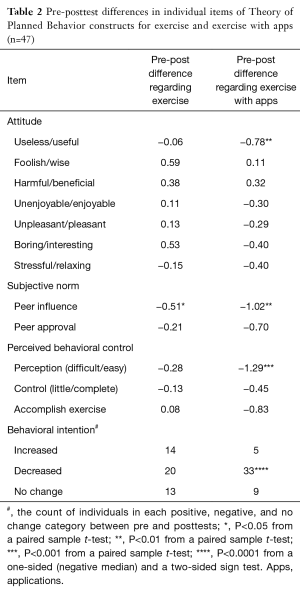
Full table
Subscale total scores of TPB constructs
There were significant differences in three subscale total scores pre to posttest (Table 3). Subjective norm regarding exercise (−0.72, P<0.05), subjective norm regarding exercise with apps (−1.72, P<0.01), and perceived behavioral control over exercising with apps (−2.56, P<0.01) showed a significant decrease between pre and posttests.
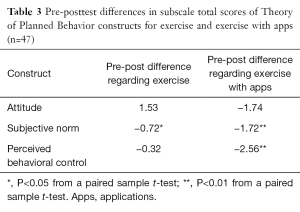
Full table
Fitness perception
There were no statistically significant changes in any of the fitness perception areas over 5 months: cardiovascular fitness, strength, endurance, flexibility, and body composition (results not shown).
Comparison of users and non-users
There was no difference in baseline characteristics that determined who becomes an app user or a non-user over the study period. Overall, app use was not associated with TPB constructs or perceived fitness at posttest.
Characteristics of users and non-users
Those who used the apps in the study were compared to those who did not use the apps, but remained in the study. Among those who participated in both pretest and posttest, 32 participants used any app at least once and 15 participants never used the apps. According to Fisher’s exact tests, there was no difference in app use status (ever used or not used) by gender, ethnicity, education, marital status, employment status (employed for at least 30 hours per week), frequency of exercise per week at baseline, exercise intensity at baseline, and behavioral intention to exercise at baseline (results not shown). According to simple logistic regression, subscale total scores of TPB constructs at baseline were not associated with app use status (results not shown).
Effects of app use
There was no significant differences in subscale total scores of TPB constructs at posttest between app users and non-users except for one (Table 4). At posttest, the attitude toward exercising using apps was significantly more favorable among users than non-users (32.3 vs. 27.6, P<0.05). There were no statistically significant difference in perceived fitness (cardiovascular fitness, muscular strength, muscular endurance, flexibility, and body composition) between users and non-users at posttest either (results not shown).
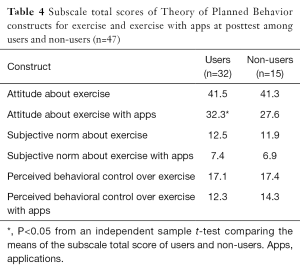
Full table
The amount of app use was not associated with TPB constructs or perceived fitness at posttest. According to simple linear regression, the amount of app use, measured by the minutes of use per month, was not associated with any of TPB constructs or perceived fitness scores at posttest (results not shown).
Discussion
This study examined the long-term adherence of fitness app use and effectiveness of fitness app use on TPB constructs and perceived fitness. Unlike previous research, this study looked at app usage over 5 months rather than a point in time. This study also used a health behavior theoretical framework as well as a technology function framework to guide the research.
When examining individual item scores, a decrease pre to posttest occurred for usefulness of using apps for exercise (attitude). Attitude of exercising with apps shifted from slightly positive to neutral. Likewise, peer influence on exercise (subjective norm) and peer influence on exercise with apps (subjective norm) decreased over the 5-month study suggesting that peers did not impact study participant behavior of exercise without apps and with apps. Another decrease was in perceived behavioral control, indicating that perceived ease in exercising with apps decreased. Participants found it more difficult to use the apps over time, which is the opposite of what may be expected. Participants did not become more comfortable using apps during the 5-month study. Lastly, the expected frequency of exercise with apps over the next 2 weeks (behavioral intention) decreased, but the expected frequency of exercise (without apps) remained similar pre to posttest. This may be because apps were not deemed necessary to complete exercise.
Subscale total scores of three TPB constructs, subjective norm regarding exercise, subjective norm regarding exercise with apps, and perceived behavioral control over exercising with apps, decreased pre-post. For subjective norm, the decrease in scores indicates that beliefs about the importance of peers for exercise, regardless of apps involved or not, weakened from pretest to posttest. This may be because the study participants were already physically active and did not require peer support. Also, given the average age of the study participants, peer influence may not be as influential as it would be for younger people. Diminished perceived behavioral control over exercising with apps may indicate that apps were seen as an external force that was more controlling of the user (e.g., the user had to input information) than the user controlling the app.
There were no statistically significant differences between users and non-users at posttest except for attitude toward exercising using apps, which was significantly more favorable among users than non-users. App users might become more positive about app use because they recognized the value of the apps through usage.
Low study attrition was a highlight of this study (26.6%) with 17 out of 64 people dropping out of the study. Technology usage attrition (study participants who remained in the study even though they stopped using the apps) was also desirable at 31.9%. This rate is much better than the average rate of 50% (28). Of the remaining 47 study participants, 32 were users and 15 were non-users. The low attrition in this study may be because participants were specifically asked to continue filling out checkpoint surveys and the posttest regardless of usage. Interestingly, fitness perception did not change in user and non-users. Therefore using and not using the apps had no impact on fitness perception. It would be expected that with app usage, users would actually engage in more exercise or perceive being fitter due to the awareness apps bring to one’s fitness status.
App usage decreased with the most significant drop occurring from month 1 to month 3 in two apps (app 1—the medium and app 2—the tool). This suggests that app users decide the utility of apps early on in usage. Interestingly, app 3 with the social actor function had the lowest usage from the beginning of the study. This is surprising because of the popularity of social media and getting support and feedback from online “friends”. However, participants in the study did provide qualitative feedback at the periodic checkpoints (no included in these results) that they did not like sharing their fitness successes and failures with strangers.
Since the apps used in this study were not theory-based, it cannot be determined if and which theory would be most beneficial in promoting exercise behavior change. Prior research suggests the necessity of theory-based apps for long-term adherence to health behavior change (7,8,10) or app developers use evidenced-based content in app development (10,29). Until theory and/or evidenced based-content becomes more prevalent in fitness technology development, apps are tested, and efficacy of technology improves, users should select technology that that suits their personal preferences (30). However, it was helpful to utilize a theory to understand app usage behaviors in the present study. Furthermore, health practitioners should be cognizant of the limitations of apps when making suggestions to clients and patients (31). A realistic perspective on the restricted ability of fitness technology can prevent disappointment and wasted time and money in the future. Also helpful tools for assessing apps are becoming available (32), which provide users and practitioners the ability to select apps with some assurance and guidance that the technology may assist behavior change. However, until current fitness technology usage is better understood and apps are developed with more health behavior science as a foundation, fitness apps will not likely serve as a catalyst for or supporter of improved fitness among users. The findings of this study suggest that app usage and effectiveness should be researched before new apps are developed and available to the public. Understanding how people use apps (and other fitness technology) and reasons why they stop usage should inform future technology.
There are a few limitations to this study. Regarding the checkpoints, recall bias is an issue as study participants were considering their app usage in the previous 5 to 6 weeks. An additional limitation is that technology-minded people engaged in the study as all participants needed a smartphone to participate. The participants of this study may be more favorable towards technology than non-smartphone users. Another limitation is that all participants in this study were physically active to some degree. Though less active and more active study participants at baseline showed no difference in outcomes at posttest (results not shown), a future study should be conducted in a non-exercising population to determine the apps’ effects on an inactive population. Lastly, this study was one group pre-posttest design, not an experimental study with a control group. This design allowed for examine of attrition, but with more time and funding, a randomized control group experimental design would be beneficial.
Despite these limitations, this study examined the effect of long-term use of apps rather than app experience at one point in time or over the short term. This study was also grounded in TPB (23) as well as the Functional Triad (25) as a means of differentiating app purposes. Also, low attrition of study participants and study technology usage of the three apps was a highlight of the present study at 26.6% and 31.9%, respectively. Studying technology attrition, in particular, helps provide an understanding of potential differences among users and non-users of apps.
In summary, to increase app adherence and effectiveness, it may be particularly important to focus on usefulness (attitude) and ease of use (perceived behavioral control) while focusing less on peer influence (subjective norm). Additionally, helping users connect with the apps immediately will lead to a more engaged user with a more positive attitude about the apps. In this study, it was helpful to use theoretical frameworks to understand apps and user behavior. A potential way to develop sound apps could be through the use of health behavior theories and frameworks. Users of apps should be conscientious of the current limited abilities of apps and how these abilities relate to personal health goals before they invest in apps. Developers and users should consider the ultimate goal of fitness apps should be to engage users in long-term health behavior change.
Acknowledgements
This study was supported by Northern Illinois University.
Footnote
Conflicts of Interest: The authors have no conflicts of interest to declare.
Ethical Statement: The study was approved by the institutional review board of Northern Illinois University (No. HS14-0129), and written informed consent was obtained from all participants.
References
- Sama PR, Eapen ZJ, Weinfurt KP, et al. An evaluation of mobile health application tools. JMIR Mhealth Uhealth 2014;2:e19. [Crossref] [PubMed]
- Dennison L, Morrison L, Conway G, et al. Opportunities and challenges for smartphone applications in supporting health behavior change: qualitative study. J Med Internet Res 2013;15:e86. [Crossref] [PubMed]
- Rabin C, Bock B. Desired features of smartphone applications promoting physical activity. Telemed J E Health 2011;17:801-3. [Crossref] [PubMed]
- Eysenbach G. The law of attrition. J Med Internet Res 2005;7:e11. [Crossref] [PubMed]
- Kohl LF, Crutzen R, de Vries NK. Online prevention aimed at lifestyle behaviors: a systematic review of reviews. J Med Internet Res 2013;15:e146. [Crossref] [PubMed]
- Glanz K, Rimer BK, Viswanath K. editors. Health Behavior and Health Education: Theory, Research, and Practice. 4th edition. San Francisco: Jossey-Bass, 2008.
- Middelweerd A, Mollee JS, van der Wal CN, et al. Apps to promote physical activity among adults: a review and content analysis. Int J Behav Nutr Phys Act 2014;11:97. [Crossref] [PubMed]
- Kratzke C, Cox C. Smartphone technology and apps: rapidly changing health promotion. Int Electron J Health Educ 2012;15:72-82.
- Yang CH, Maher JP, Conroy DE. Implementation of behavior change techniques in mobile applications for physical activity. Am J Prev Med 2015;48:452-5. [Crossref] [PubMed]
- Breton ER, Fuemmeler BF, Abroms LC. Weight loss-there is an app for that! But does it adhere to evidence-informed practices? Transl Behav Med 2011;1:523-9. [Crossref] [PubMed]
- Cowan LT, Van Wagenen SA, Brown BA, et al. Apps of steel: are exercise apps providing consumers with realistic expectations?: a content analysis of exercise apps for presence of behavior change theory. Health Educ Behav 2013;40:133-9. [Crossref] [PubMed]
- Riley WT, Rivera DE, Atienza AA. Health behavior models in the age of mobile interventions: Are our theories up to the task? Transl Behav Med 2011;1:53-71. [Crossref] [PubMed]
- Brunstein A, Brunstein J, Mansar SL. Integrating health theories in health and fitness applications for sustained behavior change: Current state of the art. Creative Educ 2012;3:1-5. [Crossref]
- Payne HE, Lister C, West JH, et al. Behavioral functionality of mobile apps in health interventions: a systematic review of the literature. JMIR Mhealth Uhealth 2015;3:e20. [Crossref] [PubMed]
- Azar KM, Lesser LI, Laing BY. Mobile applications for weight management: Theory-based content analysis. Am J Prev Med 2013;45:583-9. [Crossref] [PubMed]
- Conroy DE, Yang C, Maher JP. Behavior change techniques in top-ranked mobile apps for physical activity. Am J Prev Med 2014;46:649-52. [Crossref] [PubMed]
- Direito A, Dale LP, Shields E, et al. Do physical activity and dietary smartphone applications incorporate evidence-based behaviour change techniques? BMC Public Health 2014;14:646. [Crossref] [PubMed]
- Connelly J, Kirk A, Masthoff J, et al. The use of technology to promote physical activity in Type 2 diabetes management: a systematic review. Diabetic Med 2013;30:1420-32. [Crossref] [PubMed]
- Chatzipavlou IA, Christoforidou SA, Vlachopoulou M. A recommended guideline for the development of mHealth Apps. mHealth 2016;2:21. [Crossref]
- Coughlin SS, Whitehead M, Sheats JQ, et al. A review of smartphone applications for promoting physical activity. Jacobs J Community Med 2016;2. pii: 021.
- Dallinga JM, Mennes M, Alpay L, et al. App use, physical activity and healthy lifestyle: a cross sectional study. BMC Public Health 2015;15:833. [Crossref] [PubMed]
- Knight E, Stuckey MI, Prapavessis H, et al. Public health guidelines for physical activity: is there an app for that? A review of android and apple app stores. JMIR Mhealth Uhealth 2015;3:e43. [Crossref] [PubMed]
- Ajzen I. Perceived behavioral control, self-efficacy, locus of control, and the Theory of Planned Behavior. J Appl Soc Psychol 2002;32:665-83. [Crossref]
- Rhodes RE, Jones LW, Courneya KS. Translating exercise intentions to behavior. J Health Psychol 2003;8:447-58. [Crossref] [PubMed]
- Fogg BJ. Persuasive Technology: Using Computers to Change What We Think and Do. San Francisco: Morgan Kaufmann, 2002.
- StataCorp. Stata 12 Base Reference Manual. College Station, TX: Stata Press, 2011.
- Hald A. A History of Probability and Statistics and their Applications Before 1750. New York: Wiley, 2003.
- Kelders SM, Kok RN, Ossebaard HC, et al. Persuasive system design does matter: a systematic review of adherence to web-based interventions. J Med Internet Res 2012;14:e152. [Crossref] [PubMed]
- Bardus M, van Beurden SB, Smith JR, et al. A review and content analysis of engagement, functionality, aesthetics, information quality, and change techniques in the most popular commercial apps for weight management. Int J Behav Nutr Phys Act 2016;13:35. [Crossref] [PubMed]
- Tate DF, Lyons EJ, Valle CG. High-tech tools for exercise motivation: use and role of technologies such as the internet, mobile applications, social media, and video games. Diabetes Spectr 2015;28:45-54. [Crossref] [PubMed]
- West JH, Hall PC, Hanson CL, et al. There's an app for that: content analysis of paid health and fitness apps. J Med Internet Res 2012;14:e72. [Crossref] [PubMed]
- Stoyanov SR, Hides L, Kavanagh DJ, et al. Mobile app rating scale: a new tool for assessing the quality of health mobile apps. JMIR Mhealth Uhealth 2015;3:e27. [Crossref] [PubMed]
Cite this article as: Herrmann LK, Kim J. The fitness of apps: a theory-based examination of mobile fitness app usage over 5 months. mHealth 2017;3:2.