A mHealth application to identify cognitive communication disorder after right hemisphere stroke: development and beta testing
Highlight box
Key findings
• A mobile health (mHealth) application to screen for cognitive communication disorder (CCD) after right hemisphere (RH) stroke is a feasible evidence-based assessment option for speech-language pathologists in a hospital environment.
• Identified user interface issues should be addressed to improve the user experience of the app.
What is known and what is new?
• Literature suggests that CCD may occur in more than 50% of patients admitted with RH stroke. Despite this high prevalence, limited evidence-based screening tools exist to identify the disorder.
• We developed the first mHealth application to screen for CCD after RH stroke using expert consultation, theory and current evidence.
• Evaluation of user acceptance highlights that speech-language pathologists may find this app useful in their clinical practice. Patients responded well to an alternative method of assessment.
What is the implication, and what should change now?
• Development of an evidence-based screening tool app to identify CCD after RH stroke addresses a significant gap in best practice stroke management.
• App development should routinely include evaluation of the user experience and consideration of the digital literacy level of intended users.
Introduction
Cognitive communication disorder (CCD) affects between 50–80% of individuals with right hemisphere (RH) stroke (1-4). The presentation of CCD varies significantly among individuals with RH stroke. Impairments may be present in lexical semantics (e.g., comprehension of abstract or non-literal language), discourse (e.g., impoverished, or verbose conversation), prosody (e.g., use of intonation, pitch, rhythm, and rate in speech to convey an emotion), and/or pragmatics (e.g., use of non-verbal behaviours such as body language, gesture, or eye contact) (5). Cognitive changes (e.g., executive function, attention, memory, neglect) may also co-occur (5). Published screening tools to identify CCD after RH stroke have limitations in their validity and reliability (6), focus on cognition, and fail to include critical tests for pragmatics and prosody comprehension, which are commonly affected after RH stroke (6-8).
Paper-based assessments are the most common method for assessing cognition and communication impairment after stroke (9,10). Speech-language pathologists (SLPs) working in acute stroke settings typically use their own locally developed or non-standardised paper-based assessments to identify impairments in language function (i.e., aphasia) (11). Similarly, when identifying CCD after RH stroke, SLPs are more likely to use observation or informal paper-based assessments (12). SLPs often choose to administer non-standardised or informal assessments as there is a need to be time efficient in acute stroke settings (11). However, a challenge in using non-standardised or informal assessments is that diagnoses of cognition and communication impairment may be missed (11,12). A missed diagnosis may significantly affect an individual’s ability to access and receive timely rehabilitation (13).
With the growing popularity of mobile health (mHealth) (a category of digital health), there is an opportunity to develop an alternative to paper-based assessment. Several mHealth apps have been developed to screen for aphasia and cognitive impairment after stroke: the Mobile Aphasia Screening Tool (MAST) (14) to identify aphasia, the Oxford Cognitive Screen-Plus (OCS-Plus) (15) to identify subtle cognitive impairment and the Oxford Digital Multiple Errands Test (OxMET) (16) to identify executive dysfunction following stroke. In these studies, all three apps were found to have high correlations with paper-based assessments measuring the same construct. Although not formally explored, two studies (14,15) included qualitative information about usability. The MAST was found to be efficient and user-friendly, but tablet (touch screen sensitivity) and user interface (automatic scoring) issues were reported. Strengths of the OCS-Plus included the use of standardised administration instructions and automatic scoring. However, image quality was an issue for participants with a visual impairment, and tablet issues reported included screen damage (e.g., cracks) and battery life. The OxMET did not report on usability.
Evaluation of usability can lead to an improved user experience of a new product or technology (17). According to Nielsen (18), usability encompasses five key features: ease of learning, efficiency, memorability, error rate and restoration, and satisfaction. Beta testing is an essential step in software development and involves the evaluation of app usability from a small group of intended users (19). Feedback is gathered from users and improvements are identified and resolved before the app is released to a larger audience (19).
For a new technology to be successfully embedded into routine clinical practice, user acceptance of both patients and health professionals must be evaluated (20). Validated scales, questionnaires, and semi-structured interviews with users based on models of usability and acceptance are commonly used methods for usability evaluation (20,21). One frequently used model is the Unified Theory of Acceptance and Use of Technology (UTAUT) (22). This model suggests that four main constructs may influence behaviour intention and use: performance expectancy (usefulness), effort expectancy (ease of use), social influence and facilitating conditions (infrastructure, support). The UTAUT has been applied previously in studies investigating both patient (23,24) and health professional (25,26) acceptance of mHealth technologies.
We developed the first mHealth app, the Right Hemisphere Cognitive Communication Screener (RECOGNISE), to identify CCD after RH stroke. The aim of this study was to beta test the app with intended users. As such, we explored user acceptance in patients with RH stroke and SLPs by applying components of the UTAUT in our evaluation.
Methods
Participants
Patients admitted with RH stroke and SLPs were recruited from acute stroke and inpatient rehabilitation wards at a hospital in Queensland, Australia. Inclusion criteria for the RH stroke group were (I) unilateral RH stroke confirmed by brain imaging (either computed tomography scan or magnetic resonance imaging) or a clinical diagnosis by a stroke or rehabilitation physician; (II) aged 18 years or over; and (III) primary language was English. Exclusion criteria were (I) previous history of other neurological and/or psychiatric conditions including dementia, left hemisphere stroke and traumatic brain injury; and (II) medically unstable. Inclusion criteria for the SLP group were (I) possession of a relevant university speech pathology qualification; and (II) minimum 2 years’ clinical experience as a SLP.
Nielsen (27) recommended including 3–4 users in usability studies if testing two different groups of intended users. A total of six participants with RH stroke and three SLPs participated in this study. Similar participant numbers have been reported in previous mHealth usability studies (28-30). The study was conducted in accordance with the Declaration of Helsinki (as revised in 2013). Ethical approval was received from Metro South Human Research Ethics Committee (No. HREC/2022/QMS/85007). All participants provided written informed consent prior to inclusion in the study.
App development
The App Factory, associated with the School of Information and Communication Technology at Griffith University, custom built the alpha version of the app using an Android operating system. An Android operating system was selected for initial development due to the availability of compatible hardware and ease of use. An iOS operating system was not considered feasible as the Apple iOS testing application (TestFlight) expires after 3 months. The app was written in C# using Xamarin Form (a cross-platform mobile application development tool) as the intention was to trial the iOS version after beta testing.
The app was developed in such a way that cybersecurity risks are minimised (see Figure 1). All data is stored locally in the internal storage of the device. The generated data (recordings, test results, and PDF data) stored in the internal storage will be deleted once the app is deleted from the device. Furthermore, to comply with the Information Privacy Act 2009 (Qld) (31), RECOGNISE contains no patient-related or personally identifiable data. The data file names are generated using the creation date (time stamp) and do not contain any personally identifiable information. The software architecture is considered a ‘flat’ app. As such, there are no servers or external connections required.
App content and characteristics
RECOGNISE is designed to identify the presence of CCD after RH stroke. Test items for RECOGNISE were developed by the research team following consultation with expert SLPs (32). RECOGNISE consists of 17 test items across five domains, four related to communication deficits associated with RH stroke: lexical semantics, prosody, discourse, and pragmatics, and one to cognition (see Figure 2). These test items screen for key features of CCD after RH stroke, including pragmatics, emotional prosody comprehension, narrative discourse production, conversational discourse production and lexical semantics.
Key features of RECOGNISE include the use of standardised instructions for the SLP, automatic scoring of patient responses via touchscreen, audio recording of discourse production test item responses allowing the SLP to listen back and analyse elements of discourse such as macrostructure and cohesion, use of short audio files in test items for emotional prosody comprehension, use of short videos in test items for emotion recognition and social inferences, use of high resolution, coloured illustrations in test items for neglect and narrative discourse production, and collation of scores as a summary report, which may be downloaded as a PDF. See Figure 3 for an example test item. The estimated time to complete the screening tool is approximately 30 min.
Measurements
Demographic characteristics
Demographic information was collected from participants with RH stroke. Demographic information such as years of SLP experience and current workplace setting was obtained from the SLP participants. All participants rated their prior experience with, and frequency of smartphone and tablet use through a short survey, comprising four 5-point Likert scale questions (see Appendix 1). The SLP participants were also asked to rate how frequently they screen patients admitted with RH stroke for CCD and name the screening tools used.
User acceptance methods
The beta test evaluation investigated user acceptance in both participant groups using surveys designed by the research team based on components of the UTAUT (see Appendix 1). After the screen, participants with RH stroke completed a short survey comprising two 5-point Likert scale questions, probing ease of use and satisfaction, and two open-ended questions asking participants to describe what they liked about the app, and what could be improved. After becoming familiar with the app, SLP participants were asked to complete a survey using a secure, web-based software platform, Research Electronic Data Capture (REDCap), hosted at Griffith University (33,34). The SLP survey comprised twelve 5-point Likert scale questions based on the four constructs of the UTAUT: performance expectancy, effort expectancy, social influences and facilitating conditions. A further two open-ended questions explored aspects of the RECOGNISE App that SLP participants liked and those that could be improved.
Procedure
SLP participants used a Samsung 12.4” Galaxy Tab S7 housing the beta-version of RECOGNISE. SLP participants received written and video instructions on how to use the app prior to use with RH stroke participants. The SLP administered RECOGNISE with the RH stroke participant in their usual workplace setting (e.g., on the ward, at bedside, in a treatment room). Immediately following completion of the screen, participants with RH stroke completed the short user acceptance survey with assistance provided by the SLP if required. The SLPs were asked to complete the online user acceptance survey once they had completed the app with at least three participants with RH stroke or once they felt sufficiently familiar with the app.
Statistical analysis
Data from participant surveys were analysed using descriptive statistics. We used qualitative content analysis (35) to analyse SLP participant responses to the open-ended survey questions. This analysis included first cycle coding, pattern coding and proposition development to identify categories and subcategories (35).
Results
Six participants with RH stroke (four male, two female) participated in beta testing RECOGNISE (see Table 1). The average age was 65.34 years (range, 37–82 years) and the average years of formal education completed was 11 years (range, 10–12 years). All participants were right-handed. Approximately two-thirds of the participants with RH stroke (n=4) presented with a pre-existing visual impairment and one-third (n=2) presented with a hearing impairment. All participants were admitted to hospital with an acute RH stroke. Average time post-stroke was 17.5 days (range, 6–41 days). Most participants had little or some experience using smartphones (n=4) and their experience using a tablet ranged from not at all experienced to very experienced. Participants reported using smartphones frequently and tablets rarely.
Table 1
Demographic characteristics | P1 | P2 | P3 | P4 | P5 | P6 |
---|---|---|---|---|---|---|
Gender | M | F | M | M | M | F |
Age (years) | 37 | 52 | 76 | 73 | 82 | 72 |
Education (years) | 12 | 10 | 10 | 12 | – | 11 |
Handedness | R | R | R | R | R | R |
Pre-existing visual impairment | No | Yes | Yes | Yes | Yes | No |
Pre-existing hearing impairment | No | Yes | No | Yes | No | No |
Site of stroke | R MCA | R basal ganglia | R MCA | R PCA, ACA & MCA | R MCA | R MCA |
Time post-stroke (days) | 21 | 41 | 6 | 15 | 12 | 10 |
Upper limb impairment | Yes | Yes | Yes | Yes | No | Yes |
Experience using smartphonesa | 3 | 4 | 3 | 3 | 2 | – |
Frequency using smartphonesb | 4 | 5 | 4 | 5 | 4 | – |
Experience using tabletsa | 3 | 2 | 4 | 2 | 1 | – |
Frequency using tabletsb | 1 | 1 | 4 | 2 | 1 | – |
a, on a 5-point scale where 1= not at all experienced, and 5= very experienced; b, on a 5-point scale where 1= never, and 5= all the time. RH, right hemisphere; P, participant; M, male; F, female; R, right; MCA, middle cerebral artery; PCA, posterior cerebral artery; ACA, anterior cerebral artery.
Three SLPs participated in beta-testing of RECOGNISE (see Table 2). All participants were female with an average age of 33 years (range, 25–38 years). The average years’ of SLP experience ranged from 2.5–16 years (average 8.5 years). Two SLPs worked in an inpatient rehabilitation unit and one SLP worked in an acute hospital setting. Participants in this group reported they were very experienced using smartphones and experienced using tablets. SLP participants reported using smartphones all the time and tablets frequently. SLPs reported sometimes (n=1) or frequently (n=2) screening for CCD after RH stroke. Assessments used to screen for CCD after RH included locally developed informal screens and the Sheffield Screening Test for Acquired Language Disorders (36).
Table 2
Demographic characteristics | SLP1 | SLP2 | SLP3 |
---|---|---|---|
Gender | F | F | F |
Age (years) | 36 | 25 | 38 |
SLP experience (years) | 7 | 2.5 | 16 |
Current workplace setting | Inpatient rehabilitation, hospital | Acute, hospital | Inpatient rehabilitation, hospital |
Experience using smartphonesa | 5 | 5 | 5 |
Frequency using smartphonesb | 5 | 5 | 5 |
Experience using tabletsa | 3 | 5 | 4 |
Frequency using tabletsb | 3 | 4 | 4 |
Frequency screening for RH CCDb | 4 | 4 | 3 |
a, on a 5-point scale where 1= not at all experienced, and 5= very experienced; b, on a 5-point scale where 1= never, and 5= all the time. SLP, speech-language pathologist; F, female; RH, right hemisphere; CCD, cognitive-communication disorder.
User acceptance
Participants with RH stroke
All participants (n=6) agreed that the RECOGNISE App was easy and enjoyable to use. Responses to open-ended questions revealed that participants in this group thought the app was “easy to understand” and “made me think a bit”. Suggestions for improvements included changing the positioning and size of images to account for pre-existing visual impairments and increasing the loudness of test items.
SLP participants
All SLP participants completed the online survey. In terms of performance expectancy (i.e., usefulness), all SLP participants agreed that they would find RECOGNISE useful in their SLP role (see Figure 4). However, participants did not agree that it would allow them to be more efficient or productive when screening for CCD after RH stroke. Most participants (n=2) agreed that the app was clear and understandable but were mixed in their opinion of how easy the app was to use. All participants agreed that learning how to use the app was easy and agreed it would not be difficult to become skilful at using the app. Two SLP participants agreed that their organisation was supportive of them using the app in their clinical practice. All participants agreed that they had the resources and knowledge necessary to use RECOGNISE, and that there was support available to assist with any technical difficulties. Participants were unsure if the app was compatible with current health information systems.
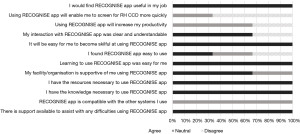
Qualitative content analysis of open-ended responses revealed three main categories: content of test items, user interface, and accessibility (see Figure 5). In terms of test item content, participants felt that the screen was comprehensive with test items based on evidence and potential CCD presentations (e.g., “considers all aspects of CCD including linguistic and emotional prosody”—Participant 3). One participant highlighted a test item (procedural discourse production) where content needed improvement (e.g., “none of the patients scored highly because the detail required seems unreasonable”—Participant 1).
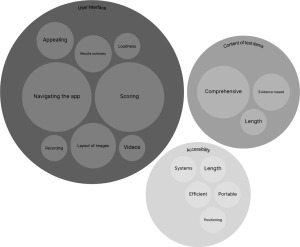
Positive user interface features included the presence of automatic scoring, use of videos, the collated results summary, and the ability to record responses. SLP participants reported a positive patient response to RECOGNISE (e.g., “the app seemed appealing to patients”—Participant 1). However, several user interface issues were identified including navigating within the app (e.g., “the lack of a go back button…makes it feel clunky and slow”—Participant 1) and difficulties interpreting the results summary (e.g., “the auto-generated data collection is very difficult to interpret”—Participant 3). Participants also identified challenges with the loudness of some audio test items and scoring (e.g., “scoring needs to be simplified”—Participant 3). SLP participants suggested that the layout of test items with photographs or illustrations needed improvement (e.g., “the detail in the pictures is so small and impossible to see”—Participant 1).
In terms of accessibility, one participant appreciated the portability of the tablet (e.g., “tablet-based makes it more compact”—Participant 3) whereas one participant felt the tablet was difficult to position (e.g., “holding the tablet and trying to pass it back and forth made the test longer to complete and awkward”—Participant 1). Another participant reported that the screen took too long to complete (e.g., more than 30 min) whereas another participant reported it was time efficient. A suggestion to improve accessibility included ensuring the app was compatible with existing medical record systems.
Discussion
We developed and beta tested the first mHealth app, RECOGNISE, to identify CCD after RH stroke. RECOGNISE is the first screening tool app developed for this population, and one of only a few screening tool apps developed to identify communication or cognitive impairment post-stroke (14-16). We gained valuable knowledge and insights about the development of mHealth apps, specifically the importance of including intended users in the development process. In the present study, all participants with RH stroke and most SLP participants rated their satisfaction with RECOGNISE, and its usability highly. The issues identified by the SLP participants will inform the first upgrade of RECOGNISE.
Performance expectancy (i.e., usefulness) of the app was rated highly by SLP participants in this study. However, participants indicated that using the app may not be time efficient or increase their productivity. Perception of ease of use also varied across participants. RECOGNISE used an Android operating system, which may have been unfamiliar to the SLP participants and contributed to the user interface challenges identified in the qualitative content analysis. Further, in our modern digital landscape, user experience expectations are high even for complex apps (37). Users expect apps to be intuitive, effortless, and efficient at every point in their interaction with the app (37). Digital literacy levels and confidence vary among health professionals (38). Determining the digital literacy level of users and providing targeted training and education may result in improved perceptions of efficiency and ease of use (38).
SLP participants identified several user interface issues in their evaluation of RECOGNISE. Specifically, participants reported challenges with navigating the app, interpreting the results summary, loudness of some test items, scoring inaccuracies, and image quality and layout. User interface issues like those identified in our study have been reported previously (14,39). Choi and colleagues (14) experienced difficulties with automatic scoring while Guo and colleagues (39) found challenges with the absence of automatic scoring and collated patient data. In our study, only one SLP participant reported a tablet issue related to positioning of the device between the SLP and participant. Other studies reported tablet issues such as touch screen sensitivity (14), screen damage, and battery life (15). None of these issues were present in our study. The user interface issues reported by SLP participants can feasibly be incorporated into the next version of RECOGNISE.
Qualitative analysis of the open-ended question responses revealed that participants felt RECOGNISE included test items likely to detect possible communication and cognition impairments post-RH stroke. RECOGNISE includes test items for pragmatics, prosody, and narrative discourse, which are not present in existing paper-based screening tools (40,41), and yet are essential for the identification of CCD. Interestingly, SLP participants reported using locally developed, informal assessments most commonly. Until recently, research into CCD after RH stroke was limited in comparison to other acquired communication impairments such as aphasia. The two paper-based assessments were developed more than 20 years ago; understandably, they are no longer contemporary nor evidence-based. This study is an essential step towards developing an evidence-based screening tool to identify CCD after RH stroke.
SLP participants rated the app features positively in the present study. Using a tablet-based app to identify the presence or absence of CCD after RH stroke has significant advantages over using a paper-based screening tool. Some aspects of CCD such as impaired lexical semantics may be relatively straightforward to identify using a paper-based assessment. However, it is far more challenging for SLPs to screen for impairments in pragmatics and prosody using paper-based assessments. Impaired pragmatics may present as inappropriate use and/or impaired comprehension of verbal and non-verbal communication related to a specific context (5). Embedding short videos depicting real-life conversations allows for more accurate detection of impaired pragmatics. Similarly, embedding audio files of real people expressing a specific emotion (e.g., sadness) means administration is standardised with no reliance on the ‘acting’ abilities of individual SLPs. Overall, RECOGNISE incorporates audio- and video-recorded test items, written instructions, in-built recording of responses, and automatic scoring to enhance the accuracy and quality of information collected.
All participants with RH stroke found RECOGNISE engaging. Positive patient and clinician reactions of using a tablet-based screening tool or assessment to identify communication and cognitive impairments post-stroke have previously been reported (14,37,42). Engagement is important in rehabilitation: an engaged patient will ensure a holistic assessment and readiness to participate in collaborative goal setting and intervention (43). Horton and colleagues (44) reported that the type and use of meaningful real-life activities may promote patient engagement. RECOGNISE is interactive and uses test items based on real-life situations (e.g., interpreting an emotion following a surprise phone call). Clinicians’ attitudes and behaviours may also affect patient engagement in rehabilitation (45), therefore, both patient and clinician satisfaction are important to achieve.
Limitations and recommendations for future research
Successfully embedding new technology into routine clinical practice requires evaluation with the intended users (20). In this study, participants with RH stroke and SLPs evaluated RECOGNISE as the intended users. We acknowledge that our sample size was small and limited to users within a single site; however, beta testing with even small numbers of intended users may reveal a new technology’s strengths and weaknesses (46). SLP participants rated their experience using RECOGNISE after only a brief familiarisation period (i.e., after completing RECOGNISE with at least three other patients with RH stroke or when they felt confident using RECOGNISE). The app was also only available via an Android operating system, which may have contributed the user interface challenges reported, particularly those related to app navigation. It is possible that SLP participants would have rated the useability of RECOGNISE higher if an iOS operating system was available and/or if more time was available to become familiar with the app.
Future research should focus on development and testing of RECOGNISE using an iOS operating system and an improved user interface. As stroke is a global health concern (47), there is scope to trial this app in different countries and languages. We developed RECOGNISE based on expert consultation and current evidence around RH CCD presentations, however, we also need to establish reliability and validity evidence for the screening tool app.
Conclusions
We developed a screening tool app, RECOGNISE, to identify CCD after RH stroke. Our study found that RECOGNISE was rated positively by most SLP participants and all participants with RH stroke. This new screening tool administered via an app offers SLPs working in acute stroke settings an evidence-based option for identifying CCD, and an alternative to an informal paper-based assessment. With stroke one of the world’s leading causes of disability (47), early identification of communication and cognition impairments is imperative to ensure early and accurate diagnoses, comprehensive communication assessment, advocacy for access to ongoing rehabilitation, and early communication-based intervention (13). Evaluation of user acceptance of the app has allowed us to understand user experience and will inform the next iteration of the app.
Acknowledgments
We thank the speech-language pathologists and patients at Logan Hospital, Queensland, Australia for their time and effort participating in this research. We also thank the student volunteers from the Master of Speech Pathology program at Griffith University for their time in contributing to audio- and video-recorded test items.
Footnote
Data Sharing Statement: Available at https://mhealth.amegroups.com/article/view/10.21037/mhealth-24-54/dss
Peer Review File: Available at https://mhealth.amegroups.com/article/view/10.21037/mhealth-24-54/prf
Funding: This research was supported by
Conflicts of Interest: All authors have completed the ICMJE uniform disclosure form (available at https://mhealth.amegroups.com/article/view/10.21037/mhealth-24-54/coif). A.L., P.C. and R.H. report receiving The Prince Charles Hospital Foundation Innovation and Capacity Building Fund (No. IACB2016-04) and The Prince Charles Hospital Equipment Fund (No. EQ2023-15) which supported the development of the RECOGNISE App that is described in the manuscript. S.B. reports receiving The Prince Charles Hospital Foundation Innovation and Capacity Building Fund (No. IACB2016-04) which supported the development of the RECOGNISE App that is described in the manuscript. The authors have no other conflicts of interest to declare.
Ethical Statement: The authors are accountable for all aspects of the work in ensuring that questions related to the accuracy or integrity of any part of the work are appropriately investigated and resolved. The study was conducted in accordance with the Declaration of Helsinki (as revised in 2013). Ethical approval was received from Metro South Human Research Ethics Committee (No. HREC/2022/QMS/85007). All participants provided written informed consent prior to inclusion in the study.
Open Access Statement: This is an Open Access article distributed in accordance with the Creative Commons Attribution-NonCommercial-NoDerivs 4.0 International License (CC BY-NC-ND 4.0), which permits the non-commercial replication and distribution of the article with the strict proviso that no changes or edits are made and the original work is properly cited (including links to both the formal publication through the relevant DOI and the license). See: https://creativecommons.org/licenses/by-nc-nd/4.0/.
References
- Benton E, Bryan K. Right cerebral hemisphere damage: incidence of language problems. Int J Rehabil Res 1996;19:47-54. [Crossref] [PubMed]
- Blake ML, Duffy JR, Meyers PS, et al. Prevalence and patterns of right hemisphere cognitive/communicative deficits: retrospective data from an inpatient rehabilitation unit. Aphasiology 2002;16:537-47. [Crossref]
- Coté H, Payer M, Giroux F, et al. Towards a description of clinical communication impairment profiles following right-hemisphere damage. Aphasiology 2007;21:739-49. [Crossref]
- Ferré P, Fonseca RP, Ska B, et al. Communicative clusters after a right-hemisphere stroke: are there universal clinical profiles? Folia Phoniatr Logop 2012;64:199-207. [Crossref] [PubMed]
- Minga J, Sheppard SM, Johnson M, et al. Apragmatism: The renewal of a label for communication disorders associated with right hemisphere brain damage. Int J Lang Commun Disord 2023;58:651-66. [Crossref] [PubMed]
- Blake ML. The right hemisphere and disorders of cognition and communication: Theory and clinical practice. San Diego, CA: Plural Publishing; 2018.
- Dara C, Bang J, Gottesman RF, et al. Right hemisphere dysfunction is better predicted by emotional prosody impairments as compared to neglect. J Neurol Transl Neurosci 2014;2:1037. [PubMed]
- Sheppard SM, Meier EL, Zezinka Durfee A, et al. Characterizing subtypes and neural correlates of receptive aprosodia in acute right hemisphere stroke. Cortex 2021;141:36-54. [Crossref] [PubMed]
- Sheppard SM, Sebastian R. Diagnosing and managing post-stroke aphasia. Expert Rev Neurother 2021;21:221-34. [Crossref] [PubMed]
- Wall KJ, Isaacs ML, Copland DA, et al. Assessing cognition after stroke. Who misses out? A systematic review. Int J Stroke 2015;10:665-71. [Crossref] [PubMed]
- Vogel AP, Maruff P, Morgan AT. Evaluation of communication assessment practices during the acute stages post stroke. J Eval Clin Pract 2010;16:1183-8. [Crossref] [PubMed]
- Ramsey A, Blake ML. Speech-Language Pathology Practices for Adults With Right Hemisphere Stroke: What Are We Missing? Am J Speech Lang Pathol 2020;29:741-59. [Crossref] [PubMed]
- Hewetson R, Cornwell P, Shum D. Cognitive-communication disorder following right hemisphere stroke: exploring rehabilitation access and outcomes. Top Stroke Rehabil 2017;24:330-6. [Crossref] [PubMed]
- Choi YH, Park HK, Ahn KH, et al. A Telescreening Tool to Detect Aphasia in Patients with Stroke. Telemed J E Health 2015;21:729-34. [Crossref] [PubMed]
- Webb SS, Hobden G, Roberts R, et al. Validation of the UK English Oxford cognitive screen-plus in sub-acute and chronic stroke survivors. Eur Stroke J 2022;7:476-86. [Crossref] [PubMed]
- Webb SS, Jespersen A, Chiu EG, et al. The Oxford digital multiple errands test (OxMET): Validation of a simplified computer tablet based multiple errands test. Neuropsychol Rehabil 2022;32:1007-32. [Crossref] [PubMed]
- Savoldelli A, Vitali A, Remuzzi A, et al. Improving the user experience of televisits and telemonitoring for heart failure patients in less than 6 months: a methodological approach. Int J Med Inform 2022;161:104717. [Crossref] [PubMed]
- Nielsen J. Usability engineering. Boston (MA): Academic Press; 1993.
- Ahamed I, Khan S. Lambda Test. [cited 2024 Jul 28]. What is beta testing: Definitions, process, and examples. Available online: https://www.lambdatest.com/learning-hub/beta-testing
- Maramba I, Chatterjee A, Newman C. Methods of usability testing in the development of eHealth applications: A scoping review. Int J Med Inform 2019;126:95-104. [Crossref] [PubMed]
- Deniz-Garcia A, Fabelo H, Rodriguez-Almeida AJ, et al. Quality, Usability, and Effectiveness of mHealth Apps and the Role of Artificial Intelligence: Current Scenario and Challenges. J Med Internet Res 2023;25:e44030. [Crossref] [PubMed]
- Venkatesh V, Morris MG, Davis GB, et al. User Acceptance of Information Technology: Toward a Unified View. MIS Quarterly: Management Information Systems 2003;27:425-78.
- Cranen K, Drossaert CH, Brinkman ES, et al. An exploration of chronic pain patients’ perceptions of home telerehabilitation services. Health Expect 2012;15:339-50. [Crossref] [PubMed]
- Zhang Y, Liu C, Luo S, et al. Factors Influencing Patients’ Intentions to Use Diabetes Management Apps Based on an Extended Unified Theory of Acceptance and Use of Technology Model: Web-Based Survey. J Med Internet Res 2019;21:e15023. [Crossref] [PubMed]
- Alabdullah JH, Van Lunen BL, Claiborne DM, et al. Application of the unified theory of acceptance and use of technology model to predict dental students’ behavioral intention to use teledentistry. J Dent Educ 2020;84:1262-9. [Crossref] [PubMed]
- Liu L, Miguel Cruz A, Rios Rincon A, et al. What factors determine therapists’ acceptance of new technologies for rehabilitation – a study using the Unified Theory of Acceptance and Use of Technology (UTAUT). Disabil Rehabil 2015;37:447-55. [Crossref] [PubMed]
- Nielsen J. Nielsen Norman Group. 2000 [cited 2024 Sep 24]. Why you only need to test with 5 users. Available online: https://www.nngroup.com/articles/why-you-only-need-to-test-with-5-users/
- Bosworth KT, Flowers L, Proffitt R, et al. Mixed-methods study of development and design needs for CommitFit, an adolescent mHealth App. Mhealth 2023;9:22. [Crossref] [PubMed]
- De Leo G, Romski M, King M, et al. A mHealth application for the training of caregivers of children with developmental disorders in South Africa: rationale and initial piloting. Mhealth 2024;10:15. [Crossref] [PubMed]
- Jones LM, Monroe KE, Tripathi P, et al. Empowering WHISE women: usability testing of a mobile application to enhance blood pressure control. Mhealth 2024;10:26. [Crossref] [PubMed]
- Information Privacy Act 2009 (QLD) s 26. Available online: https://www.legislation.qld.gov.au/view/html/inforce/current/act-2009-014#
- Love A, Cornwell P, Hewetson R, et al. Test item priorities for a screening tool to identify cognitive-communication disorder after right hemisphere stroke. Aphasiology 2022;36:669-86. [Crossref]
- Harris PA, Taylor R, Minor BLThe REDCap consortium, et al. Building an international community of software platform partners. J Biomed Inform 2019;95:103208. [Crossref] [PubMed]
- Harris PA, Taylor R, Thielke R, et al. Research electronic data capture (REDCap)--a metadata-driven methodology and workflow process for providing translational research informatics support. J Biomed Inform 2009;42:377-81. [Crossref] [PubMed]
- Miles MB, Huberman AM, Saldaña J. Qualitative data analysis: a methods sourcebook. (Third ed.). Thousand Oaks, CA: SAGE Publications, Inc; 2014.
- Syder D, Body R, Parker M, et al. Sheffield Screening Test for Acquired Language Disorders. Windsor, UK: NFER-Nelson; 1993.
- Schrepp M, Thomaschewski J, Hinderks A. Design and evaluation of a short version of the User Experience Questionnaire (UEQ-S). International Journal of Interactive Multimedia and Artificial Intelligence 2017;4:103-8. [Crossref]
- Kuek A, Hakkennes S. Healthcare staff digital literacy levels and their attitudes towards information systems. Health Informatics J 2020;26:592-612. [Crossref] [PubMed]
- Guo YE, Togher L, Power E, et al. Assessment of Aphasia Across the International Classification of Functioning, Disability and Health Using an iPad-Based Application. Telemed J E Health 2017;23:313-26. [Crossref] [PubMed]
- Burns MS. Burns Brief Inventory of Communication and Cognition. San Antonio, TX: Psychological Corp.; 1997.
- Pimental PA, Knight JA. Mini Inventory of Right Brain Injury-Second Edition. Austin, TX: Pro-Ed Inc.; 2000.
- Wall KJ, Cumming TB, Koenig ST, et al. Using technology to overcome the language barrier: the Cognitive Assessment for Aphasia App. Disabil Rehabil 2018;40:1333-44. [Crossref] [PubMed]
- Medley AR, Powell T. Motivational Interviewing to promote self-awareness and engagement in rehabilitation following acquired brain injury: A conceptual review. Neuropsychol Rehabil 2010;20:481-508. [Crossref] [PubMed]
- Horton S, Howell A, Humby K, et al. Engagement and learning: an exploratory study of situated practice in multi-disciplinary stroke rehabilitation. Disabil Rehabil 2011;33:270-9. [Crossref] [PubMed]
- Bright FAS, Kayes NM, McPherson KM, et al. Engaging people experiencing communication disability in stroke rehabilitation: a qualitative study. Int J Lang Commun Disord 2018;53:981-94. [Crossref] [PubMed]
- TED Conferences. Designers -- think big! [video on the Internet]. Brown T. 2009, July. Available online: https://www.ted.com/talks/tim_brown_designers_think_big?language=en
- Global, regional, and national burden of stroke and its risk factors, 1990-2019: a systematic analysis for the Global Burden of Disease Study 2019. Lancet Neurol 2021;20:795-820. [Crossref] [PubMed]
Cite this article as: Love A, Cornwell P, Hewetson R, Binnewies S. A mHealth application to identify cognitive communication disorder after right hemisphere stroke: development and beta testing. mHealth 2025;11:2.