Performance of a medical smartband with photoplethysmography technology and artificial intelligence algorithm to detect atrial fibrillation
Highlight box
Key findings
• Performance of artificial intelligence (AI) algorithms: the study evaluated three AI algorithms [Happitech, Preventicus, and Philips Biostensing atrial fibrillation (AF)] for detecting AF using photoplethysmography (PPG) data from a medical smartband. Sensitivity ranged from 80% to 98%, while specificity varied from 91% to 97%.
What is known and what is new?
• AF is a common arrhythmia with serious health implications, often requiring effective monitoring and detection methods. Traditional electrocardiograms (ECGs) are the gold standard but are limited to clinical settings.
• This study demonstrates that wearable technology using PPG combined with AI can reliably detect AF in both clinical and real-world settings, presenting an alternative to traditional ECG monitoring.
What is the implication, and what should change now?
• The findings indicate that wearable devices can enhance remote monitoring and early intervention for patients at risk of AF, potentially reducing complications and healthcare costs.
• There is a need for further research on the performance of PPG technology across diverse populations and in varying conditions to refine and optimize these algorithms for broader clinical applications.
Introduction
Atrial fibrillation (AF) is the most common sustained arrhythmia and one of the most important public health issues and causes of health expenditure in Western countries (1). Patients with AF have a 5-fold higher risk of stroke, a 2-fold higher risk of death, while AF also negatively impacts quality of life (1,2). Early detection is critical for minimizing complications. The electrocardiogram (ECG) is considered the golden standard for arrhythmia detection, but its application is limited to the clinical setting, making concurrent recording with an episode of symptoms challenging (3). Additionally, AF is often asymptomatic and potentially remains undetected until complications, such as thromboembolic events, occur (4).
The use of wearable devices for recording vital parameters through sensors enables mobile diagnostics by supplying data on the cardiovascular status of a patient at home (5-8). These solutions allow for efficient and easy screening, and may reduce complications of cardiovascular disease through early detection of anomalies and appropriate therapeutic intervention (7). Advances in photoplethysmography (PPG) have produced a class of wearable devices for continuous and long-term health data acquisition and monitoring (8-10). PPG does so by emitting light, usually from a green light-emitting diode (LED), onto the skin of the wrist. This light penetrates the skin and is partially absorbed by blood vessels. When the heart beats, the blood volume in these vessels increases, leading to more light being absorbed and less being reflected back. During diastole, when the heart relaxes, there is less blood, resulting in more light being reflected. A photodetector measures this reflected light, and the variations correspond to the heartbeat, allowing for real-time heart rate monitoring (9). Importantly, PPG does not capture heart rhythm as it lacks the ability to measure the electrical activity of the heart, which is essential for detailed rhythm analysis (11). Figure 1 displays an example of concurrent ECG and PPG recording.
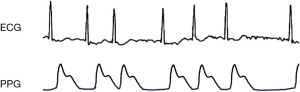
Recent studies have explored the benefits of PPG wearables in the context of post-stroke secondary prevention (12), cardiac arrest detection (13) and blood pressure monitoring (14). In the context of AF, the use of artificial intelligence (AI) to detect AF from PPG recordings is emerging, moving away from physiological thresholds to data-driven classification models such as support vector machines and deep learning (15).
These wearable devices with PPG-technology have shown to be highly accurate in heartbeat detection compared to ECG-recordings, both in healthy individuals, and in patients at risk for cardiac arrhythmias (7). However, accuracy diminishes in a real-life setting, due to movement artefacts, among other things. Additionally, patient demographics such as hair density and skin pigmentation often remain unreported, while they do have an effect on signal quality (7,16). Therefore, to further develop the PPG-technology and make it suitable for usage in clinical practice, research should focus on the impact of movement and activity on the accuracy of the PPG-signal, and on the application of algorithms to analyse heart rate variability and detect arrhythmias such as AF.
In this study, the primary objective was to compare the performance of three algorithms for detecting AF based on PPG signals acquired at the wrist, both in patients with sinus rhythm, and in patients with AF. Therefore, we aimed to compare the duration of PPG signal classified as sufficient quality to analyse the rhythm. The second aim was to compare the accuracy of each of the three algorithms for AF detection against the gold standard ECG.
Methods
Study design and patient selection
In this prospective study, we assessed the performance of the PPG sensor technology of Corsano CardioWatch 287 (Corsano Watch 287 or Corsano Medical Smartband 287, Corsano Health B.V. Bussum, The Netherlands), using three AI algorithms for AF detection. Two different populations were used in this study.
In the first phase of the study, we assessed the sensitivity and specificity of the algorithms for detecting AF in the resting condition (phase 1). In the second phase of the study, we assessed the algorithms’ sensitivity and specificity for detecting AF in free-living conditions (phase 2). Participants in phase 1 were patients with confirmed AF who were admitted to the Amsterdam University Medical Center for restoring sinus rhythm by elective electrical cardioversion (ECV). Participants in phase 2 were patients who were referred by their general practitioners to one of the outpatient clinics of Cardiology Centers, The Netherlands, for a 24-hour Holter-ECG recording. For both study phases, patients aged <18 years or those with pacemaker-dependent rhythm were excluded. The participants were consulted about the study by their treating physician, informed by a dedicated research nurse, and provided written consent for participation.
PPG-data was gathered with the Corsano CardioWatch and for each recording the presence of AF was assessed by three algorithms: Happitech (Happitech B.V., Rotterdam, The Netherlands), Preventicus (Preventicus GmbH, Jena, Germany), and Philips Biosensing AF (Koninklijke Philips N.V, Eindhoven, The Netherlands). All three algorithms use the PPG waveform, which can reveal heart rhythm by emitting light onto the skin and detecting changes in light absorption caused by blood volume fluctuations during heartbeats. The Happitech algorithm uses a shallow neural network for heartbeat detection and classifies AF by using a support vector machine model based on several rhythm and signal features, such as heart rate variability parameters and peak amplitude (17). The Preventicus algorithm detects beats by a combination of detecting the steepest slope of each pulse wave and finding the highest cross-correlation between the pulse wave and a template pulse wave (5). It classifies AF by using a complex non-linear combination analysis comprising normalised root mean square of successive difference of inter-beat intervals, two-dimensional standard deviation of the Poincare plot of successive inter-beat intervals, and Shannon entropy of inter-beat intervals (18). The Philips Biosensing AF algorithm identifies the temporal location of fiducial points in the pulse waveform to detect separate heartbeats. It uses a first-order 11-state Markov model to calculate the probability of AF given irregular patterns in the inter-beat time series (19).
The ethical approval for this study was waived by the medical ethics committee of the University of Amsterdam Medical Center. The study was conducted in accordance with the Declaration of Helsinki (as revised in 2013). All participants provided written consent upon study entry.
Data acquisition
In phase 1, PPG recordings were made before and after ECV. The participants were at rest and supine on an exam table with their arms resting on a steady surface. They were instructed not to move or speak during the measurements to ensure optimal quality of the recordings. The recordings typically lasted between 1 and 5 minutes. Cardiac rhythm was simultaneously determined using a GE ECG recorder (Munich, Germany). The participants were recruited between February and May 2021.
In phase 2, PPG recordings were made over 24 h in free-living conditions at the patients’ homes. Cardiac rhythm was simultaneously determined using a Fysiologic Holter recorder (Amsterdam, The Netherlands). A research nurse applied both the wrist band and Holter. No behavioural restrictions were imposed on the patients, except those required for the Holter, including swimming, bathing, and showering. Patients returned both devices after 24 h for the research nurse to complete the recordings. Participants were recruited from March to May 2021.
The PPG-wearables were wirelessly connected to an Android smartphone running a dedicated software. The data was transmitted from the smartband to the smartphone via Bluetooth low-energy (BLE) connection. The raw PPG data from the smartphone were transferred to a cloud server and forwarded to the cloud-based application for analysis.
Cardiovascular history, body height and weight and skin type and hair density were registered for all participants. Skin type was determined according to the Fitzpatrick classification, ranging from skin type I (pale white skin) to type VI (dark brown or black skin) (20).
Data processing and analysis
The presence of AF was labelled minute by minute in all recordings. A trained ECG technician labelled a minute of ECG recording as AF if the physiological features of AF were present for at least 30 seconds. The three investigational algorithms computed probability of AF for a minute of PPG recording based on the extraction of statistical features, and used proprietary thresholds to classify the minute as AF.
Each algorithm had at least an ‘AF’ label, but the algorithms differed in the remaining options such as sinus rhythm, non-sinus rhythm and unclassified rhythm. We manually overrode these labels with a ‘non-AF’ label, as we wanted to assess accuracy specifically for AF detection. We compared the AF- and non-AF classification with the gold standard, which is the outcome of the ECG recording as classified by a trained ECG technician. We only included the minutes of PPG recordings that were deemed of sufficient quality for analysis by each algorithm.
Statistical analysis
In phase 1, we assessed sensitivity and specificity by comparing the amount of recordings labeled as AF by the three algorithms to those labeled by the ECG technician. We considered one recording to be AF if it contained at least one minute labeled as AF. We calculated sensitivity and specificity by (21).
We calculated the confidence intervals (CI) of sensitivity and specificity by
In phase 2, we assessed sensitivity and specificity for each recording by comparing the amount of minutes labelled as AF by the three algorithms to those labelled by the ECG technician. This yielded one sensitivity and one specificity for each recording. We calculated the average sensitivity and specificity with their confidence intervals across all recordings weighted by the amount of minutes per recording.
See Figure 2 for a detailed overview of the study phases.
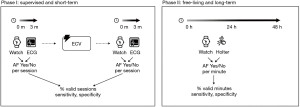
Results
Participant characteristics
In total, we studied data from 72 participants, of which 34 (21% female, 65±11 years old) were in phase 1 and 38 (61% female, 50±17 years old) in phase 2. The participants in phase 1 were patients with confirmed AF who were scheduled for elective cardioversion. Patients in phase 2 were suspected to have cardiovascular disease according to complaints, medical history, and the presence of cardiovascular risk factors and were scheduled for a diagnostic Holter ECG examination. Skin type heterogeneity was limited, as 78% of the participants were classified as cream white skin (Fitzpatrick type III). Hair density varied among the participants, with a predominance of moderate hair density. The baseline characteristics of all participants are presented in Table 1.
Table 1
Characteristic | Total (N=72) | Phase 1 (N=34) | Phase 2 (N=38) |
---|---|---|---|
Female | 30 [42] | 7 [21] | 23 [61] |
Age (years) | 57±17 | 65±11 | 50±17 |
BMI (kg/m2) | 27±5 | 28±5 | 26±4 |
Hypertension | 17 [24] | 11 [32] | 6 [16] |
Diabetes mellitus | 4 [6] | 2 [6] | 2 [5] |
Dyslipidemia | 16 [22] | 14 [41] | 2 [5] |
History of cardiac arrhythmia | – | – | 20 [53] |
History of ischaemic heart disease | – | – | 8 [21] |
Skin type | |||
I | 5 [7] | 0 [0] | 5 [13] |
II | 5 [7] | 4 [12] | 1 [3] |
III | 56 [78] | 28 [82] | 28 [74] |
IV | 6 [8] | 2 [6] | 4 [11] |
Hair density | |||
Nil | 8 [11] | 5 [15] | 3 [8] |
Sparse | 18 [25] | 9 [26] | 9 [24] |
Moderate | 34 [47] | 10 [29] | 24 [63] |
Dense | 12 [17] | 10 [29] | 2 [5] |
Data are presented as n [%] or mean ± SD. Phase 1: detection of AF in resting condition; Phase 2: detection of AF in free-living condition. BMI, body mass index; SD, standard deviation.
PPG signal quality
The first study phase resulted in 68 recordings from 34 participants before and after ECV. The Preventicus and Happitech algorithms classified more than three out of four measurements as usable, whereas the Philips Biosensing AF classified 63% as usable. Phase 2 resulted in 862 h of simultaneous ECG and PPG recording. Both the Preventicus and the Happitech algorithms classified less than half of the recorded minutes as sufficient quality, while this percentage was higher for Philips Biosensing AF. Table 2 provides an overview of the quality qualifications of the different algorithms in the data from the two populations.
Table 2
Phase | Preventicus | Happitech | Philips |
---|---|---|---|
1 (% of recordings) | 78% | 76% | 63% |
2 (% of minutes) | 46% | 48% | 70% |
PPG, photoplethysmography.
Detection of AF
In phase 1, all recordings that were made before ECV [34] showed AF, while the other half of the recordings made after ECV showed SR. Phase 2 resulted in a total of 64.967 recorded ECG minutes, of which 3.192 were classified as AF by a Holter technician.
In phase 1, the specificity for the detection of AF was high, varying in the algorithms between 90.6% (95% CI: 80.5–100%) and 96.9% (95% CI: 90.8–100%). The sensitivity was lower, with a range of 80.0% (95% CI: 62.5–97.5%) and 86.7% (95% CI: 69.5–100%). In phase 2, the three algorithms showed high sensitivity and specificity for the detection of AF, with sensitivity values varying between 96.5% (95% CI: 96.4–96.7%) and 97.6% (95% CI: 97.6–97.6%) and specificity values varying between 94.8% (95% CI: 94.6–95.0%) and 96.0% (95% CI: 95.8–96.1%). Table 3 provides an overview of the outcomes of the three algorithms.
Table 3
Phase | Preventicus | Happitech | Philips | |||||
---|---|---|---|---|---|---|---|---|
Sensitivity (95% CI) | Specificity (95% CI) | Sensitivity (95% CI) | Specificity (95% CI) | Sensitivity (95% CI) | Specificity (95% CI) | |||
1 | 81.0% (64.2–97.8%) | 96.9% (90.8–100%) | 80.0% (62.5–97.5%) | 90.6% (80.5–100%) | 86.7% (69.5–100%) | 96.4% (89.6–100%) | ||
2 | 96.5% (96.4–96.7%) | 95.9% (95.9–96.0%) | 97.1% (95.7–98.5%) | 96.0% (95.8–96.1%) | 97.6% (97.6–97.6%) | 94.8% (94.6–95.0%) |
CI, confidence interval.
Discussion
We found that the algorithms show high sensitivity and specificity for AF-detection compared to a standard 12-lead ECG. We observed this both in a clinical setting and in an unsupervised ambulant environment. The part of the recordings that is discarded because of insufficient quality varies among the algorithms but is generally high. Movement of the wrist on which the smartbands with the PPG-sensors are worn causes artefacts, making considerable parts of the signal unusable for algorithm classification, even in patients lying on the examination table for undergoing ECV.
The fact that PPG-based applications are hampered by motion artefacts has long been recognized, but figures on this phenomenon to evaluate this potential drawback are scarce (22-24). However, recent studies typically show insufficient quality for around one third to half of the recorded data, similar to our results (10,25,26). For two of the algorithms that we tested, we found that only half of the data could be used to detect AF. The implications of this finding depend on the application. Given its robustness and accuracy, ECG remains the first choice for rapid hospital screening. However, in the ambulatory setting, the non-invasive nature of wearable PPG technology enables longer duration of monitoring, significantly adding to the chance to detect rhythm abnormalities (27). A discard rate of 50% of PPG data may then be considered acceptable, as long as the accuracy is high enough to analyse the remaining data to avoid false positives and false negatives, which our study demonstrates.
We divided our study into two phases and used different methodologies. In phase 1, we classified each recording as AF or non-AF, and in phase 2, we classified each recording minute-by-minute. We chose this approach considering the differences in patient populations. Patients in phase 1 had a confirmed diagnosis of persistent AF, full recording would be expected to show the AF rhythm. Patients in phase 2 were suspected of having paroxysmal AF; therefore, the recording would be expected to contain both AF and non-AF rhythms.
The investigational algorithms have been studied before in controlled settings, yielding sensitivity and specificity values of 91.5% and 99.6% for Preventicus (28), 98.1% and 98.1% for Happitech (17), and 97% and 99% for Philips Biosensing AF (19), respectively. We found similar sensitivity and specificity values in phase 2 of our study, but phase 1 yielded different results, contrasting our expectations. There may be multiple explanations for this finding. First, the number of observations was considerably lower in phase 1. Therefore, incorrect classifications may have had a greater impact on the overall sensitivity and specificity measures compared to phase 2. Second, and most likely, the measurement time in phase 1 was too short to reach optimal conditions for both the sensors and the algorithms. Sensors have a ‘warm-up’ time and normally algorithms need time to find the best thresholds for beat detection, which differ among individuals. Third, the signal qualifiers of the algorithms are likely to be most sensitive to motion artifacts. Therefore, the algorithms may have been more aggressive in rejecting signals in the free-living environment of phase 2, resulting in higher sensitivity and specificity compared to phase 1.The minute-by-minute approach in phase 2 means that only a few seconds of low signal quality can render the entire minute unusable for analysis. Although a second-by-second analysis would result in less loss of data due to low signal quality and possibly higher sensitivity and specificity, this approach was not applicable in our methodology as it would not have been feasible for the ECG technician to label the recordings second-by-second comparing the three algorithms. We first analyzed the parts of the recordings that were classified as high quality by each of the algorithms. This classification had an impact on the following analysis, in which we compared the accuracy, defined by sensitivity and specificity. Although Preventicus and Happitech had similar classifications of signal quality, this was different for the Philips Biosensing AF algorithm. In phase 1, Philips classified a lower number of recordings as high quality compared to the other two algorithms, while in phase 2, the reverse was true. As expected, this resulted in a relatively high sensitivity and specificity for Philips Biosensing AF in phase 1. In phase 2, this difference had a limited impact on the results, as the number of observations was considerably higher.
The selection of the algorithms for this study was based on their different modes of action. The Preventicus algorithm is cloud-based, while the Happitech algorithm runs on a companion smartphone, and the Philips algorithm is designed to run on the wearable itself. The Preventicus cloud-based algorithm enables the long-term storage of the underlying PPG and accelerometry data for future development. A disadvantage is the heavy data transmission load and associated costs. The companion-app-based Happitech algorithm offers the same accuracy without the cost of the required transmission of raw data to the cloud. Finally, the Philips algorithm is located in a wearable device and does not require a companion app or cloud-based processing. While the accuracy is slightly lower, this solution is particularly useful for in-hospital monitoring, where the wearable syncs data directly to a router and the hospital information technology (IT) system.
Our study has some limitations. First, wearables with PPG sensors typically use accelerometer data to determine movement and track external disturbances. These data help the algorithm correctly classify PPG data as AF or non-AF. In our study, CardioWatch generated a one-dimensional accelerometer signal, whereas three-dimensional data positively influenced the sensitivity and specificity. Second, our study aimed to compare the accuracy of the three individual algorithms for detecting AF in a clinical setting. We used ECG as the ground truth to achieve this, but did not aim to set PPG off against ECG in the first place. As such, we chose not to include the recorded minutes labelled as bad quality when calculating the sensitivity and specificity. This provides an optimistic estimation of sensitivity and specificity, as these metrics would most likely drop when artifactual PPG data are included. However, this is specifically relevant when viewing PPG as a potential replacement for ECG, while PPG is mostly regarded as a companion to ECG; e.g., to accessibly screen before deploying a Holter or to extend monitoring time when a Holter could not capture AF. It should be noted that little is known about the proportion of analyzable minutes from a Holter under free-living conditions. We suggest that future studies perform a detailed comparison of the analyzable minutes between ECG and PPG. Third, we were unable to explore whether patient demographics contributed to the sensitivity and specificity values, as participant heterogeneity was relatively limited. For example, the skin type has a direct effect on the quality of the signal, as more pigments yield less reflection of the light emitted by LEDs. However, 78% of the cohort belonged to one skin-type class, leaving too few patients in other classes for comparison. Future studies should investigate participant diversity through a design. Finally, we selected participants who had already been referred to a cardiologist with a high a priori risk of AF. We foresee that AF detection using wearables will be deployed on a larger scale as a screening method in the future, for example, in the general practitioner population. We suggest that future studies explore the accuracy and benefits of PPG wearables in this context.
Based on our comprehensive study comparing the three algorithms utilizing PPG technology for AF detection, we conclude that wearable devices equipped with PPG sensors offer promising sensitivity and specificity for AF detection. Our findings suggest that the Preventicus and Happitech algorithms demonstrate superior performance, with high accuracy rates. Therefore, we recommend the use of the Preventicus and Happitech algorithms for patients with persistent AF and those suspected of having paroxysmal AF. This approach can significantly enhance the efficiency and accuracy of arrhythmia detection using PPG technology, ultimately improving patient outcomes and the quality of care.
Conclusions
This study demonstrated the potential of medical smartbands with PPG technology combined with AI algorithms for reliable AF detection. These findings suggest a promising direction for remote AF monitoring and early intervention, potentially reducing AF-related complications and healthcare costs.
Acknowledgments
None.
Footnote
Data Sharing Statement: Available at https://mhealth.amegroups.com/article/view/10.21037/mhealth-24-10/dss
Peer Review File: Available at https://mhealth.amegroups.com/article/view/10.21037/mhealth-24-10/prf
Funding: This research was supported by a grant from
Conflicts of Interest: All authors have completed the ICMJE uniform disclosure form (available at https://mhealth.amegroups.com/article/view/10.21037/mhealth-24-10/coif). All authors report the grant from Corsano Health B.V. The authors have no other conflicts of interest to declare.
Ethical Statement: The authors are accountable for all aspects of the work in ensuring that questions related to the accuracy or integrity of any part of the work are appropriately investigated and resolved. The study was conducted in accordance with the Declaration of Helsinki (as revised in 2013). The ethical approval for the study design and procedures was waived by the ethics committee of the University of Amsterdam Medical Center. All participants provided written consent upon study entry.
Open Access Statement: This is an Open Access article distributed in accordance with the Creative Commons Attribution-NonCommercial-NoDerivs 4.0 International License (CC BY-NC-ND 4.0), which permits the non-commercial replication and distribution of the article with the strict proviso that no changes or edits are made and the original work is properly cited (including links to both the formal publication through the relevant DOI and the license). See: https://creativecommons.org/licenses/by-nc-nd/4.0/.
References
- Zoni-Berisso M, Lercari F, Carazza T, et al. Epidemiology of atrial fibrillation: European perspective. Clin Epidemiol 2014;6:213-20. [Crossref] [PubMed]
- Wolf PA, Abbott RD, Kannel WB. Atrial fibrillation as an independent risk factor for stroke: the Framingham Study. Stroke 1991;22:983-8. [Crossref] [PubMed]
- Stracina T, Ronzhina M, Redina R, et al. Golden Standard or Obsolete Method? Review of ECG Applications in Clinical and Experimental Context. Front Physiol 2022;13:867033. [Crossref] [PubMed]
- Hochstadt A, Chorin E, Viskin S, et al. Continuous heart rate monitoring for automatic detection of atrial fibrillation with novel bio-sensing technology. J Electrocardiol 2019;52:23-7. [Crossref] [PubMed]
- Koenig N, Seeck A, Eckstein J, et al. Validation of a New Heart Rate Measurement Algorithm for Fingertip Recording of Video Signals with Smartphones. Telemed J E Health 2016;22:631-6. [Crossref] [PubMed]
- Gielen W, Gielen M. Two causes of palpitations, detected by photoplethysmography on a mobile phone. Neth Heart J 2019;27:56-7. [Crossref] [PubMed]
- Blok S, Piek MA, Tulevski II, et al. The accuracy of heartbeat detection using photoplethysmography technology in cardiac patients. J Electrocardiol 2021;67:148-57. [Crossref] [PubMed]
- Dur O, Rhoades C, Ng MS, et al. Design Rationale and Performance Evaluation of the Wavelet Health Wristband: Benchtop Validation of a Wrist-Worn Physiological Signal Recorder. JMIR Mhealth Uhealth 2018;6:e11040. [Crossref] [PubMed]
- Allen J. Photoplethysmography and its application in clinical physiological measurement. Physiol Meas 2007;28:R1-39. [Crossref] [PubMed]
- Selder JL, Te Kolste HJ, Twisk J, et al. Accuracy of a Standalone Atrial Fibrillation Detection Algorithm Added to a Popular Wristband and Smartwatch: Prospective Diagnostic Accuracy Study. J Med Internet Res 2023;25:e44642. [Crossref] [PubMed]
- Yuda E, Shibata M, Ogata Y, et al. Pulse rate variability: a new biomarker, not a surrogate for heart rate variability. J Physiol Anthropol 2020;39:21. [Crossref] [PubMed]
- Motolese F, Capone F, Magliozzi A, et al. A smart devices based secondary prevention program for cerebrovascular disease patients. Front Neurol 2023;14:1176744. [Crossref] [PubMed]
- Edgar R, Scholte NTB, Ebrahimkheil K, et al. Automated cardiac arrest detection using a photoplethysmography wristband: algorithm development and validation in patients with induced circulatory arrest in the DETECT-1 study. Lancet Digit Health 2024;6:e201-10. [Crossref] [PubMed]
- van Vliet M, Monnink SHJ, Kuiper MJ, et al. Evaluation of a novel cuffless photoplethysmography-based wristband for measuring blood pressure according to the regulatory standards. Eur Heart J Digit Health 2024;5:335-43. [Crossref] [PubMed]
- Harmon DM, Sehrawat O, Maanja M, et al. Artificial Intelligence for the Detection and Treatment of Atrial Fibrillation. Arrhythm Electrophysiol Rev 2023;12:e12. [Crossref] [PubMed]
- Koerber D, Khan S, Shamsheri T, et al. Accuracy of Heart Rate Measurement with Wrist-Worn Wearable Devices in Various Skin Tones: a Systematic Review. J Racial Ethn Health Disparities 2023;10:2676-84. [Crossref] [PubMed]
- Mol D, Riezebos RK, Marquering HA, et al. Performance of an automated photoplethysmography-based artificial intelligence algorithm to detect atrial fibrillation. Cardiovasc Digit Health J 2020;1:107-10. [Crossref] [PubMed]
- Krivoshei L, Weber S, Burkard T, et al. Smart detection of atrial fibrillation. Europace 2017;19:753-7. [PubMed]
- Bonomi AG, Schipper F, Eerikäinen LM, et al. Atrial fibrillation detection using photo-plethysmography and acceleration data at the wrist. In: 2016 Computing in Cardiology Conference (CinC). IEEE; 2016:277-80.
- Fitzpatrick TB. The validity and practicality of sun-reactive skin types I through VI. Arch Dermatol 1988;124:869-71. [Crossref] [PubMed]
- Fleiss JL. Statistical Methods for Rates and Proportions. Second Edition. New York: Wiley, John and Sons, Incorporated; 1981.
- Yu C, Liu Z, McKenna T, et al. A method for automatic identification of reliable heart rates calculated from ECG and PPG waveforms. J Am Med Inform Assoc 2006;13:309-20. [Crossref] [PubMed]
- Nemati S, Ghassemi MM, Ambai V, et al. Monitoring and detecting atrial fibrillation using wearable technology. Annu Int Conf IEEE Eng Med Biol Soc 2016;2016:3394-7. [Crossref] [PubMed]
- Yang C, Veiga C, Rodríguez-Andina JJ, et al. Using PPG Signals and Wearable Devices for Atrial Fibrillation Screening. IEEE Trans Ind Electron 2019;66:8832-42. [Crossref]
- Selder JL, Proesmans T, Breukel L, et al. Assessment of a standalone photoplethysmography (PPG) algorithm for detection of atrial fibrillation on wristband-derived data. Comput Methods Programs Biomed 2020;197:105753. [Crossref] [PubMed]
- Mohagheghian F, Han D, Ghetia O, et al. Atrial fibrillation detection on reconstructed photoplethysmography signals collected from a smartwatch using a denoising autoencoder. Expert Syst Appl 2024;237:121611. [Crossref]
- Diederichsen SZ, Haugan KJ, Kronborg C, et al. Comprehensive Evaluation of Rhythm Monitoring Strategies in Screening for Atrial Fibrillation: Insights From Patients at Risk Monitored Long Term With an Implantable Loop Recorder. Circulation 2020;141:1510-22. [Crossref] [PubMed]
- Brasier N, Raichle CJ, Dörr M, et al. Detection of atrial fibrillation with a smartphone camera: first prospective, international, two-centre, clinical validation study (DETECT AF PRO). Europace 2019;21:41-7. [Crossref] [PubMed]
Cite this article as: Blok S, Gielen W, Piek MA, Hoeksema WF, Tulevski I, Somsen GA, Winter MM. Performance of a medical smartband with photoplethysmography technology and artificial intelligence algorithm to detect atrial fibrillation. mHealth 2025;11:5.