Monitoring health as an opportunity to categorize preventative and early-treatment actions in a self-care journey: our experience with a Healthcare Magenta Scorecard
Highlight box
Key findings
• We demonstrate the results of the development and implementation of a health scorecard (Magenta Score), which is a broad and multidimensional mobile-based tool that measures health and wellbeing in a health insurance environment.
What is known and what is new?
• There are some promising emergent initiatives on the topic, but few reports regarding usefulness, specially in the decision-making process.
• We describe the rationale that underpins the development and implementation of this novel tool and present early findings.
What is the implication, and what should change now?
• Further studies are needed to explore how healthcare decisions could be supported by such prospective health and wellbeing data collection.
Introduction
The rapid increase of internet users over the last few decades was followed by an incremental integration of health information technology (HIT) into the healthcare system globally. These technologies facilitate the collection and incorporation of patient-reported information into clinical practice. Electronic Patient Reported Outcomes (e-PRO) evaluation systems allow for continuous standardized patient assessments and have resulted in a lower response burden and better user experience compared to paper-based PRO evaluations (1-3). Additionally, e-PRO systems contain features that enable progress tracking by both clinicians and patients providing real-time assessment and feedback (2).
Most studies and implementations of e-PRO systems have focused on specific diseases or conditions such as oncology. These systems have the potential to improve the identification and monitoring of essential aspects of care, such as treatment-related adverse effects (4-7). These tools are growing in use and applicability and we believe that applying them to primary care and prevention could have myriad potential benefits (2,3,8). Thus, we developed a combination of e-PROs that measure a broad scope of health dimensions associated with an increased risk of chronic diseases: dietary habits, physical activity, sleep, and mental health.
This research piece is derived from the efforts of a primary-care driven, digital-first healthcare system that seeks to implement more preventative-focused actions, supported by digital-native patients invited to join self-care journeys. These patients are supported by a nursing team that are guided by evidence-based medicine protocols. Our recent research has shown that 64% of acute conditions were resolved with the assistance of our telehealth platform, mainly driven by nurses (1). Thus, acute conditions are already partly being addressed by our digital ecosystem. However, preventative measures are still lacking a clearer and data-driven approach.
We aimed to develop a Healthcare Magenta Scorecard, mainly from the aggregation e-PROs, as a guide to understand which healthcare actions were lacking in our population and also as a method for patients to partake in their own selfcare. This study aims to describe the rationale of our healthcare scoreboard (Magenta Score) and some preliminary data from our initial efforts. As a secondary objective we investigated some psychometric properties among the domains that encompasses our Magenta Scorecard.
Methods
This is a single-center preliminary pilot study regarding the development of a health scorecard in our private healthcare system (Alice Healthcare, São Paulo, SP, Brazil). The study was conducted in accordance with the Declaration of Helsinki (as revised in 2013).
The rationale behind the development of our Magenta Scorecard
We believe that continuous improvement in 6 domains of health and wellbeing are crucial to health promotion and prevention. We realize that there is great heterogeneity in defining which are the most relevant domains that best translate into realising health and well being improvements. In our thesis, we chose test the following (9-21): physical activity, sleep quality, nutrition, habits/lifestyle, mental health, quality of life (QoL).
Our decision to scrutinize these domains is the fact that previous research has proven benefits in early-action from these conditions. Our score ranks our patients’ health status from 0 to 1,000, categorizing into 3 levels our health status and wellbeing: poor [<500]; good [500–750]; and excellent [>750].
These categories serve as a guide to stratify which patients are in need of a more detailed follow-up and care approach by our care coordination team, and also to foster engagement and ongoing compliance in using a mobile-based app.
Data capturing and guided approach
We administered the score card every 3–5 months and patients supplied responses to questionnaires by means of a mobile app. As most of the information about the health plan (accredited network, available medical network, pending health actions) is within the mobile application, the scorecard was a pending action when joining the health plan and a new assessment was encouraged every 3–5 months. We included a convenience sample, which is those that responded to the scorecard from December 2021 to November 2022. We included only patients that responded at the scorecard completely two times in a more than 3-month timespan. Incomplete scorecard responders were excluded. There were no extra incentives regarding to responding the scorecard besides measuring patient health and wellbeing status. Data was captured inside our healthcare standard alone native IOS and/or Android mobile-app. For data collected we used a sliding scale or descriptive alternatives according to scorecard phases. Data was sent to the investigator by online update applied to central database. Data was captured prospectively into a mobile app by participants themselves and data extracted retrospectively from our database for this manuscript needs.
Score categorization from our Magenta Scorecard divided patients into 4 categories, which guided our approach and the resources available, as follows:
- Case management: in-person primary-care physician and nursing strategies, focused on personal frequent consultations and health specialist intensive support. Example: obese and depressive patient with previous suicidal attempts.
- Disease management: primary care nursing and physician intensive care, may require health specialist support (preferable from mobile/telehealth sources). Example: patients with uncontrolled diabetes, with disease-related complications.
- Self-care support: primary care nursing and physician care, does not require health specialist support. Example: patient with controlled type II diabetes and no organ-target disease.
- Health and wellbeing promotion: primary care nursing a physician care based on health-care journeys. Patients may join into mobile-based health prevention and promotion journeys if they choose to do so. Example: middle-age patients with no health conditions, willing to improve their food ingestion quality.
Characteristics of our domain selection
Physical activity
Engaging in physical activity reduces the risk of death up to 40% (9). In our Magenta Scorecard we incorporated the short version of International Physical Activity Questionnaire (IPAQ) (10,11) in which metabolic equivalent of tasks (METs) were translated into our Magenta Score as follows:
- More than 3,000 METs/week: excellent Magenta Scorecard: >750).
- Action: digital compliments for exercising maintenance and provision of digital group classes.
- More than 1,680–3,000 METs/week: good (Magenta Scorecard: 500–750).
- Action: suggest an online approach focused on physical education and provision of digital group classes.
- <600 METs/week: poor (Magenta Scorecard: <500).
- Action: strongly suggest online consultation with physical education professionals.
Sleep quality
Several robust systematic reviews support the association of poorer sleep quality and increased risk of mortality (12-14). For our scoreboard, we adapted the mini sleep questionnaire (MSQ) (15), which focuses on sleep quality and wake complaints (insomnia and excessive daytime sleepiness). Each item is scored on a seven-point Likert scale ranging from 1 (never) to 7 (always), and is based on symptoms occurring over the past 7 days. Scores are categorized as: 10–24, good sleep quality; 25–27, mild sleep difficulties; 28–30, moderate sleep difficulties; ≥31, severe sleep difficulties. The MSQ questionnaire has been validated as a good method for screening sleep disorders and also as a tool for measuring sleep-related QoL (16). The questionnaire is short and easy to apply. Health actions triggered by MSQ responses occur as follow:
- Good/mild sleep difficulties (Magenta Scorecard: >750).
- Action: maintenance of good habits, e-leaflets for good sleep maintenance.
- Moderate sleep difficulties (Magenta Scorecard: 500–750).
- Action: synchronous online advising with a nurse and/or psychologist; offer talking circles.
- Severe sleep difficulties (Magenta Scorecard: <500).
- Action: synchronous online consultation (with a primary care physician and/or sleep specialist; offer talking circles.
Nutrition
Research has proven that improved food intake is associated with a decrease in mortality (17). We opted to measure the quality of food intake by adapting recommendations from Brazil’s Ministry of Health recommendations (18). In this part of the scoreboard, we consider both healthy and unhealthy food intake in order to build a score that represents the overall patient nutritional pattern.
- Patients with excellent pattern of food intake (Magenta Scorecard: >750).
- Action: maintenance of good habits; offer self-care journeys.
- Patients with good patterns of food intake (Magenta Scorecard: >500–750).
- Action: main offer self-care e-journeys, maintenance of good habits, offer talking circles.
- Patients with poor patterns of food intake (Magenta Scorecard <500).
- Action: improvement of habits related to nutrition; offer talking circles; offer self-care journeys.
- Patients who spontaneously desires to improve the quality of food intake (own initiative).
- Action: offer self-care e-journeys.
Habits/lifestyle
Alcohol and smoking are modifiable risk factors and ceasing the use of such substances has been proven to reduce morbidity and mortality (19-21). In our Magenta Scorecard, we considered the number of cigarettes/week? and weekly doses of alcohol to determine longitudinal tracking in primary care.
- More than 5 cigarettes per week or more than 14 doses of alcohol per week: poor (Magenta Scorecard <500).
- Action: strongly suggest an online nursing consultation to assess motivation to change habits, health literacy and referral to substance abuse cessation programs.
- Less than 5 cigarettes per week and less than 14 doses of alcohol per week: good and excellent (Magenta Scorecard >500).
- Action: digital compliments for healthy lifestyle habits and digital materials for health promotion.
Mental health
Anxiety and depression screening compose the Mental Health domain of Magenta Scorecard. It is administered using the reduced versions of the Generalized Anxiety Disorder (GAD) scale for anxiety symptoms (22,23) and the Patient Health Questionnaire (PHQ) (24) for depression symptoms. In the case of scores greater than 2 points in any of the scales, the extended version of the scale with altered score is applied (22), in order to deeply investigate mental health conditions.
- Patients with negative screening for anxiety and depression (GAD-2 and PHQ-2 scores <3): excellent (Magenta Scorecard: >750).
- Action: asynchronous psychoeducational materials that aim to empower patients’ habits, behaviors and health promotion.
- Patients with GAD-7 or PHQ-9 ≥10: good (Magenta Scorecard: 500–750).
- Action: in-person online consultation with a primary care physician.
- Patients with GAD-7 or PHQ-9 <10: good (Magenta Scorecard: 500–750).
- Action: e-leaflets for mental health promotion, talking circles referral, offer self-care e-journeys.
- Patients with GAD-7 or PHQ-9 ≥10: poor (Magenta Scorecard <500).
- Action: in-person online consultation (primary care physician).
- Patients with GAD-7 or PHQ-9 <10: poor (Magenta Scorecard <500).
- Action: e-leaflets for mental health promotion, offer self-care e-journeys.
QoL
We measured QoL as a subjective indicator to determine how globally QoL is perceived from their perspective (25,26). We opted to employ the EuroQol short version (5Q-5D-5L), which contains questions regarding mobility, self-care, usual activities, pain/discomfort, and anxiety/depression (25,27).
Magenta Scorecard
Magenta Scorecard is the summary of the score (mean score) of all the 6 domains described above.
Statistical analysis
We used descriptive statistics including means and 95% confidence intervals (CIs) to describe sample characteristics. The distribution of the data was checked by the Shapiro-Wilk test and also visual distribution. For continuous data, if considered as normal, Student’s paired t-tests were applied, otherwise a non-parametric alternative was used, such as Wilcoxon (2 sets of data) or Kruskal-Wallis (>2 sets of data). After Kruskal-Wallis test, pairwise analysis was performed in order to identify differences with Dwass-Steel-Critchlow-Fligner pairwise comparisons. For those patients with more than one measure of Magenta Score throughout the year were created a sample group to evaluate the evolution through time. For this sample, Wilcoxon rank test was performed to compare the initial Magenta Score value with the final Magenta Score value.
A correlation matrix was constructed using the Spearman correlation test in order to summarize the strength and direction of a relationship between domains. In order to categorize the strength of the correlation, Cohen criteria were used: (I) ≤0.39 weak correlation; (II) 0.4–0.69 moderate correlation; (III) 0.7–0.89 strong correlation; (IV) ≥0.9 very strong correlation (28). For all analysis, we considered an alpha less than 0.05 as significant.
Results
Sample characteristics
Data was collected cross-sectionally from December 2021 to November 2022 and included 5,757 participants. Of 5,757 potential participants considered for enrollment in this study, 4,095 participants were excluded after applying inclusion and exclusion criteria. 1,662 participants completed follow-up at both T0 and T1 milestones and were included in the final data analysis.
The mean age of our sample was 31.3 years (95% CI: 31.2–31.5), ranging from 18 to 89 years old. Most of the participants were female (63.4%). From our sample, 0.8% were categorized as case management, 8.4% disease management, 35.3% self-care care support and 55.5% health and wellbeing promotion.
Magenta Scorecard measures: the impact of our healthcare actions
From our sample, 0.8% were categorized as case management, 8.6% disease management, 35.6% self-care care support and 55% health and wellbeing promotion, respectively.
From 5,757 participants, 1,622 responded at T0 and T1 and were included in the final analyses. The mean interval between measurements was 141 ± standard deviation (SD) days. At baseline, 25% were categorized as excellent, 61% as good and 14% as poor. After the study period, 34% were categorized as excellent; 53% as good and 13% as poor. Table 1 and Figure 1 depicts our baseline scores categorized by our Magenta Scorecard domains.
Table 1
Variables | Baseline (T0) (n=1,622) | T1 (n=1,622) | |||
---|---|---|---|---|---|
Mean [SD] | 95% CI | Mean [SD] | 95% CI | ||
Sleep quality* | 585 [198] | 575–595 | 626 [213] | 615–636 | |
Quality of life* | 716 [144] | 709–723 | 731 [152] | 724–738 | |
Physical activity* | 457[340] | 440–473 | 467 [346] | 450–484 | |
Nutrition* | 638 [163] | 630–646 | 671 [158] | 664–679 | |
Mental health** | 688 [243] | 676–700 | 697 [260] | 684–709 | |
Habit/lifestyle* | 849 [160] | 841–857 | 861 [150] | 854–868 | |
Magenta Score* | 649 [132] | 643–656 | 675 [143] | 668–682 |
Wilcoxon W test. *, P<0.001; **, P=0.085. CI, confidence interval; SD, standard deviation.
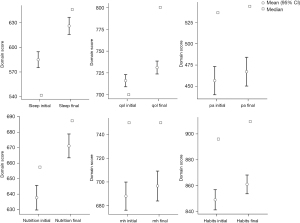
Only patients that responded to both baseline T0 and T1 (n=1,622) questionnaires were analyzed. The Magenta Scorecard scores improved from 649±132 to 675±143, paired samples t-test, P<0.001. Our analysis demonstrated that the only parameter that did not display a statistically significant difference was Mental Health (P=0.085 >0.05).
Magenta Score and our guided approach
The results of Table 2 and Figure 2 demonstrate that scores derived from Magenta Score were significantly different. From the Dwass-Steel-Critchlow-Fligner pairwise comparisons we can conclude that there is a difference for all domains examined except between case management and disease management, which are the more resource demanding scenarios. Our Magenta Scorecard domains had significant, however, weak spearman correlations (Table 3) in pairwise comparisons.
Table 2
Mean [SD], baseline, n=575 | Case management (n=38) | Disease management (n=408) | Self-care support (n=1,718) | Health & wellbeing (n=2,702) | |
---|---|---|---|---|---|
Case management (n=38) | 523 [152] | N/A | 0.691** | 0.002** | <0.001** |
Disease management (n=408) | 547 [132] | N/A | <0.001** | <0.001** | |
Self-care support (n=1,718) | 615 [137] | N/A | <0.001** | ||
Health & wellbeing (n=2,702) | 698 [121] | N/A | |||
P value* | 0.001* |
*, Kruskal-Wallis; **, Dwass-Steel-Critchlow-Fligner pairwise comparisons. SD, standard deviation; N/A, not applicable.
Table 3
Quality of life | Nutrition | Habits | Physical activity | Mental health | Sleep quality | ||||||||||||
---|---|---|---|---|---|---|---|---|---|---|---|---|---|---|---|---|---|
Spearman’s rho | P value | Spearman’s rho | P value | Spearman’s rho | P value | Spearman’s rho | P value | Spearman’s rho | P value | Spearman’s rho | P value | ||||||
Quality of life | – | – | |||||||||||||||
Nutrition | 0.338 | <0.001*** | – | – | |||||||||||||
Habits | 0.193 | <0.001*** | 0.209 | <0.001*** | – | – | |||||||||||
Physical activity | 0.333 | <0.001*** | 0.375 | <0.001*** | 0.094 | <0.001*** | – | – | |||||||||
Mental health | 0.443 | <0.001*** | 0.241 | <0.001*** | 0.113 | <0.001*** | 0.251 | <0.001*** | – | – | |||||||
Sleep quality | 0.416 | <0.001*** | 0.273 | <0.001*** | 0.155 | <0.001*** | 0.249 | <0.001*** | 0.531 | <0.001*** | – | – |
***, P<0.001.
Discussion
Our results demonstrate that our Magenta Scorecard (Magenta Score) may be a useful tool to categorize and prioritize healthcare delivery and action. It may be a useful adjunct to triage and individualize the care plan for patients entering the healthcare system. We can allocate resources for effectively and efficiently with respect to preventative measures and case/disease management. A patient’s Magenta Score may potentially be used as a valuable “healthcare compass” aiding the predictability, and allocation of costs in a more targeted manner. Future cost-effectiveness studies are needed to explore this area.
Our Magenta Scorecard was empirically developed based on medical and non-medical resources to measure parameters of well-being and disease. Since the beginning of the “outcomes measurement movement” most of the stakeholders focused on measurement of disease-related outcomes (29). It is becoming clearer that measuring non-medical parameters, such as habits/lifestyle may be as important as measuring disease itself. One recent study (30) has proposed a “whole person score” which is in line with our Magenta Score approach. These authors considered: physical Health, emotional Health, resource utilization, socioeconomics, ownership, and nutrition and lifestyle as pillars to measure non-medical parameters and they focus on non-medical actions in order to promote health and well-being. Their approach focuses on efforts such as social status and ownership. Their further results will be important as a guide to the improvement of our Magenta Score (30).
One limitation of our study concerns patient engagement rates. As we use mobile-based e-PROs, we need to address some challenges, especially by using gamification strategies for serial measurements over time. e-PRO completion may empower and prompt patients to engage in the care process (31-33), the rates of continual responses are below expected. Patient engagement rates are below ideal and may be skewed by factors, such as patient health status and the type of survey. Electronic-based questionnaires may have a response rate as low as 46%. Comprehensive and long e-PROs, such as Magenta Score may have even lower response rates (34). As such, we still need to conduct a prospective validation study to verify whether our scorecard has acceptable psychometric properties.
The only domain that did not significantly improve over time was mental health. As it covers mostly anxiety and depressive conditions, it is likely that our timespan was not long enough to elicit changes. We hypothesize that maybe a 6-month measurement would be a more accurate timeframe for mental health conditions. In addition, it is now clear that the young population is suffering chronically from mental disorders, with the previous coronavirus disease (COVID) pandemic as a major contributor (35,36). In line with these assumptions, correlations were weaker for QoL × habits/lifestyle and habits/lifestyle × mental health which may be a result of the challenge for re-adapting day-to-day life patterns in the post-covid era (35,36).
Conclusions
We present the rational for our Magenta Scorecard and its guided approach. The Magenta Scorecard demonstrated responsiveness across the two serial measurements investigated and was signicantly correlated with all the construct domains examined. Further research is needed to validate our results in the long term and additional strategies for patient engagement should be explored.
Acknowledgments
We acknowledge Stephanie Witzel, Priscilla Fassini, Lilian Moreno, Bruno Cerazi for their contribution with the scorecard development.
Funding: None.
Footnote
Data Sharing Statement: Available at https://mhealth.amegroups.com/article/view/10.21037/mhealth-22-56/dss
Peer Review File: Available at https://mhealth.amegroups.com/article/view/10.21037/mhealth-22-56/prf
Conflicts of Interest: All authors have completed the ICMJE uniform disclosure form (available at https://mhealth.amegroups.com/article/view/10.21037/mhealth-22-56/coif). All authors report that they are Alice employees with close relations with the company. The authors have no other conflicts of interest to declare.
Ethical Statement: The authors are accountable for all aspects of the work in ensuring that questions related to the accuracy or integrity of any part of the work are appropriately investigated and resolved. The study explored and exposed our Magenta Scorecard framework and used anonymous data collected previously for operational/healthcare purposes. There was no analysis from health individual personal data. As anonymous preliminary data, it fits as waived from ethical approval (including informed consent) (Resolution 674, Capítulo IX, Art. 26, 2022). The study was conducted in accordance with the Declaration of Helsinki (as revised in 2013).
Open Access Statement: This is an Open Access article distributed in accordance with the Creative Commons Attribution-NonCommercial-NoDerivs 4.0 International License (CC BY-NC-ND 4.0), which permits the non-commercial replication and distribution of the article with the strict proviso that no changes or edits are made and the original work is properly cited (including links to both the formal publication through the relevant DOI and the license). See: https://creativecommons.org/licenses/by-nc-nd/4.0/.
References
- de Moraes VY, Ferreira CB, Kawagoe CK, et al. An integrative telehealth platform managed by nurses. BMC Res Notes 2022;15:301. [Crossref] [PubMed]
- Hans PK, Gray CS, Gill A, et al. The provider perspective: investigating the effect of the Electronic Patient-Reported Outcome (ePRO) mobile application and portal on primary care provider workflow. Prim Health Care Res Dev 2018;19:151-64. [Crossref] [PubMed]
- Steele Gray C, Gill A, Khan AI, et al. The Electronic Patient Reported Outcome Tool: Testing Usability and Feasibility of a Mobile App and Portal to Support Care for Patients With Complex Chronic Disease and Disability in Primary Care Settings. JMIR Mhealth Uhealth 2016;4:e58. [Crossref] [PubMed]
- Hayashida T, Nagayama A, Seki T, et al. Feasibility study on collecting patient-reported outcomes from breast cancer patients using the LINE-ePRO system. Cancer Sci 2022;113:1722-30. [Crossref] [PubMed]
- Wright A. Evaluation of two mobile health apps for patients with breast cancer using the Mobile Application Rating Scale. mHealth 2021;7:60. [Crossref] [PubMed]
- Ravn S, Thaysen HV, Verwaal VJ, et al. Cancer follow-up supported by patient-reported outcomes in patients undergoing intended curative complex surgery for advanced cancer. J Patient Rep Outcomes 2021;5:120. [Crossref] [PubMed]
- Denis F, Krakowski I. How Should Oncologists Choose an Electronic Patient-Reported Outcome System for Remote Monitoring of Patients With Cancer? J Med Internet Res 2021;23:e30549. [Crossref] [PubMed]
- Singh H, Tahsin F, Nie JX, et al. Exploring the perspectives of primary care providers on use of the electronic Patient Reported Outcomes tool to support goal-oriented care: a qualitative study. BMC Med Inform Decis Mak 2021;21:366. [Crossref] [PubMed]
- Andrade LH, Wang YP, Andreoni S, et al. Mental disorders in megacities: findings from the São Paulo megacity mental health survey, Brazil. PLoS One 2012;7:e31879. [Crossref] [PubMed]
- Hallal PC, Gomez LF, Parra DC, et al. Lessons learned after 10 years of IPAQ use in Brazil and Colombia. J Phys Act Health 2010;7:S259-64. [Crossref] [PubMed]
- Matsudo S, Araújo T, Matsudo V, et al. Questionário InternacionaL De Atividade Física (IPAQ): Estudo De Validade E Reprodutibilidade No Brasil. Rev Bras Atividade Física Saúde 2001;5-18.
- Cappuccio FP, D'Elia L, Strazzullo P, et al. Sleep duration and all-cause mortality: a systematic review and meta-analysis of prospective studies. Sleep 2010;33:585-92. [Crossref] [PubMed]
- de Morais LC, Zanuto EAC, Queiroz DC, et al. Association between sleep disorders and chronic diseases in patients of the Brazilian national health system. J Phys Educ 2017;28:e2844.
- Yin J, Jin X, Shan Z, et al. Relationship of Sleep Duration With All-Cause Mortality and Cardiovascular Events: A Systematic Review and Dose-Response Meta-Analysis of Prospective Cohort Studies. J Am Heart Assoc 2017;6:e005947. [Crossref] [PubMed]
- Falavigna A, de Souza Bezerra ML, Teles AR, et al. Consistency and reliability of the Brazilian Portuguese version of the Mini-Sleep Questionnaire in undergraduate students. Sleep Breath 2011;15:351-5. [Crossref] [PubMed]
- Fabbri M, Beracci A, Martoni M, et al. Measuring Subjective Sleep Quality: A Review. Int J Environ Res Public Health 2021;18:1082. [Crossref] [PubMed]
- Wang DD, Li Y, Bhupathiraju SN, et al. Fruit and Vegetable Intake and Mortality: Results From 2 Prospective Cohort Studies of US Men and Women and a Meta-Analysis of 26 Cohort Studies. Circulation 2021;143:1642-54. [Crossref] [PubMed]
- Jaime PC, da Silva ACF, Lima AMC, et al. Food and nutrition actions in primary healthcare: the experience of the Brazilian government. Rev Nutr 2011;24:809-24. [Crossref]
- Stead LF, Buitrago D, Preciado N, et al. Physician advice for smoking cessation. Cochrane Database Syst Rev 2013;2013:CD000165. [Crossref] [PubMed]
- O'Malley SS, O'Connor PG. Medications for unhealthy alcohol use: across the spectrum. Alcohol Res Health 2011;33:300-12. [PubMed]
- Stahre M, Roeber J, Kanny D, et al. Contribution of excessive alcohol consumption to deaths and years of potential life lost in the United States. Prev Chronic Dis 2014;11:E109. [Crossref] [PubMed]
- Spitzer RL, Kroenke K, Williams JBW, et al. A Brief Measure for Assessing Generalized Anxiety Disorder: The GAD-7. Arch Intern Med 2006;166:1092-7. [Crossref] [PubMed]
- Moreno AL, DeSousa DA, de Souza AMFLP, et al. Factor Structure, Reliability, and Item Parameters of the Brazilian-Portuguese Version of the GAD-7 Questionnaire. Temas Em Psicol 2016;24:367-76. [Crossref]
- Arroll B, Goodyear-Smith F, Crengle S, et al. Validation of PHQ-2 and PHQ-9 to screen for major depression in the primary care population. Ann Fam Med 2010;8:348-53. [Crossref] [PubMed]
- Rabin R, de Charro F. EQ-5D: a measure of health status from the EuroQol Group. Ann Med 2001;33:337-43. [Crossref] [PubMed]
- Santos M, Monteiro AL, Santos B. EQ-5D Brazilian population norms. Health Qual Life Outcomes 2021;19:162. [Crossref] [PubMed]
- Feng YS, Kohlmann T, Janssen MF, et al. Psychometric properties of the EQ-5D-5L: a systematic review of the literature. Qual Life Res 2021;30:647-73. [Crossref] [PubMed]
- Schober P, Boer C, Schwarte LA. Correlation Coefficients: Appropriate Use and Interpretation. Anesth Analg 2018;126:1763-8. [Crossref] [PubMed]
- de Moraes VY, Ferrari PM, Gracitelli GC, et al. Outcomes in orthopedics and traumatology: translating research into practice. Acta Ortop Bras 2014;22:330-3. [Crossref] [PubMed]
- The Whole PERSON Health Score. A Patient-Focused Tool to Measure Nonmedical Determinants of Health. NEJM Catal Innov Care Deliv 2022; [Crossref]
- Greenhalgh J, Gooding K, Gibbons E, et al. How do patient reported outcome measures (PROMs) support clinician-patient communication and patient care? A realist synthesis. J Patient Rep Outcomes 2018;2:42. [Crossref] [PubMed]
- Neale J, Strang J. Philosophical ruminations on measurement: methodological orientations of patient reported outcome measures (PROMS). J Ment Health 2015;24:123-5. [Crossref] [PubMed]
- Kettis Å, Fagerlind H, Frödin JE, et al. Quality of life assessments in clinical practice using either the EORTC-QLQ-C30 or the SEIOQL-DW: a randomized study. J Patient Rep Outcomes 2021;5:58. [Crossref] [PubMed]
- Meyer VM, Benjamens S, Moumni ME, et al. Global Overview of Response Rates in Patient and Health Care Professional Surveys in Surgery: A Systematic Review. Ann Surg 2022;275:e75-81. [Crossref] [PubMed]
- Gagné T, Nandi A, Schoon I. Time trend analysis of social inequalities in psychological distress among young adults before and during the pandemic: evidence from the UK Household Longitudinal Study COVID-19 waves. J Epidemiol Community Health 2021;76:421-7. [Crossref] [PubMed]
- Pedersen MT, Andersen TO, Clotworthy A, et al. Time trends in mental health indicators during the initial 16 months of the COVID-19 pandemic in Denmark. BMC Psychiatry 2022;22:25. [Crossref] [PubMed]
Cite this article as: de Moraes VY, Silva RP, Kawagoe CK, Távora PRP, Cassola N, Ferretti M. Monitoring health as an opportunity to categorize preventative and early-treatment actions in a self-care journey: our experience with a Healthcare Magenta Scorecard. mHealth 2023;9:25.